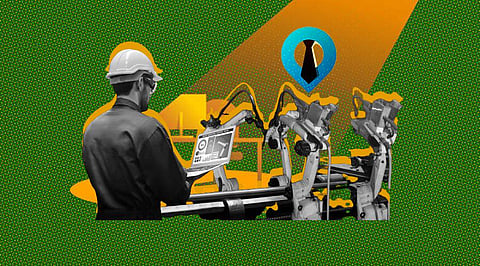
Does that mean Data Scientist hiring has reached its peak? A Forbes report states that in the past 10 years data scientist hiring has drastically come down. There will be no data science posts left by the end of 2029. The reason it states is that data science as a discipline has reached the peak of its technology hype cycle and is currently dwelling in its transition phase. Companies almost went wrong in adapting to proof of concept so much so that they ended up hiring statisticians who can code. Academics were given too much precedence over practical skills only to end up with models which never made it to the production stage. It's not that only presumptions about data science were responsible but also the ignorance around machine learning technology – which mostly got the black box treatment – was the culprit. A successful business model requires practical knowledge and therefore companies are into posting more machine learning jobs. Though reluctant to accept it in the beginning, companies, and business managers are slowly adapting to the reality: machine learning solutions do not require statisticians who are specialists in theories but engineers who can put those theories into practice.
For advanced machine learning companies particularly large tech or fintech companies, the demand for ML engineers is huge for the complexity of its services. More the complexity, the more nuanced machine learning engineers are required. This increase in complexity is due to the increase in the amount of cross-connected data that puts limits on the processing speed. Certain domains such as fraud detection require machine learning tools to process data from massive data to draw inferences from dynamic sources. It needs fine-tuning the machine learning model from time to time and hence the requirement for machine learning engineers. In computations that require less than a few milliseconds, only a deeper neural network and complex engineering could generate the desired results, all while adhering to the constraints.
Companies develop AI applications with the sole aim to take them to the market, predominantly to generate revenue. Data Scientists with statistical skills can only predict what variables are favorable for a certain product but cannot identify the path towards achieving the goal. Whereas ML engineers can take a business idea, identify a viable approach from several available software options, and take it to its culmination point after testing and improvising it, thus making a product more accessible. The demand for machine learning engineers depends on where the company lies on the service as a platform and customized product development spectrum. The more the company leans towards service as a platform, the more the demand for ML engineer jobs; and businesses just want to be a platform as all the action lies in knitting the parts together while picking up the required components from the cloud.
Intelligent process automation is expected to be a growing trend according to the 2020 McKinsey Global Survey, thereby increasing the demand for machine learning experts. Of the 1,179 companies polled, 66% said they were looking forward to implementing business automation and it is an increase from 57% who expressed interest in the same in 2018. Therefore, companies that want to be ahead of the curve would warm up to machine learning by hiring more machine learning engineers. However, it doesn't mean it will end the spell of data science as a skill though it cannot be held up as a job title putting an end to the data scientists Vs ML engineer's debate.
Join our WhatsApp Channel to get the latest news, exclusives and videos on WhatsApp
_____________
Disclaimer: Analytics Insight does not provide financial advice or guidance. Also note that the cryptocurrencies mentioned/listed on the website could potentially be scams, i.e. designed to induce you to invest financial resources that may be lost forever and not be recoverable once investments are made. You are responsible for conducting your own research (DYOR) before making any investments. Read more here.