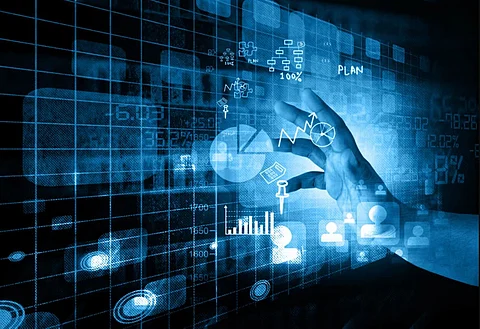
Since data science is expansive, with strategies drawing from computer science, statistics, and different algorithms, and with applications showing up in all areas, these challenge areas address the wide scope of issues spreading over science, innovation, and society. Even however big data is the highlight of operations as of 2020, there are still likely issues or difficulties the analysts can address. A few of these issues overlap with the data science field.
A lot of questions are raised concerning the challenging research issues about data science. To answer these questions we have to identify the research challenge areas which the researchers and data scientists can focus on to improve the efficiency of research. Below are the top ten research challenge areas which will help to improve the efficiency of data science.
1. Scientific comprehension of learning, particularly deep learning algorithms
As much as we respect the astounding triumphs of deep learning, we despite everything do not have a logical understanding of why deep learning works so well. We don't analyze the numerical properties of deep learning models. We don't have a clue how to clarify why a deep learning model produces one outcome and not another.
It's challenging to understand how vigorous or delicate they are to discomforts to include data deviations. We don't understand how to confirm that deep learning will perform the proposed task well on new input information. Deep learning is a case where experimentation in a field is a long way in front of any sort of hypothetical understanding.
2. Handling synchronized video analytics in a distributed cloud
With the expanded access to the web even in developing nations, videos have turned into a typical medium of information trade. There is a role of the telecom system, administrators, deployment of the Internet of Things (IoT), and CCTVs in boosting this.
Could the current systems be improved with low latency and more preciseness? When the real-time video information is accessible, the question is how the information can be transferred to the cloud, how it can be processed effectively both at the edge and in a distributed cloud?
3. Carefree reasoning
AI is a useful asset to discover patterns and analyze relationships, especially in enormous data sets. While the adoption of AI has opened numerous productive zones of research in economics, sociology, and medicine, these fields require techniques that move past correlational analysis and can handle causal inquiries.
Financial analysts are now returning to casual reasoning by formulating new strategies at the intersection of economics and AI that makes causal induction estimation more productive and adaptable.
Data scientists are simply starting to investigate numerous causal inferences, not simply to overcome a portion of the solid assumptions of causal outcomes, but since most genuine perceptions are because of different factors that interact with one another.
4. Dealing with vulnerability in big data processing
There are different approaches to deal with the vulnerability in big data processing. This incorporates sub-topics, for example, how to gain from low veracity, inadequate/uncertain training data. How to deal with vulnerability with unlabeled information when the volume is high? We can try to utilize dynamic learning, distributed learning, deep learning, and indefinite logic hypothesis to solve these sets of issues.
5. Multiple and heterogeneous information sources
For certain issues, we can gather heaps of information from various data sources to improve our models. Cutting edge data science strategies can't so far handle combining numerous, heterogeneous sources of information to construct a single, precise model.
Since a large number of these data sources may be valuable information, focused examination in consolidating different sources of information will provide a significant impact.
6. Taking care of data and aim of the model for real-time applications
Do we have to run the model on inference information if one realizes that the data pattern is changing and the performance of the model will drop? Would we be able to recognize the aim of the data circulation even before passing the information to the model? If one can recognize the aim, for what reason should one pass the information for inference of models and waste the compute power. This is a convincing research issue to understand at scale in reality.
7. Computerizing front-end phases of the data life cycle
While the enthusiasm in data science is due to a great extent to the triumphs of machine learning, and more explicitly deep learning, before we get the opportunity to utilize AI strategies, we have to set up the data for analysis.
The beginning phases in the data life cycle are still labor-intensive and tedious. Data scientists, utilizing both computational and statistical techniques, need to devise automated strategies that address data cleaning and information brawling, without losing other significant properties.
8. Building domain-sensitive large scale frameworks
Building a large scale domain-sensitive framework is the most recent trend. There are some open-source endeavors to launch. Be that as it may, it requires a ton of effort in gathering the correct set of information and building domain-sensitive frameworks to improve search capacity.
One can pick a research issue in this subject based on the fact that you have a background on search, information graphs, and Natural Language Processing (NLP). This can be applied to all other areas.
9. Protection
Today, the more information we have, the better the model we can design. One approach to get more information is to share information, e.g., numerous parties pool their datasets to assemble all in all a superior model than any one party can construct.
However, much of the time, because of guidelines or privacy concerns, we have to safeguard the confidentiality of each party's dataset. We are just now investigating viable and adaptable ways, utilizing cryptographic and statistical techniques, for different parties to share information and additionally share models to safeguard the security of each party's dataset.
10. Building large scale productive conversational chatbot systems
One specific sector picking up pace is the production of conversational systems, for example, Q&A and Chatbot systems. A great variety of chatbot systems are available in the market. Making them productive and preparing a summary of real-time discussions are still challenging issues.
The multifaceted nature of the issue increases as the scale of business increases. A large amount of research is going on around there. This requires a decent understanding of natural language processing (NLP) and the most recent advances in the world of machine learning.
Join our WhatsApp Channel to get the latest news, exclusives and videos on WhatsApp
_____________
Disclaimer: Analytics Insight does not provide financial advice or guidance. Also note that the cryptocurrencies mentioned/listed on the website could potentially be scams, i.e. designed to induce you to invest financial resources that may be lost forever and not be recoverable once investments are made. You are responsible for conducting your own research (DYOR) before making any investments. Read more here.