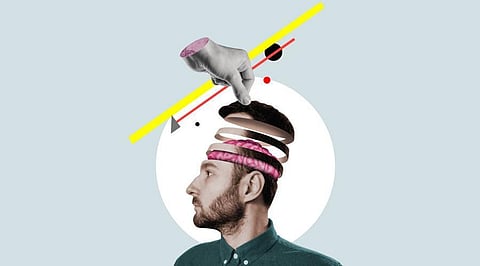
To identify, organize, or reason data, many artificial intelligence (AI) techniques are used. In order to synthesize sights, sounds, and movies that frequently appear to be getting more realistic, generative algorithms build data using models of the real world. The algorithms build a simulated environment that matches the model by starting with assumptions about how a world should be. Numerous content creation roles commonly involve generative AIs. They are sometimes employed by filmmakers to carry a significant portion of the plot or to fill in narrative holes. Some news outlets produce brief summaries of events or even complete pieces, particularly in the case of highly structured sports or financial reporting.
Some generative algorithms don't result in content. In order to improve the screen or user interfaces, some algorithms are used in user interfaces. Some people assist the blind by producing audio descriptions. The strategies often support rather than take center stage in many applications. Since algorithms are now so widespread, developers can choose their objectives creatively. Some individuals strive for the most lifelike results and evaluate them by the way they like humans or animals compared to photographs of genuine animals. Others seek to create a more stylized product that is obviously not real but rather more like a cartoon. They think like painters or animators.
Realistic pictures, sounds, and story creation is a relatively new field with a lot of current studies. There are many different and flexible techniques. Today, scientists are still developing novel structures and tactics. Generative Adversarial Networks (GAN), a popular strategy, rely on at least two separate AI algorithms battling against one another before convergent decision-making. A single algorithm, frequently a neural network, is in charge of generating a first draft of the answer. The phrase "generative network" describes it. The quality of the solution is assessed by a second algorithm, which is typically a neural network, by contrasting it with other plausible solutions. The "discriminator network" is a common term for this. There may occasionally be different iterations of the discriminator or generator.
Each side of the algorithm trains the other as the procedure is repeated numerous times. The generator picks up on which outcomes are more desirable. The discriminator gains knowledge of the results' components that are most likely to reflect realism. Transformers use a different way that avoids the adversarial method. The most practical results are generated by a single network that has been trained. Microsoft has one that has been trained over time using sizable blocks of text culled from Wikipedia and the broader internet. It is known as GPT-n for General, Pre-trained Network. The most recent version, GPT-3, is a closed source and directly licensed for several activities, including generative artificial intelligence. There are reportedly more than 175 billion factors in it. Other comparable models exist, such as Google's LaMDA (Language Model for Dialogue Applications) and Wu Dao 2.0 in China. The term "Variational Auto-Encoder" is sometimes used to describe a third type. These methods rely on compression algorithms, which reduce the size of data files by taking use of some of the internal patterns and structures. These algorithms operate backward, driving creation with random values.
Many concepts and methods used by computer games and computer graphics are frequently adapted by generative AI researchers. Nevertheless, generative AI and the realm of computer games are sometimes seen as independent entities. Algorithms that use generative AI aim to replace artists in this capacity. The AI is in charge of organizing the scenes and selecting the elements before placing them there. The model's rules may have been partially developed by a human, but the algorithm should ultimately serve as the director or creator.
Some generative AI algorithms have the capacity to fool. These outcomes, which are frequently referred to as "deep fakes," might be used to pose as someone else and engage in various types of fraud in their name. Some people might try to impersonate someone else to steal money from a bank. In an effort to accuse someone of a crime like libel or slander, other people may try to put words in another person's mouth. Making pornography appears to incorporate another person is one particularly sleazy method. These outcomes might be employed for retaliation, extortion, blackmail, or coercion.
Join our WhatsApp Channel to get the latest news, exclusives and videos on WhatsApp
_____________
Disclaimer: Analytics Insight does not provide financial advice or guidance. Also note that the cryptocurrencies mentioned/listed on the website could potentially be scams, i.e. designed to induce you to invest financial resources that may be lost forever and not be recoverable once investments are made. You are responsible for conducting your own research (DYOR) before making any investments. Read more here.