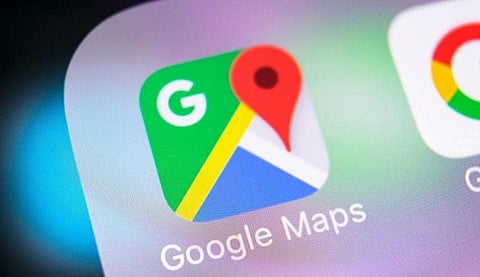
DeepMind is one of the companies that are leading the AI charge and coming up with innovative uses of AI. This London-based AI lab has been under the umbrella of Alphabet since the latter acquired it in January 2014. While Google's AI ventures have been keeping it running, DeepMind is most helpful when it comes to Google Maps. For years, it has been a challenge to design a machine-learning algorithm to train AI models and softwares to help in navigation, especially in unstructured surroundings. Therefore understanding how AI can learn about cruising through an environment and guide us in the future is always an area of interest for researchers.
The reason why it is an arduous task is primarily that long-range navigation is a complex cognitive task that relies on developing an internal representation of space, grounded by familiar landmarks and robust visual processing, that can simultaneously support continuous self-localization ("I am here") and a representation of the goal ("I am going there"). This is where DeepMind's deep reinforcement learning helps to solve the hitch. Besides, it is essential to address this as people rely on the accuracy of Google Maps to assist them. Every day, this app provides useful directions, real-time traffic information, and information on businesses to millions of people, along with accurate traffic predictions and estimated times of arrival (ETAs). As a result, it is crucial to mirror the ever-changing landscape of urban lands.
Recently, researchers at DeepMind teamed up with Google Maps to improve the accuracy of real-time ETAs by up to 50% in places like Berlin, Jakarta, São Paulo, Sydney, Tokyo, and Washington D.C. by using advanced machine learning techniques. At present, the Google Maps traffic prediction system consists of a route analyzer for processing traffic information to construct Supersegments (multiple adjacent segments of road that share significant traffic volume). It also has a Graph Neural Network model, which is optimized with various objectives and predicts the travel time for each Supersegment.
The data collected to train the machine learning model of DeepMind was extracted from authoritative data input from local governments and real-time feedback from users. The authoritative data lets Google Maps learn about speed limits, tolls, or road restrictions due to things like construction, excavation works, or COVID-19 shutdown. Meanwhile, feedback from users lets Google know that paved roads are better for driving than unpaved ones. It also helps Google to make a neural network model opt a long stretch of highway as efficient routes than a smaller shortcut road with multiple stops.
After collecting the data, in the Graph Neural Network, the model considers the local road network as a graph, with each route segment resembling as a node and edges that exist between segments that are consecutive on the same road or connected through an intersection. When a message-passing algorithm gets executed, neural networks learned those messages and studied their effect on node states and edge. Now, in the real world, these Supersegments are road subgraphs, which were sampled at random in proportion to traffic density. When a single model was successfully trained via these subgraphs, the algorithm was then deployed at scale.
Through Graph Neural Network, researchers were able to carry spatiotemporal reasoning by incorporating relational learning biases to model the connectivity structure of real-world road networks. Google Maps product manager Johann Lau says, "We saw up to a 50 percent decrease in worldwide traffic when lockdowns started in early 2020. To account for this sudden change, we've recently updated our models to become more agile — automatically prioritizing historical traffic patterns from the last two to four weeks, and deprioritizing patterns from any time before that.
Join our WhatsApp Channel to get the latest news, exclusives and videos on WhatsApp
_____________
Disclaimer: Analytics Insight does not provide financial advice or guidance. Also note that the cryptocurrencies mentioned/listed on the website could potentially be scams, i.e. designed to induce you to invest financial resources that may be lost forever and not be recoverable once investments are made. You are responsible for conducting your own research (DYOR) before making any investments. Read more here.