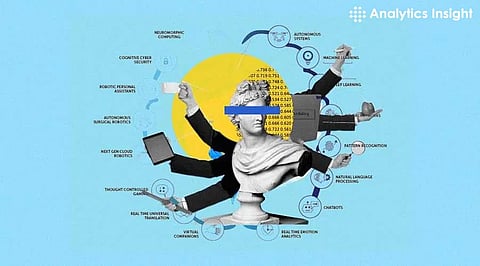
As a complete last-year records creative skill scientist, it is essential to produce substantial solid areas and to demonstrate your capabilities and information to limit administrators. Showing your capacity to utilize measurement driven ways to deal with and tackle genuine issues is similarly essentially as significant as hypothetical information in portfolio projects. Here are the top 10 portfolio project Ideas for data science final years that will help you improve.
One of the top 10 portfolio project ideas for Data Science is Customer Segmentation, it is a fundamental issue of any advancing and displaying device in the consumer department. Customers' demographics, buying behavior, or exceptional relevant talents can be used to group them with the usage of clustering algorithms much like the K-approach and DBSCAN. Your capability to recognize various client corporations and regulate marketing and advertising strategies for that reason might be set up thru this project.
Fake news detection has become increasingly crucial as online misinformation has elevated. Using natural language processing (NLP) strategies, create a model that can distinguish between actual and faux information articles. To assemble an accurate classifier, look into techniques like TF-IDF, phrase embeddings, and recurrent neural networks (RNNs). It belongs to the second top 10 portfolio project ideas for data science final year students.
Sentiment analysis involves extracting and analyzing subjective information from text data to determine the sentiment or opinion expressed. Build a sentiment analysis model using techniques such as bag-of-words, word embeddings, or pre-trained language models like BERT. Apply your model to social media data or product reviews to understand public sentiment.
Create an intelligent chatbot that can converse with users in natural language. Using libraries like TensorFlow or PyTorch, create a chatbot framework and connect it to messaging platforms like Slack or Facebook Messenger. Create a chatbot that can answer questions, provide information, or carry out specific tasks.
Credit card extortion is a goliath challenge for monetary foundations and buyers the same. Create a machine learning model that uses only transactional data and patterns of behavior to identify fraudulent transactions. For organization, look into irregularity recognition calculations or administered research methods like strategic relapse or random timberlands.
Groups need you to wait for customer churn so that you can hold precious customers and reduce income loss. Utilizing historic patron records, bring together a predictive model to discover factors that contribute to churn and forecast destiny churn activities. For accurate churn prediction, use strategies like gradient boosting, choice bushes, or logistic regression.
Data exploration involves analyzing and visualizing datasets to gain insights and identify patterns or trends. To perform exploratory data analysis (EDA) on a private dataset in addition to a public dataset from Kaggle or the UCI Machine Learning Repository, make use of libraries like Pandas, Matplotlib, or Seaborn. It is essential to present your findings in an effective and beneficial manner.
Choose a device studying-to-comprehend venture that makes a speciality of a specific vicinity or problem. Choose a dataset and use the proper gadget learning algorithms to effectively remedy the trouble, whether or not or not you're the use of it for predictive modeling, image class, or time series forecasting. Optimize performance by way of experimenting with various algorithms, hyperparameters, and evaluation metrics.
Discourse acknowledgment innovation empowers PCs to decipher and interpret communication in language into textual content. Utilize deep mastering architectures like convolutional neural networks (CNNs) or recurrent neural networks (RNNs) to assemble a speech recognition machine. Utilizing audio datasets, evaluate the robustness and precision of your model's speech transcription.
New product, carrier, or provider suggestions can be made using recommendation systems that track consumer choices and moves. Using information-based filtering, collaborative filtering, or a combination of the two, a recommendation engine can be built. You can use your picture in product, music, or film proposals based on feedback from customers.
These top 10 portfolio project ideas for Data Science helps students to perform well in their job interviews and achieve their dream job. Having a good portfolio is one of the reasons for being selected in most prominent companies.
For students in their final year of data science, starting portfolio projects is a great way to demonstrate their practical application skills and knowledge. By focusing on various business concepts like customer segmentation, fraud detection, sentiment analysis, and so on, you can demonstrate your proficiency in predictive modeling, machine learning, and data processing. Remember to meticulously document your projects, including the initial steps of data generation, model selection, and business analysis criteria, in order to produce compelling portfolio pieces.
Join our WhatsApp Channel to get the latest news, exclusives and videos on WhatsApp
_____________
Disclaimer: Analytics Insight does not provide financial advice or guidance. Also note that the cryptocurrencies mentioned/listed on the website could potentially be scams, i.e. designed to induce you to invest financial resources that may be lost forever and not be recoverable once investments are made. You are responsible for conducting your own research (DYOR) before making any investments. Read more here.