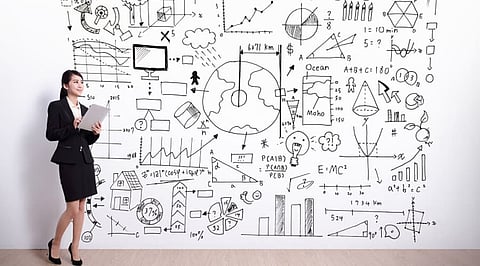
Data engineers are inquisitive, competent problem solvers who enjoy both data and creating helpful things for people. In any case, data engineers, along with data scientists and analysts, are part of a squad that converts raw data into information that gives their companies a competitive advantage. In this article, you will learn about the difference between data engineer and data scientist along with why you should choose data engineer over data scientist.
A data engineer is in charge of establishing and maintaining the data architecture and infrastructure that underpins an organization's IT systems and environments. Programming, data storage, database management, and system implementation are all skills that data engineers must have.
A data scientist is a person who analyses massive data using statistical tools and methodologies, particularly artificial intelligence and machine learning. Data scientists' job is critical to modern technology firms, whether it's assisting Facebook in determining which advertisements to display you or advising Netflix on which films and TV shows to recommend.
When it comes to skills and duties, data engineers and data scientists have a lot in common. The most significant distinction is one of concentration. Data engineers are responsible for developing data generating infrastructure and architecture. Data scientists, on the other hand, are concerned with sophisticated mathematics and statistical analysis of the data collected.
Data scientists deal with the infrastructure that is developed and maintained by data engineers on a regular basis, but they are not accountable for it. Alternatively, they are internal clients charged with doing high-level market and business operation research in order to discover patterns and relationships tasks that need them to engage with and act on data using a range of sophisticated technologies and techniques.
Data engineers, on the other hand, help data scientists and analysts by providing infrastructure and tools that may be utilised to create end-to-end business solutions. Data engineers design batch and real-time analysis of data collected, as well as scalable, high-performance systems for delivering solid business data from raw data sources. They also conduct complicated analytical projects with an emphasis on gathering, managing, analysing, and displaying data.
To put it another way, data scientists rely on data engineers. Data scientists work with advanced analysis tools like R, SPSS, Hadoop, and complex statistical modelling, while data engineers work with the solutions that enable such tools. NoSQL, SQL, MySQL, Cassandra, and other data organisation services, for example, may be in a data engineer's toolkit.
A data engineer creates, designs, tests, and maintains infrastructures like databases and large-scale processing technologies. On the other side, a data scientist is somebody who cleans, massages, and organises (huge) data.
You might think the verb "massage" to be particularly strange, but it simply emphasises the distinction between data engineers and data scientists.
In general, the efforts required by both parties to extract the data into a useable format will be vastly different.
Raw data containing human, machine, or instrument mistakes is dealt with by data engineers. The data may not have been verified and may contain suspicious records; it will be unformatted and may include system-specific codes.
Data engineers will be responsible for recommending and, on occasion, implementing methods to increase data dependability, efficiency, and quality. To do so, they'll need to use a range of languages and tools to connect systems or look for ways to obtain fresh data from other networks so that system-specific codes, for example, may be turned into information for data scientists to analyse.
The notion that data engineers will need to guarantee that the architecture in place meets the requirements of the data scientists and the interested parties, the business, is intimately connected to these two.
Finally, the data engineering group will need to create data set procedures for data modelling, mining, and production in order to send the data to the data science team.
Data scientists are typically given data that has already been cleaned and manipulated, which they may feed into sophisticated analytics tools and machine learning and statistical approaches to prepare data for descriptive and predictive modelling. To create models, they will need to conduct industry and business research, as well as utilise huge amounts of data from internal and external sources to respond to business demands. This can also include data exploration and examination in order to uncover hidden patterns.
After the data scientists have completed their analysis, they must provide a clear storey to key stakeholders, and after the results have been accepted, they must ensure that the process is automated so that the insights can be supplied to business stakeholders on a regular, monthly, or annual basis.
Both sides must clearly collaborate in order to wrangle the data and give insights into business-critical choices. Whereas the data engineer will deal with database systems, data APIs, and technologies for ETL purposes, as well as data modelling and putting up data warehouse options, the data scientist will have to know about stats, arithmetic, and machine learning to create predictive models.
The data scientist must be familiar with distributed computing because he or she will require access to data that has been analyzed by the data engineering team, but he or she will also have to be able to communicate to business stakeholders, which necessitates an emphasis on narrative and visualisation.
In comparison to other data-driven occupations, data engineers are in great demand. In some ways, this is a step forward for the field as a whole. When machine learning became popular 5-8 years ago, businesses realised they needed individuals who could create data classifiers. However, frameworks such as Tensorflow and PyTorch grew extremely popular, making deep learning and machine learning more accessible to the general public. The data modelling skills became commoditized as a result of this. Data issues are now the bottleneck in assisting organisations in implementing machine learning and modelling ideas into production.
Join our WhatsApp Channel to get the latest news, exclusives and videos on WhatsApp
_____________
Disclaimer: Analytics Insight does not provide financial advice or guidance. Also note that the cryptocurrencies mentioned/listed on the website could potentially be scams, i.e. designed to induce you to invest financial resources that may be lost forever and not be recoverable once investments are made. You are responsible for conducting your own research (DYOR) before making any investments. Read more here.