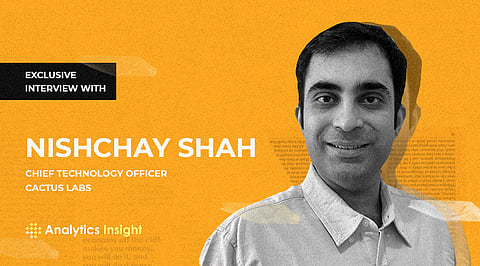
Cactus Communications is at the forefront of making scientific research accessible worldwide through language services, communication, publishing services, and AI-powered products for researchers. CACTUS Labs, the R&D division, operates at the forefront of AI, NLP, and big data technology. It is a globally distributed company with a remote-first policy. Lately, the company has been creating solutions that are becoming one of the finest, most rapid interpreters and enhancers of scientific information and literature. Analytics Insight has engaged in an exclusive interview with Nishchay Shah, Chief Technology Officer, CACTUS.
Cactus Communications is at the forefront of making scientific research accessible worldwide through language services, communication, publishing services, and AI-powered products for researchers. We work with researchers, scientists, Nobel laureates, academic societies, life science companies, scholarly publishers, government bodies and think tanks from every part of the world. We build products powered by some of the most sophisticated AI-based language technology that exists.
CACTUS Labs, the R&D division, operates at the forefront of AI, NLP and bigdata technology. We are a globally distributed company with a remote-first policy. Lately, we have been creating solutions that are becoming one of the finest, most rapid interpreters and enhancers of scientific information and literature. We combine design, tech, and the best language experts to tackle real-world science problems. The products we create are helping great science come to the forefront in more significant ways than ever before.
I oversee technology and innovation across products and brands globally at CACTUS. I am experienced in handling tech-budgeting, outsourcing, and global tech recruitment, and manage a large department with over 300 experts working in product management, software development, UX, DevOps, Digital innovation, and Machine Learning. I focus on creating, translating, and mobilizing big-picture visions downstream. I have over 17 years of experience in software development and technology and strive to stay on the bleeding edge of innovation. I've worked in the US for over a decade and handled a diverse team in the US, Belarus, Bulgaria, India, and the UK. Having successfully led both B2C and B2B product teams in the past, I have a thorough understanding of the end-to-end product and technology lifecycles.
Academic Language Editing
Helps editors and researchers by taking care of grammar error detection + correction and providing sentence formation suggestions and potential reference checks from a corpus of millions of research artifacts
Academic Data Intelligence
We have deduplicated and cleaned nearly 300M+ academic articles and their metadata (Eg – journals, papers, clinical reports, etc) and created a large data lake
One of the most successful outcomes out of this data lake is R Discovery, our flagship product, which provides research paper recommendations for reading based on user profiles and macro + micro subject areas
Concept Extraction
The Concept Extraction engine facilitates the creation of content fingerprints and intent fingerprints, making it possible to group and match similar fingerprints with each other. It makes millions of documents accessible by leading users to where they want to be, going beyond brute force free text search. R discovery, one of our apps powered by this engine, recently hit 1M+ downloads
Language and Phrase Bank Query Engine
It supports users during the drafting stage by helping them reference contextual word usage and grammar from published sources and make informed decisions
SoTA Language Model for Text Infiling for Academic Data Corpus
These are custom-trained large transformer models which are also in the range of billions of parameters. These are specifically trained on academic data and work much, much better than general-purpose language editors
AI as expert systems are evolving more towards assistive and pseudo-automatic stages in very shallow applications, given that people have realised it's better to solve small than to build generic systems. Recent trends emerging in the field are
Augmented Reality (AR) and smart glasses
Since the release of, among others, the Google Glass and Microsoft HoloLens applications, in the last few years, there have been significant advances in AR. This year, various organizations announced the release of their AR glasses products. Even companies like Ray-Ban, which are fashion-first, are foraying into smart glasses. These smart glasses allow people to interact and work in a real-world simulated environment. Over the next 5 years, we'll see.
Responsible And Ethical AI
If a self-driving car is faced with two choices, both of which result in some harm to a human, which decision should the model make? Should it be based on data OR should there be some override rule? If a very novel advancement in AI has been made, is it okay for it to be used in a military application that will eventually be used in warfare? These are some of the questions, along with bias, data protection, discrimination, etc., that responsible and ethical AI attempts to address. There is a strong movement around the ethical use of AI, and many companies are creating dedicated task forces and coalitions that deal with this.
AI Explainability
AI models, especially those that deal with larger derived dimensions of data and data gathered from various touchpoints, are largely deep-learning model black boxes. The data go in and the decision (output) comes out. There is very little reasoning behind why a certain decision was made. As we move into the future where AI is being used in applications such as medical diagnosis, self-driving vehicles, automated trading, and even in recruitment and other decision-making functions, it becomes important to ensure transparency and visibility on why a certain machine-learned model reached a particular decision. There are many open-source tools and frameworks that have yielded good early results in the interpretation of AI models.
Fairness
Privacy/ Federated Learning
Join our WhatsApp Channel to get the latest news, exclusives and videos on WhatsApp
_____________
Disclaimer: Analytics Insight does not provide financial advice or guidance. Also note that the cryptocurrencies mentioned/listed on the website could potentially be scams, i.e. designed to induce you to invest financial resources that may be lost forever and not be recoverable once investments are made. You are responsible for conducting your own research (DYOR) before making any investments. Read more here.