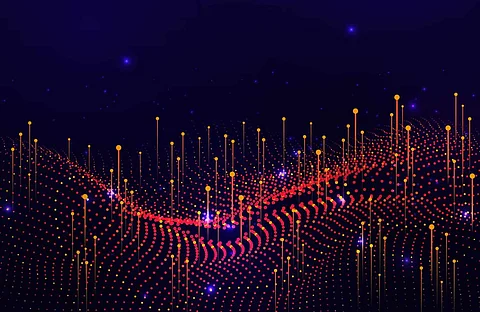
Data virtualization uses a simple three-step process—connect, combine, consume, to deliver a holistic gamut of intelligent information to business users.
Big Data is both structured and unstructured coming from rows and columns integrated into a traditional database, in formats like social media, logs and email content. Big Data in its many forms is stored in databases, log files, CRM, SaaS, and other apps.
So how do enterprises get an overview of their data and manage it in all of its disparate forms? They deploy data virtualization, a banquet term to describe master data management leveraging for data manipulation and retrieval.
Data virtualization integrates data from disparate sources without copying or moving the data, thus giving users a single virtual layer that spans multiple formats, physical locations and applications, which means breaking data silos and quicker access to data pipelines.
Data Virtualization is the ultimate big data integration because it breaks down condensed data, performs data replication and federation in a real-time format, allowing for greater speed and agility and response time. It helps with data mining, enabling effective data analytics, which is a critical success factor for predictive analytics tools. Effective use of machine learning and AI is unlikely without data virtualization.
Data virtualization connects to all types of data sources— cloud applications, big data repositories, excel files, databases and data warehouses.
Data virtualization combines intelligent information into business views irrespective of their data format which may include Hadoop, web services, Cloud APIs, relational databases, noSQL, etc.
Data virtualization lets business users consume data through multiple portals, mobile apps, web applications, reports and dashboards.
Data virtualization has many uses, like data integration, logical data warehouses, big data and predictive analytics. Data Integration is the most likely case enterprises encounter since they all have data coming from multiple data sources. This means bridging old data sources, housed in a client/server setup, with new digital systems like social media and integrating connections, like Java DAO, ODBC, SOAP, or other APIs, and search enterprise data with a data catalogue.
Here is how Data virtualization is bringing a new era into demystifying data silos
Data Virtualization can be used for data preparation before getting into the data lakes, not just restricted as a delivery layer on the outbound side of the data lake or data warehouse providing a seamless access to centralized data quality services. This enables data managers to replace multiple single-use services across the enterprise. The combination of metadata management and data catalogue capabilities allow to get closer to data democratization by helping an enterprise's internal customers discover, govern, and access data in ways they could not access before.
Join our WhatsApp Channel to get the latest news, exclusives and videos on WhatsApp
_____________
Disclaimer: Analytics Insight does not provide financial advice or guidance. Also note that the cryptocurrencies mentioned/listed on the website could potentially be scams, i.e. designed to induce you to invest financial resources that may be lost forever and not be recoverable once investments are made. You are responsible for conducting your own research (DYOR) before making any investments. Read more here.