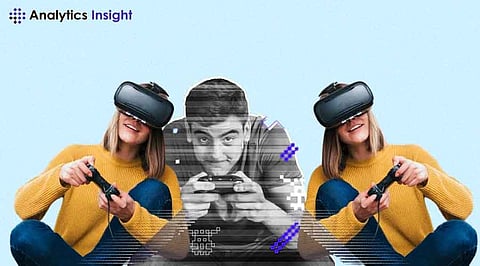
The world of online gaming has grown exponentially over the past few decades, becoming one of the most significant sectors in the entertainment industry. With millions of players around the globe engaging in online games daily, the industry generates vast amounts of data. This data, when harnessed effectively, can provide deep insights into player behavior, preferences, and trends, which in turn drives the development of more engaging and personalized gaming experiences.
Data science plays a crucial role in this process, offering the tools and methodologies needed to analyze and interpret the data generated by online games. This comprehensive guide explores how data science is used in online gaming, highlighting the various applications, techniques, and impacts on the industry.
Data science in online gaming involves the collection, analysis, and interpretation of data generated by players and game environments. This data can include information about player interactions, in-game events, purchases, and social behaviors. By applying data science techniques, game developers and companies can gain insights that help them improve game design, enhance user experiences, and optimize monetization strategies.
The use of data science in online gaming is driven by several factors:
Player Behavior Analysis: Understanding how players interact with a game is critical for improving gameplay and retaining users. Data science allows developers to analyze patterns in player behavior, identify popular features, and detect potential issues that may cause players to disengage.
Personalization: Modern gamers expect personalized experiences that cater to their individual preferences. Data science enables the creation of customized content, recommendations, and gameplay experiences that align with each player's unique tastes and habits.
Fraud Detection and Prevention: The online gaming industry is not immune to fraud, with issues such as cheating, account hacking, and payment fraud posing significant challenges. Data science provides the tools needed to detect and prevent fraudulent activities, ensuring a fair and secure gaming environment.
Monetization Optimization: In-game purchases, subscriptions, and advertisements are common revenue streams in online gaming. Data science helps optimize these monetization strategies by analyzing player spending habits, identifying the most effective pricing models, and predicting future revenue trends.
The first step in utilizing data science in online gaming is data collection. Online games generate vast amounts of data, including:
Player Data: Information about player demographics, preferences, and behavior is collected through user profiles, in-game interactions, and social media integrations. This data helps developers understand their audience and tailor the gaming experience to different player segments.
Game Data: This includes data generated by the game itself, such as in-game events, level completion rates, and interaction with game mechanics. Analyzing this data provides insights into how players engage with the game and which features are most popular.
Monetization Data: Data on in-game purchases, subscriptions, and ad interactions is collected to understand player spending habits and optimize revenue streams. This data is crucial for developing effective monetization strategies and predicting future revenue.
Performance Data: Technical data on game performance, such as server load, latency, and error rates, is collected to ensure a smooth gaming experience. Analyzing this data helps developers identify and resolve performance issues, improving the overall quality of the game.
Data is collected through various methods, including in-game analytics tools, user surveys, and social media monitoring. The data is then stored in databases or data warehouses, where it can be accessed and analyzed by data scientists.
Once the data is collected, data scientists apply various analysis techniques to extract valuable insights. These techniques include:
Descriptive Analytics: Descriptive analytics involves summarizing and interpreting historical data to understand past player behavior and game performance. This technique is used to generate reports and dashboards that provide an overview of key metrics, such as player retention rates, average session duration, and in-game purchases.
Predictive Analytics: Predictive analytics uses statistical models and machine learning algorithms to predict future outcomes based on historical data. In online gaming, this technique is used to forecast player behavior, such as predicting which players are likely to churn or which in-game items will be popular.
Prescriptive Analytics: Prescriptive analytics involves recommending actions based on the insights gained from data analysis. For example, if predictive analytics indicates that a group of players is at risk of churning, prescriptive analytics can suggest targeted interventions, such as personalized offers or content updates, to retain those players.
Sentiment Analysis: Sentiment analysis uses natural language processing (NLP) techniques to analyze player feedback, reviews, and social media interactions. This analysis helps developers understand player sentiment and identify areas for improvement in the game.
Cluster Analysis: Cluster analysis groups players into segments based on their behavior and preferences. This technique is used to create personalized experiences and targeted marketing campaigns, ensuring that each player receives content that resonates with their interests.
Data science has numerous applications in the online gaming industry, impacting various aspects of game development, player engagement, and monetization. Some of the key applications include:
Data science plays a crucial role in the design and development of online games. By analyzing player data, developers can identify which game mechanics are most engaging and which features may need improvement. This data-driven approach ensures that the game is optimized for player enjoyment and long-term engagement.
For example, data science can help determine the optimal difficulty level for a game. By analyzing player progression data, developers can identify when players are most likely to quit due to frustration or boredom. Adjusting the game's difficulty based on this data can enhance the overall player experience and reduce churn.
Additionally, data science can be used to test new features and game mechanics before they are released. A/B testing, a common data science technique, involves comparing the performance of two different versions of a game feature to determine which one is more effective. This approach allows developers to make informed decisions about which features to include in the final game.
Retaining players is a major challenge in the highly competitive online gaming industry. Data science provides the tools needed to analyze player behavior and identify factors that contribute to player retention and engagement.
One common application of data science in this area is the analysis of player churn. By identifying patterns in player behavior that are associated with churn, developers can take proactive steps to retain players. For example, if data analysis reveals that players are more likely to churn after a certain level, developers can introduce new content, rewards, or challenges at that point to keep players engaged.
Data science is also used to personalize the gaming experience for each player. By analyzing player preferences and behavior, developers can create customized content, such as personalized recommendations, in-game events, and special offers. This personalized approach not only enhances player experience but also increases player retention and loyalty.
The in-game economy is a critical aspect of many online games, particularly those that rely on microtransactions, virtual currencies, and in-game purchases for revenue. Data science is used to analyze and optimize the in-game economy, ensuring that it is balanced and profitable.
One application of data science in this area is pricing optimization. By analyzing player spending data, developers can identify the most effective pricing strategies for in-game items, virtual currencies, and premium content. This analysis can also reveal which items are most popular and which ones may need to be adjusted to increase sales.
Data science is also used to analyze the effectiveness of monetization strategies, such as ad placements, subscription models, and in-game events. By tracking player interactions with these strategies, developers can identify which ones are most successful and make data-driven decisions to optimize revenue.
Fraud is a significant concern in the online gaming industry, with issues such as cheating, account hacking, and payment fraud posing risks to both players and game developers. Data science provides the tools needed to detect and prevent fraudulent activities, ensuring a fair and secure gaming environment.
Machine learning algorithms are commonly used in fraud detection, as they can analyze large datasets and identify patterns associated with fraudulent behavior. For example, data science can be used to detect unusual patterns of in-game behavior, such as players who achieve high levels of success in a short period of time, which may indicate cheating.
Data science is also used to monitor payment transactions and detect potential payment fraud. By analyzing transaction data, developers can identify suspicious patterns, such as multiple transactions from the same account or unusual spending behavior and take appropriate action to prevent fraud.
Predicting player behavior is one of the most powerful applications of data science in online gaming. By using predictive analytics, developers can anticipate how players will interact with the game, allowing them to make data-driven decisions that enhance the player experience.
One common application of player behavior prediction is in the design of in-game events and challenges. By analyzing player behavior data, developers can predict which events and challenges will be most popular and design them accordingly. This approach ensures that players remain engaged and motivated to continue playing.
Predictive analytics is also used to identify potential high-value players, known as "whales," who are likely to spend significant amounts of money on in-game purchases. By identifying these players early, developers can create targeted marketing campaigns and special offers to maximize their spending.
While data science offers numerous benefits to the online gaming industry, it also presents challenges and ethical considerations that must be addressed.
One of the primary challenges is data privacy. Online games collect vast amounts of personal data from players, including demographic information, gameplay behavior, and financial data. Ensuring that this data is collected, stored, and used responsibly is critical to maintaining player trust and complying with data protection regulations.
Another challenge is the potential for bias in data analysis. Machine learning algorithms are only as good as the data they are trained on. If the data used in training these algorithms is biased, the resulting predictions and recommendations can also be biased. In the context of online gaming, this could lead to unfair treatment of certain player groups or the reinforcement of harmful stereotypes. It is essential for data scientists and game developers to carefully consider the sources of their data and actively work to mitigate any potential biases.
Ethical considerations also come into play when using data science to influence player behavior. For instance, while predictive analytics can be used to identify players who are likely to make in-game purchases, it raises questions about the ethics of targeting these players with aggressive marketing tactics. There is a fine line between enhancing player experience and exploiting players for profit, particularly when it comes to vulnerable populations such as minors or individuals with gambling tendencies.
Additionally, the use of data science in game design can sometimes lead to unintended consequences, such as the creation of addictive gameplay loops that encourage excessive playtime. While this may increase engagement in the short term, it can have negative long-term effects on players' well-being. Game developers must balance the desire to create engaging content with the responsibility to promote healthy gaming habits.
As technology continues to evolve, the role of data science in online gaming is expected to grow even more significant. Several emerging trends and advancements are likely to shape the future of this field:
AI and Machine Learning Integration: The integration of artificial intelligence (AI) and machine learning (ML) into game development is already underway, and this trend is expected to accelerate. AI-driven game design, personalized AI-driven experiences, and more sophisticated predictive models will enhance the ways in which games are developed and experienced. For example, AI could be used to create dynamic, adaptive game worlds that respond to player behavior in real-time.
Real-time Data Processing: The ability to process and analyze data in real-time will become increasingly important, particularly in multiplayer and live-service games. Real-time analytics can provide immediate feedback to developers and enable rapid adjustments to gameplay, ensuring a smoother and more responsive player experience.
Cross-Platform Data Integration: As gaming becomes more cross-platform, with players engaging across multiple devices and platforms, the ability to integrate and analyze data from these various sources will be crucial. This will allow for a more holistic understanding of player behavior and enable the creation of seamless experiences across different platforms.
Enhanced Player Analytics: Advances in data analytics tools and techniques will provide deeper insights into player behavior. This could lead to more refined player segmentation, more accurate predictions of player preferences, and the ability to create even more personalized gaming experiences.
Ethical AI and Responsible Data Use: As the gaming industry continues to leverage data science, there will be increased focus on ethical AI and responsible data use. Industry standards and regulations will likely evolve to address concerns around data privacy, bias, and the ethical implications of data-driven game design.
Immersive Experiences: With the growth of virtual reality (VR) and augmented reality (AR) gaming, data science will play a crucial role in creating immersive experiences that are personalized and responsive to player actions. Analyzing data from VR/AR interactions will enable developers to refine these experiences and push the boundaries of what is possible in immersive gaming.
Data science has become an integral part of the online gaming industry, offering powerful tools for analyzing player behavior, optimizing game design, and enhancing the overall gaming experience. From predictive analytics and personalized content to fraud detection and monetization strategies, data science touches every aspect of the gaming lifecycle.
As the industry continues to grow and evolve, the importance of data science will only increase. However, with this power comes responsibility. Game developers and data scientists must navigate the challenges and ethical considerations associated with data-driven decision-making to ensure that they are creating games that are not only engaging and profitable but also fair and respectful of player rights.
The future of online gaming will be shaped by the continued integration of data science, AI, and real-time analytics, leading to more dynamic, personalized, and immersive gaming experiences. By harnessing the power of data science, the gaming industry can continue to innovate and provide players with experiences that are not only entertaining but also deeply engaging and fulfilling.