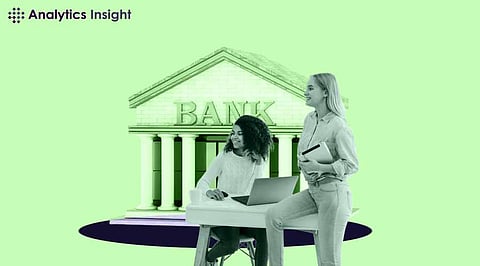
Data science has emerged as a game-changer in the banking industry, fundamentally transforming how financial institutions operate, make decisions, and engage with customers. By leveraging vast amounts of data, banks are now able to gain deeper insights, enhance operational efficiency, and offer more personalized services.
Here, this article delves into the transformation by data science in banking sector across various domains, from fraud detection to customer experience, and explores the future trends of data science in the banking sector:
Fraud detection and prevention are among the most critical applications of data science in banking. With millions of transactions processed daily, banks are prime targets for fraudulent activities. Traditional methods of fraud detection often fall short due to their reliance on predefined rules, which can be easily bypassed by sophisticated fraud schemes. Data science, however, offers a more robust solution.
By analyzing transaction patterns and customer behavior, data science enables banks to identify anomalies that may indicate fraudulent activities. Machine learning algorithms can process vast datasets in real-time, flagging suspicious transactions almost instantly. This capability significantly reduces the risk of fraud while also minimizing false positives, which can be costly and disruptive for both banks and customers.
For example, JPMorgan Chase implemented a machine learning model that reduced false positives by 50% in identifying potential fraudulent credit card transactions. This not only improved the accuracy of fraud detection but also saved the bank around US$200 million in fraudulent transaction losses. Such advancements highlight the transformative impact of data science in safeguarding financial institutions against fraud.
Credit risk assessment is another area where data science has made significant strides. Traditional credit scoring models typically rely on a limited set of data points, such as credit history, income, and employment status. While these factors are important, they provide an incomplete picture of an individual’s creditworthiness. Data science allows banks to incorporate a much broader range of data, leading to more accurate and comprehensive credit risk assessments.
For instance, data science can analyze non-traditional data sources such as social media activity, online behavior, and even utility bill payments. By integrating these additional data points, banks can better assess the credit risk of potential borrowers, reducing the likelihood of loan defaults.
FICO, a leading credit scoring company, exemplifies this approach by using advanced data analytics and machine learning algorithms to assess credit risk. Their models consider non-traditional data sources to improve the accuracy of risk assessments, enabling more informed lending decisions . As a result, banks can extend credit to a broader range of customers while managing risk more effectively.
The ability to segment customers based on their behavior, preferences, and financial needs is a powerful tool in the banking sector. Data science enables banks to create detailed customer profiles by analyzing transaction data, online interactions, and customer feedback. These profiles allow banks to tailor their products, services, and marketing efforts to meet the unique needs of each customer segment.
Wells Fargo, for example, uses data science to segment its customers based on spending patterns, demographics, and credit scores. This segmentation allows the bank to offer personalized products and services, enhancing customer satisfaction and loyalty. By providing targeted offers and recommendations, banks can improve customer engagement and drive higher conversion rates.
Moreover, personalization extends beyond product offerings. Data science can also enhance the overall customer experience by predicting customer needs and preferences. For instance, if a bank notices that a customer frequently travels internationally, it could proactively offer travel insurance or favorable currency exchange rates. Such personalized experiences not only meet customer expectations but also build long-term relationships.
Improving customer experience is a top priority for banks, and data science plays a crucial role in achieving this goal. By analyzing customer interactions across various channels, banks can identify pain points and areas for improvement. Predictive analytics can anticipate customer needs and preferences, enabling banks to offer proactive solutions that enhance the overall experience.
One notable example is the use of chatbots and virtual assistants powered by natural language processing (NLP). These AI-driven tools can handle routine inquiries, provide financial advice, and assist with transactions, offering customers a seamless and efficient banking experience. By automating these interactions, banks can provide 24/7 support, reduce wait times, and free up human agents to focus on more complex tasks.
Additionally, data science allows banks to personalize customer interactions across all touchpoints. Whether through personalized email campaigns, tailored product recommendations, or customized mobile app experiences, data-driven personalization helps banks deliver relevant content and services to their customers. This not only improves satisfaction but also fosters customer loyalty in an increasingly competitive market.
Data science also plays an important role in the enhancement of the functionality of the banks. In this way, the banks become aware of patterns of activities with regard to their work-flows that require optimization. For instance, predictive maintenance draws algorithms to recognize that a piece of equipment is likely to fail and subsequently plan for maintenance without causing disruption to the operations or making losses.
Moreover, advances in data science help banks, in turn, to improve the management of people resources. Reviewing the performance data of the employees, these banks are able to determine what training the employees require, where resources have to be invested and how productivity can be enhanced. This approach to the management of human capital allows for quality delivery of services and at the same time, the costs are effectively regulated.
Another of the operational competitiveness components is risk management. Data science gives the edge to banks by offering tools that enable them to understand, mitigate and control different types of risks such as- market risks, credit risks and operational risks. When it comes to application of predictive analytics in banking, it is about calculating the probable threats and coming up with ways of dealing with the threats.
For example, data science can improve the understanding of how changes in the economy affect portfolios and how, therefore, a bank should proceed in order to avoid significant losses. How risk management strengthens the position of banks when faced with volatility in the global market. These proactive measures of risk management helped in an improvement of the overall position of the various banks in the event that the market experienced volatilities.
One of the major constraints that face banks is the inability to deal with the regulatory environment since financial laws are complex and continually developing. In simplifying matters, data science helps in running compliance checks and guarantee all the transactions are in compliance with the laws. Machine learning algorithms can analyze vast amounts of data to detect any deviations from compliance norms, reducing the risk of penalties and legal issues.
In addition, by using data science, banks avoid having incorrect records, and they obtain fresh data making it easier to conduct audits and meet the necessary reporting requirements. Besides making compliance easier this automation also saves the compliance department’s time that can then be used on other crucial aspects. While regulatory standards will only continue to rise in the future, if your business is to survive, data science will have to step up to the task of keeping you in compliance.
The future of data science in the financial sector seems rather encouraging as several trends are expected to rise in the future. One of the trends that translate to the mixing of two or more fields is the use of blockchain in data science. While on one hand, blockchain offers a secure and integrated means of preserving the record of transactions, the corresponding execution of data science can identify these transactions and also use them to make better decisions. This hybrid approach holds the promise to reinvent domains that include fraud prevention, risk assessment, and managing compliance.
Another common trend is the upgrowth of the use of artificial intelligence and machine learning in the banking sector. All these technologies will remain as important technologies as they will give a higher level of tools in order to fight frauds, manage risks and serve customers. For instance, predictive analytics could help the banks to be more accurate in predicting the market and customer behaviors in future.
Additionally, the rise of open banking and the growing availability of big data will further expand the scope of data science in banking. As banks gain access to more diverse and granular data, they will be able to develop even more personalized and targeted offerings. This will not only enhance customer satisfaction but also create new revenue streams for banks.
Data science is clearly revolutionizing the banking industry, bringing a wide array of advantages such as better fraud prevention and risk oversight, improved customer satisfaction, and increased operational effectiveness. As financial institutions continue to adopt data science, they will be more prepared to tackle the intricacies of today's financial environment and provide outstanding value to their clients.
The effective use of data science in banking demands a precise equilibrium between innovation and risk control. Financial institutions need to allocate resources to the appropriate technologies, establish the essential data infrastructure, and foster a culture that values data to fully harness the power of data science. By doing this, they can maintain a competitive edge, adapt to changing customer needs, and achieve steady expansion in a world that is becoming more digital.
How does data science enhance fraud detection in banking?
Data science enhances fraud detection in banking by analyzing transaction patterns and customer behavior to identify anomalies that may indicate fraudulent activity. Machine learning algorithms process vast datasets in real-time, enabling banks to detect suspicious transactions quickly. This reduces both the risk of fraud and the number of false positives. By continuously learning from new data, these algorithms improve over time, providing a more robust and efficient fraud detection system.
How is data science used in credit risk assessment?
Data science improves credit risk assessment by incorporating a broader range of data points beyond traditional credit scores, such as social media activity, online behavior, and utility bill payments. By analyzing this diverse data, banks can make more accurate predictions about a borrower's ability to repay loans. This comprehensive approach reduces the likelihood of loan defaults and enables banks to extend credit to a wider range of customers, enhancing financial inclusion.
What role does data science play in customer personalization for banks?
Data science plays a crucial role in customer personalization by analyzing transaction data, online interactions, and customer feedback to create detailed profiles. Banks can segment customers based on behavior, preferences, and financial needs, allowing them to offer personalized products, services, and promotions.
How does data science improve operational efficiency in the banking sector?
Data science improves operational efficiency by analyzing internal processes, identifying bottlenecks, and optimizing workflows. Banks can use predictive maintenance to anticipate equipment failures, reducing downtime and operational costs. Additionally, data-driven workforce management allows banks to allocate resources more effectively and improve employee productivity.
What are the future trends of data science in the banking sector?
Future trends in data science for banking include the integration of blockchain technology, which provides a secure and transparent way to record transactions, and the continued evolution of artificial intelligence (AI) and machine learning. These technologies will offer more sophisticated tools for fraud detection, risk management, and customer service.