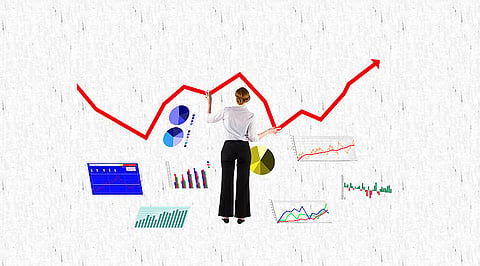
Quality of decision-making is directly proportional to the quality of information one has, and hence Data Science is not an exception. Inappropriate or siloed data is one of the top most reasons a data science project fails. What bad data can do? It should be of no consequence one may ponder. As per an IBM report, bad data can cost the US economy $3.1 trillion per year. These costs include the time employees spend correcting bad data that make for errors. That apart, poor-quality data can actually, result in wrong decisions the way blind spots do. New technologies like artificial intelligence and machine learning, and automation, literally thrive on data and the lack of quality data means death for these industries. In fact, data quality is the one significant factor that remains a hurdle in achieving the near sentient generative AI, a promising sphere in artificial intelligence. New Vantage Partner's recent survey of senior executives revealed that more than three-fourths of respondents said that the increase in data volumes and sources is driving the increase in investments in AI and cognitive learning.
With data management techniques getting more sophisticated with time businesses are fast integrating data science into their processes instead of treating it as a separate entity. Data dependence has grown in leaps and bounds with obligations towards bringing out novel and competitive products into the market. Data compliance issues also come into the picture when good quality data is concerned. With evolving data compliance regulations, it has become quite essential for companies to follow proper data management techniques.
As in data analytics no problem has a direct solution, measuring data quality too is a complex one. A set of factors to measure data quality. Determining if a data set is of high quality purely depends on the context of the problem at hand. A data set that proves to be premium for one project can be outright crap for another. Calculating the quality always depends on certain factors and the purpose of the project, however, there will always be ambiguities in determining the extent. For an optimal calculation, one can depend on characteristics of quality like validity, consistency, accuracy, completeness, uniformity, and relevance. Like said before, there will always be wiggle room in measuring data quality against the parameters. So is there a way to arrive at optimum result?
It is the degree to which a dataset follows the format or set of rules. For example, data is defined by the data type, filling in mandatory data, or following a format for expressing dates, etc.
Accuracy gives the measure of the correctness of data. The data can be of anything from date of birth, bank balance, eye colour, or geographical location. However, it is impossible to measure data accuracy accurately as it is impossible to measure it against any pre-set standards.
Datasets are meant to be comprehensive and exhaustive for the project to be equipped with all the necessary information. Incomplete information is not always about looking for empty cells as they might be holding incomplete information such as half surnames that becomes an issue when the data has to be sorted in alphabetical order.
Data should match when collected from different sources. As it is not possible always to refer to the source, deciding which data is consistent requires a little bit of thinking. It is easy to infer which data is correct by looking at the most recent entry or determining the reliability of the data in some other way.
Data uniformity ensures that the units of measure, metrics and so on are uniform. For instance if you have to combine two datasets on weight then it should be recorded in one particular metric system, FPS, MKS, or CGS. Having this standard is relatively easy compared to others.
A more subjective parameter, it checks whether data is complete, uniform, and consistent. Apart, this is one parameter that ensures the timeliness of the data collected, a major issue most data science projects face today.
Join our WhatsApp Channel to get the latest news, exclusives and videos on WhatsApp
_____________
Disclaimer: Analytics Insight does not provide financial advice or guidance. Also note that the cryptocurrencies mentioned/listed on the website could potentially be scams, i.e. designed to induce you to invest financial resources that may be lost forever and not be recoverable once investments are made. You are responsible for conducting your own research (DYOR) before making any investments. Read more here.