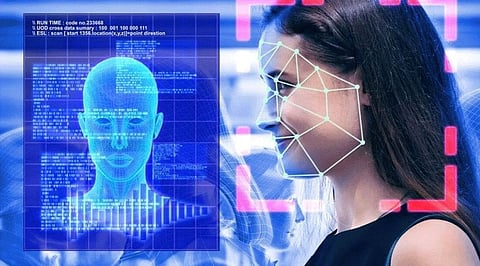
Emotional AI is taking artificial intelligence to the next level with devices that can understand human moods and emotions. With devices listening to everything, the concern for privacy is now more than ever. From smartphones to smart home devices and work appliances, the technology around us in day-to-day life is so advanced that it is noting our conversations, which sometimes seems like technology is crossing a line.
A common example, in this case, is mobile apps and virtual assistants that can recognize emotions in real-time and adapt to the user's mood. While the logic behind this snooping is that ML software can create more natural conversations with human-like understanding, where can the users draw a line when the audio stored contains sensitive information that one might not want to disclose to anyone?
The discussion about ethical AI is a good starting point. In a paper by CSE Ph.D. student Mimansa Jaiswal and Prof. Emily Mower Provost, they propose a method to enable secure ML technologies. By using adversarial ML, they've shown that ML technology can be powered with an ability to unlearn sensitive information like gender identifiers before it is stored and use altered representations of the user to train emotional AI models.
Emotion recognition and sentiment analysis are two technologies that automatically identify and analyze complex features of speech work with the machine learning models that are trained using large piles of labeled data. To be accurate, the ML model has to undergo training that will help it understand common features of human speech.
Devices that smartphones are exposed to various forms of human speech, as the recording of conversations. According to Jaiswal, "the hope of this paper is to show that these machine learning algorithms end up encoding quite a lot of information about a person's gender or demographic information." This information is stored on company servers that leverage a specific app or a voice assistant, leaving the information open for company or hackers who might attack the company's servers for some information.
The authors believe that the consequence of leaking sensitive information will be huge.
Sometimes, apps provide options to the user to opt-out of these services, but some devices can override this option. The solution to this issue is moving the data to the cloud, after pre-processing. To encode audio, previous attempts were made by adding random noise to the datasets. This technique only works if the listener has no idea about the kind of noise added. If the attacker could figure out the source of the noise, that person can collect all the information.
The paper suggests another way. Before the audio is even stored, information about demographics and other sensitive topics can be removed. What remains is the abstract version of the original recording. To make this happen, professionals have to ensure that this new technique can still be used to train ML models effectively.
After testing, the authors found out that "the performance is either maintained or there is a slight decrease in performance for some setups." In some instances, they even noticed an increase in performance, implying that the ML model is unaffected by gender.
"ML models are mostly black-box models, meaning you don't usually know what exactly they encode, what information they have, or whether that information can be used in a good or malicious way. The next step is to understand the difference in information being encoded between two models where the only difference is the one has been trained to protect privacy", concluded the authors of the paper.
Join our WhatsApp Channel to get the latest news, exclusives and videos on WhatsApp
_____________
Disclaimer: Analytics Insight does not provide financial advice or guidance. Also note that the cryptocurrencies mentioned/listed on the website could potentially be scams, i.e. designed to induce you to invest financial resources that may be lost forever and not be recoverable once investments are made. You are responsible for conducting your own research (DYOR) before making any investments. Read more here.