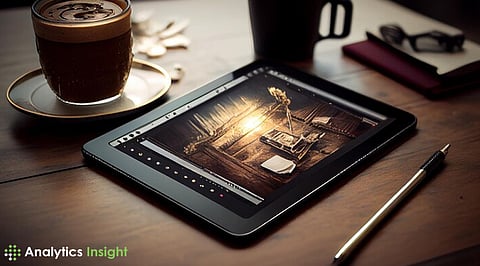
The intersection of text and image understanding is a hard nut to crack in the field of Artificial Intelligence, more specifically under generative AI. OpenAI's CLIP model fully breaks it, however. The way it changes text-to-image creation now machines not only understand but generate images from textual descriptions with even greater accuracy and flexibility than previously thought is groundbreaking. This article explains how CLIP works, its impact on generative AI, and its industry applications.
OpenAI developed CLIP, an AI model that by a stretch of the imagination can understand and relate text and images close enough to human perception. CLIP stands for Contrastive Language–Image Pre-training.
In simple terms, this is the training of a neural network so that it can understand concepts from natural language supervision. Unlike conventional models, which are based on labeled datasets, CLIP learns from a large corpus of text-image pairs available on the internet.
The dual-encoder architecture used by CLIP is composed of a text encoder and an image encoder. Here is how it works:
Collection of data: The model learns from the data, which is a wide dataset with millions of images and, most importantly, textual descriptions.
Text Encoder: The text encoder encodes the textual descriptions in high-dimensional vectors.
Image Encoder: The image encoder does a similar job but with images, turning them into high-dimensional vectors.
This is through a contrastive loss function used while training, to make the vector spaces of matched texts and images close and the ones of unmatched pairs far apart. After being pre-trained, the model can execute all tasks with no tasks or specific training inscribed in the models' generalization ability on pre-trained datasets.
This generalization entails contrasting a given piece of extracted text from an image with another image.
Before CLIP, most of the text-to-image models failed to create coherent and contextually correct images from textual descriptions. In other words, CLIP text-image representations are both robust and nuanced in their capture of semantics. This improvement, in turn, enables the image being generated to be more accurate and detailed.
CLIP's ability to understand both text and images and their relationship closes significant gaps in AI by delivering more human and intuitive experiences. This is critical for applications that need to understand the visual and textual contexts for applications like image search engines, content-creation tools, and digital assistance.
Zero-Shot Capabilities
One of the most disruptive features of CLIP comes in the form of zero-shot learning. More traditional models need task-specific fine-tuning to perform new tasks, while CLIP can generalize from its pre-training across a variety of tasks without any further training. This significantly reduces the time and resources spent on the deployment of AI models over different applications.
Artists/designers can use CLIP to generate artwork/designs from textual descriptions. For instance, an artist would be able to input a description like "a serene sunset over a mountain range" and use CLIP to generate an image that matches this description. This can be a great new way of art expression in the future, extended toward collaboration among human beings and various machines.
It can use the generation of images for articles, blogs, and social media. With just a brief description or set of keywords, input CLIP can output images relevant to the content to improve visual features. This whole automation in the generation of the content ensures that the visuals are proper and are a direct relation to the textual content.
Virtual reality and gaming would be one of the things that CLIP can do in tectonic shifts to image search engines, empowering them with more intuitive and accurate search results. Users can look up images using natural language descriptions rather than needing to rely on specific keywords.
For example, searching for "a red sports car on a rainy day" returns images that closely match this description, giving a user-friendly experience.
It can be used to create realistic settings and characters from textual descriptions into a virtual reality and a game. A developer can describe a scene, an object, or a character, and then with the use of CLIP, it can generate corresponding visuals. This capacity enhances the dynamic and engaging game experience.
Can also be a tool to make things accessible to people with visual impairment by providing descriptive images, these can help transfer information visually in a more accessible approach. The application can improve the scope of inclusive and accessible elements of digital content.
CLIP is trained on a massive dataset of images and their associated text. All are sourced from the web. This is an interesting architecture employing two modules, a Vision Transformer, in the case of the Image Encoder, and another Transformer-based one for text encoding. All these encoders map their respective inputs into the same latent space, in which similarity is measured.
The contrastive loss function is one of the most critical components of CLIP that relates texts and images. It pushes the cosine similarity of matching Text-Image pairs to the maximum, and for non-matching pairs, it pushes them to the minimum during training. This is how CLIP can learn robust and generalizable representations of visual and textual concepts.
The training it receives across heterogeneous internet data gives it broad coverage of most visual and textual concepts, hence generalizing well across new tasks. It does this generalization very explicitly in its performance on zero-shot learning tasks, where it applies pre-trained knowledge to execute new tasks without further training.
Like most AI models, CLIP is vulnerable to biases in its training data. Since the source of data is the Internet, it surely contains biased or inappropriate content. Discussing how this would be addressed is very important to have fair and ethical applications of AI.
While the training and deployment of CLIP are both highly compute-intensive, a venue for the democratization of the benefits of CLIP is still an open question.
While performance is very good, it often remains a hard problem to glean how CLIP arrives at a decision and interprets relationships between text and images. Making the outputs of CLIP more interpretable is a predicate of trust-building and responsible use.
One may direct the future study toward ways to establish techniques for identifying and purifying the biases in both the training data and outputs of CLIP. Such techniques, including adversarial training, augmentation of the training data, and bias correction algorithms, would ensure the fairness and ethics of the models developed within artificial intelligence.
Another key research direction would be to make CLIP's outputs interpretable. As much as the performance of the tool can be improved, the way CLIP understands and associates text and images might be important in eliciting insights and instilling trust in the minds of users.
Fully unlocking the potential of CLIP requires looking at new applications in areas such as health, education, and environmental sciences. For example, in the synthesis of medical images derived from textual descriptions, educational content creation, and visualization of climate data.
The realization of this multimodal potential with CLIP and integrated other AI models opens a powerful system that gives much more comprehensive and sophisticated AI capability, opening wide possibilities for innovations.
CLIP is a giant progress in generative AI, and the old way of text-to-image generation by machines has been greatly revolutionized. This works by zero-shot learning of a model for vision-language alignment, so it is versatile and thus powerful—be it for art, design, content creation, or even accessibility.
As we delve further into the powers and continue to finesse those of CLIP, it will be very critical for us to confront other challenging fronts associated with data bias, interpretability, and accessibility. This will unlock the full potential that this milestone model holds for driving innovation and creativity in AI. As such, the future of text-to-image creation is bright, and the leader in this journey is CLIP.