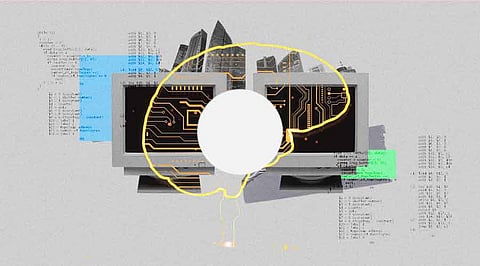
As Machine Learning (ML) gains traction in the market, Deep Learning (DL), another cutting-edge field of study in data science, is on the rise. A division of machine learning is called deep learning. When educated with a large quantity of data, Deep Learning systems can match (and perhaps surpass) the cognitive abilities of the human brain, which is what makes deep learning so special. Naturally, data scientists working in this cutting-edge field of learning got busy creating a variety of logical Deep Learning frameworks. These Deep Learning frameworks, which can be either an interface or a library/tool, make it much easier for Data Scientists and ML Developers to create Deep Learning models.
1. TensorFlow, an open-source platform from Google, is perhaps the most well-liked machine learning and deep learning technology. TensorFlow is a JavaScript-based framework that includes several tools and community resources to make it simple to train and use ML/DL models. TensorFlow Lite may be used to deploy models on mobile or embedded devices while the main tool allows you to construct and deploy models on browsers. Additionally, TensorFlow Extended may be used to train, create, and deploy ML/DL models in large production contexts. This framework for deep learning is excellent.
2. Keras is another open-source deep learning framework that we have included. This useful tool may be used with PlaidML, Theano, Microsoft Cognitive Toolkit, and TensorFlow. The unique selling point of Keras is its speed. Because it has built-in parallelism support, it can analyze enormous amounts of data while reducing model training time. It is really simple to use and extendable because it was designed in Python. This framework for deep learning is excellent.
3. Sonnet is a high-level toolkit created by DeepMind for creating intricate neural network architectures in TensorFlow. The fundamental Python objects corresponding to each individual component of a neural network are developed and created by Sonnet. The computational TensorFlow graph is then separately linked to these items. The creation of high-level architectures is made easier by this method of independently generating Python objects and connecting them to a graph. One of the better Deep Learning frameworks available is this one.
4. A cutting-edge platform called Swift for TensorFlow combines the strength of TensorFlow with that of the Swift programming language. Swift for TensorFlow integrates all the most recent findings in machine learning, differentiable programming, compilers, systems architecture, and much more because it was created exclusively for machine learning. The project is available to anybody who wants to play with it, even if it is still in its infancy.
5. MXNet is an open-source deep learning framework made for deep neural network deployment and training. As a result of its tremendous scalability, it encourages quick model training. It supports several programming languages, including C++, Python, Julia, Matlab, JavaScript, Go, R, Scala, Perl, and Wolfram, in addition to having a flexible programming style. This fantastic deep learning platform might be very helpful to you.
6. On top of the NumPy and CuPy libraries, Chainer is an open-source deep learning system built in Python. The define-by-run method was initially introduced by this Deep Learning framework. In this method, the fixed connections between the network's mathematical operations (such as matrix multiplication and nonlinear activations) must first be defined. The actual training calculation is then executed. You may successfully navigate deep learning interviews by being familiar with these frameworks, and you'll even be able to tell which of the following is not a deep learning framework.
7. Facebook created the open-source Deep Learning framework PyTorch. It was created with the single goal of speeding up the complete process from research prototype to production deployment. It is built on the Torch library. The intriguing thing about PyTorch is that it has a Python interface on top of a C++ frontend. The torch backend supports scalable distributed training and performance optimization in both research and production, and the frontend acts as the fundamental building block for model construction. One of the better deep learning frameworks available is this one.
8. A distributed Deep Learning library for Java and the JVM, Deeplearning4J (DL4J), was created. As a result, it works with all JVM languages, including Scala, Clojure, and Kotlin. The basic calculations of DL4J are programmed in C, C++, and Cuda. In order to speed up model training and integrate AI into business contexts for usage on distributed CPUs and GPUs, the platform combines both Apache Spark and Hadoop. In fact, it can do just as well as Caffe on several GPUs.
9. Gluon is an open-source Deep Learning interface that has only recently been added to the list of Deep Learning frameworks. It aids developers in creating machine learning models rapidly and efficiently. ML/DL models may be defined utilizing a selection of pre-built and optimized neural network components using a clear and succinct API. Users may define neural networks using Gluon using short, clear, and easy code. It includes an entire set of plug-and-play building components for neural networks, such as initializers, optimizers, and preconfigured layers. These assist in removing many of the intricate implementation details that lie behind.
10. Microsoft and Facebook created the ONNX initiative, which stands for Open Neural Network Exchange. It is an open ecosystem created for the creation and dissemination of machine learning and deep learning models. Along with specifications of built-in operators and common data types, it also offers a definition of an extendable computation graph model. Models may be trained in one framework and then transferred to another for inference thanks to ONNX, which streamlines the process of doing so.
Join our WhatsApp Channel to get the latest news, exclusives and videos on WhatsApp
_____________
Disclaimer: Analytics Insight does not provide financial advice or guidance. Also note that the cryptocurrencies mentioned/listed on the website could potentially be scams, i.e. designed to induce you to invest financial resources that may be lost forever and not be recoverable once investments are made. You are responsible for conducting your own research (DYOR) before making any investments. Read more here.