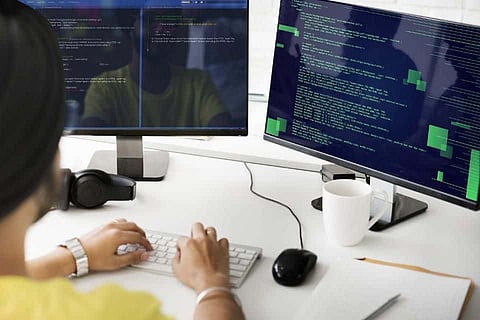
Data science is one of the most in-demand careers in the 21st century. In today's high-tech world, everyone has important questions that "big data" must answer. From businesses to non-profits to government agencies, there is an almost limitless amount of data that can be processed, reviewed, and used for a number of purposes. Continue reading to find out how to become a data scientist and get started on this rewarding career path.
Data science is a complex and often puzzling field that necessitates hundreds of different skills, making it difficult to categorise.
A data scientist is basically someone who collects and analyses data with the objective of obtaining a conclusion. They may provide the data in a visual context, which is commonly referred to as "visualising the data," allowing a user to seek for evident patterns that would be missed if the data was given in raw numbers on a spreadsheet. They frequently develop extremely sophisticated algorithms that are used to identify patterns and transform data from a mess of numbers and statistics into something that may be helpful to a company or organisation. Data science is, at its most basic level, the process of seeking for significance in large volumes of data.
Let's take a look at a common scenario with a data scientist. Perhaps a large corporation, such as a mobile phone provider, wants to determine which of its present customers are most likely to shift to a competitor's service. They may engage a data analyst who can examine millions of data points (or, more precisely, build an algorithm to examine millions of data points) relating to previous consumers. Consumers who consume a particular bandwidth are more likely to quit, or customers who are engaged and between the ages of 35 and 45 are more likely to transfer carriers. To engage and keep these consumers, the mobile phone provider might adjust its business model or marketing activities.
Whenever a Netflix user logs in, they see a real-world example of data management in motion. The video service includes a software that will provide recommendations based on your tastes. An algorithm uses information from your previous watching behaviour to make suggestions for shows you might love.
Future data scientists may start preparing for their careers before even setting foot on a university campus or enrolling in an online degree programme. Ambitious data scientists may get a jump start by learning the most frequently used computer languages in the field, such as Python, Java, and R, as well as brushing up on their understanding of applied math and statistics. In reality, students' learning rates often improve when they attend college with a well-developed skill set. Most significantly, early introduction to data science knowledge needs will help you figure out if a data science profession is suitable for you.
Statistics, computer programming, communication technology, mathematics, or data science, if accessible, are the most sought-after courses in data science. If you're currently enrolled in another undergraduate programme and don't want to move, minoring in one of the disciplines is also a good idea. Continue learning computer languages and database design, as well as adding SQL/MySQL to your "data science to-do list." Now would be the time to start networking professionally by exploring connections within academic groups, looking for internship possibilities, and seeking advice from instructors and advisers.
Companies are frequently on the lookout for someone to fill entry-level data science positions. Jobs such as Junior Data Analyst or Junior Data Scientist can be found by doing a job search. When seeking for entry-level data science employment, system-specific training or qualifications in data-related disciplines (e.g., business intelligence tools, relational database systems, data visualisation tools, etc.) may be beneficial.
Data science is an area where people with advanced degrees, such as a Master's or Ph.D., have a better chance of landing a job. Graduate degrees in data science are in high demand, and they include the same requirements as undergraduate degrees: computer science, data science (if available), information technology, arithmetic, and statistics. Many firms, on the other hand, accept STEM degrees like biotechnology, engineering, and physics (among others). Remember that data scientists need to know how to utilise enterprise-grade data management tools and how networked storage and computing (example, Hadoop, MapReduce, and Spark) work in order to create models and do predictive analytics.
Further education and experience are important elements in being promoted or becoming a highly sought-after data scientist. Businesses place a premium on outcomes. When strong technical abilities are combined with project leadership and management experience, it usually leads to more substantial opportunities and greater pay.
In the ever-changing area of data science, being current is critical. Continuous learning is a buffer against career market changes in this age of rapid technological innovation. This is also true for data science, which isn't as well-known as other analytically and technologically oriented fields. A data scientist with a long career is continuously learning and growing with the industry. Try to network and attend boot camps and conferences to seek out educational and professional opportunities.
Companies are increasingly searching for data scientists to assist them in processing and understanding data. Consider the world's most powerful and influential businesses, such as Apple, Amazon, Google, and Facebook, which all rely heavily on data-driven strategic planning. Amazon, for example, utilises data analytics to power sales and marketing algorithms that propose goods to users based on previous purchases and behaviours. However, Apple makes product selections based on how and when you, the consumer, use its iPhones, iPads, Macbooks, and other products and technology.
Join our WhatsApp Channel to get the latest news, exclusives and videos on WhatsApp
_____________
Disclaimer: Analytics Insight does not provide financial advice or guidance. Also note that the cryptocurrencies mentioned/listed on the website could potentially be scams, i.e. designed to induce you to invest financial resources that may be lost forever and not be recoverable once investments are made. You are responsible for conducting your own research (DYOR) before making any investments. Read more here.