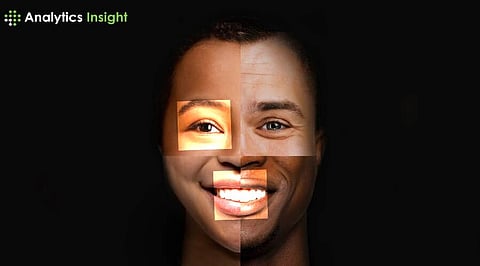
Facial recognition technology, powered by deep learning algorithms, has emerged as a groundbreaking application with widespread implications across various industries. From unlocking smartphones to enhancing security systems and even aiding law enforcement, the capabilities of facial recognition are vast. However, the implementation of deep learning in facial recognition is not without its challenges. In this article, we explore the hurdles faced by this technology and delve into innovative solutions that are shaping the future of facial recognition.
One of the foremost challenges in training robust facial recognition models is the availability and quality of diverse datasets. Deep learning algorithms heavily rely on large and varied datasets to generalize well across different demographics, ethnicities, and environmental conditions. Biases can emerge if the training data is not representative, leading to inaccurate and unfair results, especially for underrepresented groups.
Solution: To address this challenge, researchers are advocating for more inclusive and diverse datasets. Efforts are being made to collect facial data from a wide range of sources to ensure models are exposed to a rich set of features, reducing biases and improving overall accuracy.
The increased adoption of facial recognition has sparked concerns about privacy and the potential misuse of personal information. Deep learning models, when deployed without proper safeguards, can infringe on individuals' privacy rights. There are concerns about the unauthorized use of facial data and the creation of comprehensive databases that could be exploited for surveillance purposes.
Solution: Privacy-preserving techniques such as federated learning and differential privacy are being explored to strike a balance between effective facial recognition and protecting individuals' privacy. These methods allow models to be trained across decentralized devices, minimizing the need for central databases and reducing the risk of misuse.
Deep learning models, including those used in facial recognition, are vulnerable to adversarial attacks. These attacks involve manipulating input data with imperceptible changes to deceive the model into making incorrect predictions. For facial recognition, this could lead to unauthorized access or identity theft.
Solution: Researchers are developing robustness mechanisms, including adversarial training, to fortify facial recognition models against attacks. By incorporating adversarial examples during training, models become more resilient to subtle manipulations, enhancing their real-world performance.
Deep learning models, especially complex neural networks used in facial recognition, demand substantial computational resources. This poses challenges for real-time applications, as processing power and memory constraints can hinder the deployment of these models on resource-constrained devices.
Solution: Edge computing and optimization techniques are being employed to address hardware limitations. By offloading some computation to local devices, facial recognition systems can achieve real-time processing without overburdening central servers.
Transfer learning has proven to be a game-changer in addressing the data scarcity issue. By pre-training models on large datasets for related tasks, such as object recognition, and fine-tuning them for facial recognition, researchers can achieve impressive results even with limited facial data. This approach helps mitigate biases and improves the generalization capabilities of facial recognition models.
The lack of transparency in deep learning models has been a significant concern. Explainable AI (XAI) methods are now being integrated into facial recognition systems to provide insights into how decisions are made. This not only enhances trust in the technology but also allows for the identification and correction of biased behaviors.
To address the ethical concerns surrounding facial recognition, there is a growing emphasis on establishing clear guidelines and regulations. Governments and organizations are working together to define ethical frameworks that govern the responsible use of facial recognition technology. This includes limitations on data storage, transparency in usage, and measures to ensure fairness and accountability.
Facial recognition systems are not static; they must adapt to evolving environments and changing demographics. Continuous monitoring and regular updates to the training datasets and algorithms are crucial to maintaining accuracy and preventing biases from emerging over time.
Deep learning has propelled facial recognition technology to unprecedented heights, revolutionizing the way we interact with security systems, devices, and public spaces. However, the path is not without hurdles. By addressing issues related to data quality, privacy concerns, adversarial attacks, and hardware limitations, researchers and developers are paving the way for a more robust and responsible era of facial recognition technology. Through innovative solutions such as transfer learning, explainable AI, ethical guidelines, and continuous monitoring, the industry is working towards creating facial recognition systems that are not only accurate but also fair, transparent, and respectful of individual privacy rights.
Disclaimer: Analytics Insight does not provide financial advice or guidance. Also note that the cryptocurrencies mentioned/listed on the website could potentially be scams, i.e. designed to induce you to invest financial resources that may be lost forever and not be recoverable once investments are made. You are responsible for conducting your own research (DYOR) before making any investments. Read more here.