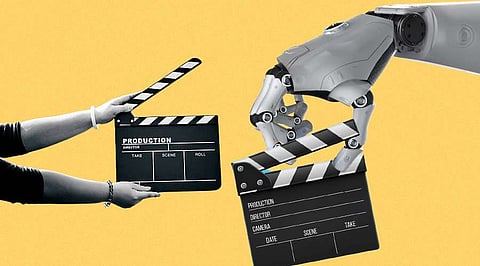
Generative AI models are artificial intelligence that can create new and realistic content based on existing data, such as images, text, music, and video. Generative models have many potential applications in various domains, such as entertainment, education, marketing, design, and healthcare. However, deploying generative models in production also poses many challenges that must be addressed. In this article, we will discuss three of these challenges and some possible solutions.
Generative models require high-quality data to learn from and generate realistic and diverse content. However, data collection and preparation can be costly, time-consuming, and prone to errors and biases. Moreover, data privacy and security issues may limit the availability and accessibility of data for generative models. Therefore, generative models must ensure that they use reliable and representative data sources, apply proper data cleaning and augmentation techniques, and comply with ethical and legal standards for data protection.
Generative models are often complex and computationally intensive to train and serve. They may require specialized hardware and software infrastructure, such as GPUs, TPUs, cloud computing, and distributed systems. Moreover, generative models are difficult to evaluate and compare, as there is no clear and objective metric to measure their quality and diversity. Therefore, generative models need to optimize their resource utilization, scalability, and efficiency and develop appropriate evaluation methods and benchmarks that reflect the desired outcomes and user feedback.
Generative models are often opaque and unpredictable in their behavior and outputs. They may generate inaccurate, inappropriate, or harmful content to users or society. They may also infringe on the original content creators' or consumers' intellectual property rights or moral values. Therefore, generative models need to ensure that they are transparent and accountable for their actions and decisions and respect the rights and preferences of the stakeholders involved. They also need to provide explanations and justifications for their outputs and mechanisms for correction and control.
These are some of the challenges that generative models face when deploying in production. However, these challenges also present opportunities for innovation and improvement. By addressing these challenges, generative models can become more robust, reliable, and responsible for creating valuable content for users and society.
Join our WhatsApp Channel to get the latest news, exclusives and videos on WhatsApp
_____________
Disclaimer: Analytics Insight does not provide financial advice or guidance. Also note that the cryptocurrencies mentioned/listed on the website could potentially be scams, i.e. designed to induce you to invest financial resources that may be lost forever and not be recoverable once investments are made. You are responsible for conducting your own research (DYOR) before making any investments. Read more here.