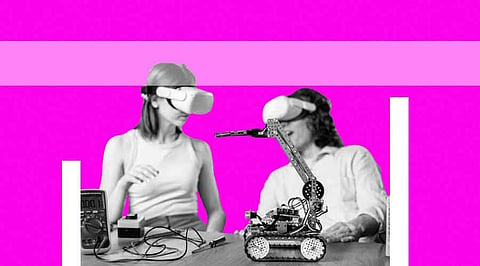
In a machine learning approach called reinforcement learning, an agent learns how to operate in a given environment by taking particular behaviors and watching the rewards it receives. It is a form of trial-and-error learning where the agent uses their prior mistakes to inform future judgments. We will examine the fundamentals of reinforcement learning in this blog, as well as its models, methods, applications, and difficulties. We will also explore the significance of Reinforcement Learning in the field of artificial intelligence and how it could influence the future.
As we take you on a tour through the realm of reinforcement learning, please clasp up! The environment in which an agent functions is specified using reinforcement learning models. They offer a framework for comprehending how an agent's activities, the condition of the environment, and the rewards they get are related. Four of the most popular Reinforcement Learning models—Markov Decision Process (MDP), Semi-MDP, Partially Observable Markov Decision Process (POMDP), and Multi-Agent Reinforcement Learning—will be covered in this section.
The most fundamental Reinforcement Learning model, the Markov Decision Process (MDP), involves an agent interacting with the environment and being rewarded for its actions. MDP presupposes that the environment is completely observable, which means that the agent has full knowledge of the environment's current state. Semi-MDP: Semi-MDPs are an extension of MDPs in which the probability of the states transitioning are unknown. In circumstances where the environment is only partially visible, this paradigm is helpful. POMDPs are employed when the environment is only partially visible and the agent does not have a complete understanding of the environment's state. In this paradigm, the agent makes judgments based on its prior observations and actions. When several agents are working together in the same environment, they employ the multi-agent reinforcement learning paradigm to learn from and interact with the surroundings. This paradigm allows for cooperative or antagonistic interactions between the agents, and it also allows for the agents to learn from one another's experiences.
The foundation of the reinforcement learning process are reinforcement learning algorithms. These algorithms are used to identify the optimum course of action that maximizes expected reward over time while mapping states to actions. Q-Learning, SARSA, Deep Reinforcement Learning, and Evolutionary Algorithms are the four Reinforcement Learning algorithms that are most frequently utilized.
One of the most adaptable machine learning approaches is reinforcement learning, which has many uses in a variety of professions and sectors. Robotics use reinforcement learning to teach machines to carry out tasks like grabbing items, navigating, and manipulating. The agent develops these skills by acting and monitoring the rewards it receives, which enables it to gradually improve its behavior. Gaming has effectively used reinforcement learning, especially in games of strategy like chess and go. By learning from their mistakes and improving their decision-making over time, reinforcement learning algorithms are used to train agents to play games at a superhuman level.
There are several uses for reinforcement learning across numerous disciplines and sectors. Reinforcement Learning is proven to be a versatile and effective method for optimizing decision-making and behavior, whether it is in robots, gaming, finance, or healthcare. There are countless applications, therefore Reinforcement Learning will likely be used in a lot more industries in the future.
Join our WhatsApp Channel to get the latest news, exclusives and videos on WhatsApp
_____________
Disclaimer: Analytics Insight does not provide financial advice or guidance. Also note that the cryptocurrencies mentioned/listed on the website could potentially be scams, i.e. designed to induce you to invest financial resources that may be lost forever and not be recoverable once investments are made. You are responsible for conducting your own research (DYOR) before making any investments. Read more here.