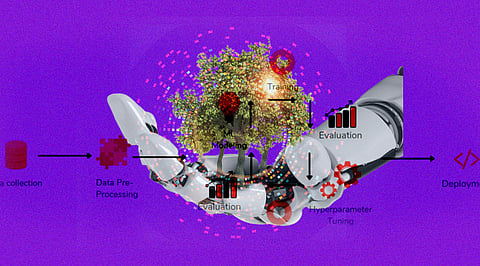
Every AI system needs data and models to function properly and generate the desired results. We all understand that machine learning is an iterative process because it is largely an empirical field. Thinking about the problem inhibits you from coming up with the best solution right away since you are unable to clearly articulate what it would involve. As a consequence, you use empirical methods to look for better solutions. When you are utilizing this iterative method, you have two primary possibilities.
To enhance performance, this entails constructing empirical tests around the model. Finding the best model architecture and training method from a vast number of options is what this entails. Since machine learning (ML) is an iterative process, developing empirical tests to evaluate the model's performance is part of it. Getting a better solution entails selecting the best model architecture and training method from a vast universe of options. Ng claims that under the prevalent model-centric approach to AI, you gather all the data you can and then create a model powerful enough to handle the noise in the data. According to the defined procedure, the data must be kept constant while the model is improved iteratively until the intended outcomes are obtained.
This entails methodically modifying or upgrading the datasets to raise the AI system's accuracy. Data collecting is frequently considered a one-time operation and this is largely neglected. This entails methodically modifying or upgrading the datasets to raise the AI system's accuracy. Data collection is frequently considered a one-time operation and this is largely neglected.
The consistency of the data is crucial in the emerging data-centric approach to AI, according to Ng. You must keep the model or code unchanged and iteratively raise the data quality to achieve the desired outcomes. This strategy is more appealing to most machine learning engineers, in part because it gives them a chance to put their understanding of machine learning models to use. Contrarily, dealing with data is sometimes viewed as a low-skill endeavor, and many engineers would rather work with models. Is this focus on models, however, justified? Why is it there?
The majority of individuals have been focusing a significant percentage of their efforts on model-centric AI. The fact that the AI business closely monitors AI university research is one possible explanation. The majority of cutting-edge advancements in the area are easily accessible to practically everyone who can utilize GitHub because of the open source ethic in AI. To ensure that AI research is still applicable to tackling issues in the real world, tech titans also fund and direct a significant percentage of it.
Recently, AI research has only ever focused on models! This is because it has become customary to create difficult and sizable datasets that serve as generally recognized standards for evaluating a problem's performance. Thereafter, scholars compete to meet these goals at the cutting edge of technology! Since the dataset's condition has already been fixed, the majority of research is focused on model-centric approaches. This gives the community the sense that a model-centric approach is more promising.
Although the machine learning community recognizes the value of data and credits voluminous data as a key factor in the development of AI. In the course of an ML project's life cycle, it occasionally gets neglected. In a recent speech, Andrew NG made clear his preference for a data-centric strategy and called for a shift in the community's attitude in that direction. He gives a few examples as an illustration of how the data-centric approach generated better results.
The volume of data is crucial; you need to have enough data to solve your problem. Deep Networks are low bias, high variance computers, and we think the solution to the variance issue is more data. However, accumulating data indiscriminately may be expensive and extremely data efficient.
Consistency in data annotation is crucial since any discrepancy might cause the model to fail and render your evaluations invalid. According to a recent study, around 3.4% of samples in frequently used datasets were incorrectly tagged. They also discovered that the bigger models are more negatively impacted by this. Many study articles surpass prior standards by a percentage point or two, but if the accuracy cannot be assessed without the mistake of +=3.4 percent, serious concerns are raised! Therefore, we require a consistently labeled dataset for improved training and trustworthy assessment.
Your data should cover all variables that deployment data will show and be an accurate representation of the data you anticipate seeing during deployment. All data qualities that aren't causal features should ideally be sufficiently randomized.
Join our WhatsApp Channel to get the latest news, exclusives and videos on WhatsApp
_____________
Disclaimer: Analytics Insight does not provide financial advice or guidance. Also note that the cryptocurrencies mentioned/listed on the website could potentially be scams, i.e. designed to induce you to invest financial resources that may be lost forever and not be recoverable once investments are made. You are responsible for conducting your own research (DYOR) before making any investments. Read more here.