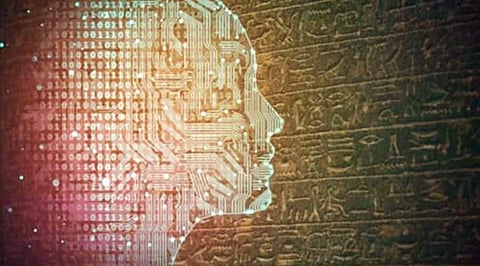
Researchers built a system that can interpret lost ancient scripts automatically.
There is no doubt there were several ancient languages existed back then that are now either vanishing or already became extinct. Such languages that no longer exist were the native language of particular communities. Research shows that about six thousand languages are spoken in the world currently. Though it is hard to come up with exact numbers, linguists estimated around 31,000 languages have existed in the human history.
The research further revealed that more than half of the languages spoken today have fewer than 10,000 speakers; similar to the population of Wasilla, Alaska. On the other side, nearly 82 % of languages have fewer speakers than people in Waco, Texas. Considering this fact, linguists envisions that at least half the world's languages will become extinct in the next one hundred years.
While lost ancient languages are more than a mere academic inquisitiveness, humans miss a complete knowledge about the people who spoke them. Thus, in an effort to decode such languages, researchers at MIT's Computer Science and Artificial Intelligence Laboratory (CSAIL) developed a system that is claimed to be able to automatically decipher a lost language,
Spearheaded by MIT Professor Regina Barzilay, the system works on numerous principles grounded in insights from historical linguistics, such as the fact that languages generally only evolve in certain predictable ways. For instance, when a given language rarely adds or deletes an entire sound, certain sound substitutions are likely to occur. A word with a "p" in the parent language may convert into a "b" in the descendant language, but changing to a "k" is less likely owing to the significant pronunciation gap.
To this context, Barzilay and MIT Ph.D. student Jiaming Luo built a decipherment model that can handle the vast space of possible transformations and the dearth of a guiding signal in the input. This project has developed on a paper they wrote last year that decrypted the dead languages of Ugaritic and Linear B, a syllabic language related to ancient Greek, where their model correctly translated 67.3% of cognates.
According to the report, most undeciphered lost languages exhibit two characteristics that pose significant decipherment challenges. First, the scripts are not fully segmented into words, and second, the closest known language is not determined. In this way, the proposed decipherment model is claimed to handle both challenges by building on rich linguistic constraints reflecting consistent patterns in historical sound change.
The model is also able to analyze the proximity between two languages. When tested on known languages, it can even accurately identify language families. Researchers applied their decipherment model to Iberian considering Basque, as well as less-likely candidates from Romance, Germanic, Turkic, and Uralic families. They found that Basque and Latin were closer to Iberian than other languages, despite they were still too distinct to be considered related.
Moreover, researchers hope to expand their work beyond the act of correlating texts to related words in a known language in the future.