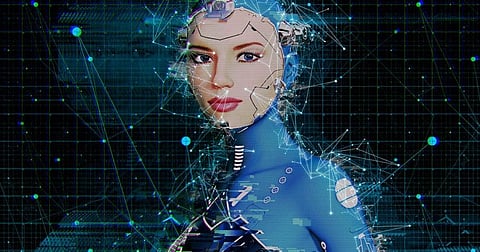
Over the last few decades, research, activity, and funding have been devoted to improving the recruitment, retention, and advancement of women in the fields of science, engineering, and medicine. In recent years the diversity of those participating in these fields, particularly the participation of women, has improved and there are significantly more women entering careers and studying science, engineering, and medicine than ever before. However, as women increasingly enter these fields they face biases and barriers and it is not surprising that sexual harassment is one of these barriers.
According to the National Academies of Sciences, Engineering, and Medicine 2018, report, the count of women in science is decreasing since 1990. The report also revealed that till 2015, women made up only 18% of computer science majors in the US — a decline from a high of 37% in 1984. A study by the National Center for Women in Information Technology discovered that around 50 percent of the women who go into technology eventually leave the field — more than double the percentage of men who leave.
Another revelation made by the AI Now Institute last year says, women currently make up 24.4% of the computer science workforce and receive median salaries that are only 66% of the salaries of their male counterparts.
Furthermore, the AI Now Institute report says, there is a diversity crisis in the AI sector across gender and race. Recent studies found only 18% of authors at leading AI conferences are women and more than 80% of AI professors are men. This disparity is extreme in the AI industry: women comprise only 15% of AI research staff at Facebook and 10% at Google. There is no public data on trans workers or other gender minorities. For black workers, the picture is even worse. For example, only 2.5% of Google's workforce is black, while Facebook and Microsoft are each at 4%.
Given decades of concern and investment to redress this imbalance, the current state of the field is alarming.
The AI sector needs a profound shift in how it addresses the current diversity crisis. The artificial intelligence industry needs to acknowledge the gravity of its diversity problem, and admit that existing methods have failed to contend with the uneven distribution of power, and the means by which AI can reinforce such inequality. Further, many researchers have shown that bias in AI systems reflects historical patterns of discrimination. These are two manifestations of the same problem, and they must be addressed together.
The overwhelming focus on 'women in tech' is too narrow and likely to privilege white women over others. We need to acknowledge how the intersections of race, gender, and other identities and attributes shape people's experiences with AI. The vast majority of artificial intelligence studies assume gender is binary, and commonly assign people as 'male' or 'female' based on physical appearance and stereotypical assumptions, erasing all other forms of gender identity
Fixing the 'pipeline' won't fix AI's diversity problems. Despite many decades of 'pipeline studies' that assess the flow of diverse job candidates from school to industry, there has been no substantial progress in diversity in the AI industry. The focus on the pipeline has not addressed deeper issues with workplace cultures, power asymmetries, harassment, exclusionary hiring practices, unfair compensation, and tokenization that are causing people to leave or avoid working in the AI sector altogether.
The use of artificial intelligence systems for the classification, detection, and prediction of race and gender is in urgent need of re-evaluation. The histories of 'race science' are a grim reminder that race and gender classification based on appearance is scientifically flawed and easily abused. Systems that use physical appearance as a proxy for character or interior states are deeply suspect, including AI tools that claim to detect sexuality from headshots, predict 'criminality' based on facial features, or assess worker competence via 'micro-expressions.' Such systems are replicating patterns of racial and gender bias in ways that can deepen and justify historical inequality. The commercial deployment of these tools is a cause for deep concern.
Remedying bias in artificial intelligence systems is almost impossible when these systems are opaque. Transparency is essential, and begins with tracking and publicizing where AI systems are used, and for what purpose. Rigorous testing should be required across the lifecycle of AI systems in sensitive domains. Pre-release trials, independent auditing, and ongoing monitoring are necessary to test for bias, discrimination, and other harms.
Moreover, the field of research on bias and fairness needs to go beyond technical de-biasing to include a wider social analysis of how AI is used in context. This necessitates including a wider range of disciplinary expertise. The methods for addressing bias and discrimination in AI need to expand to include assessments of whether certain systems should be designed at all, based on a thorough risk assessment.
Join our WhatsApp Channel to get the latest news, exclusives and videos on WhatsApp
_____________
Disclaimer: Analytics Insight does not provide financial advice or guidance. Also note that the cryptocurrencies mentioned/listed on the website could potentially be scams, i.e. designed to induce you to invest financial resources that may be lost forever and not be recoverable once investments are made. You are responsible for conducting your own research (DYOR) before making any investments. Read more here.