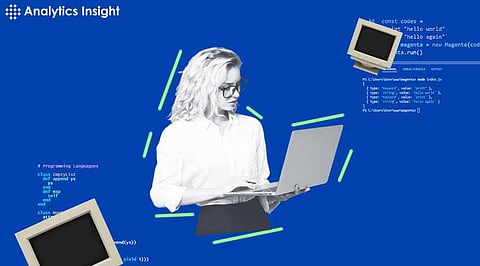
Data science is transforming industries by enabling companies to extract valuable insights from data. However, the challenges faced by startups and enterprises differ significantly due to disparities in resources, scale, and data complexity. Understanding these challenges is crucial for developing effective data science strategies tailored to different organizational structures. The following analysis highlights the key challenges faced by data science teams in startups compared to enterprises.
One of the primary challenges in data science is access to high-quality data. Startups often face limited access to data, which can impede the development of accurate models and insights. Startups typically lack the historical data required for building predictive models, which affects the performance of machine learning algorithms. In early stages, startups may rely on small datasets, leading to challenges in training robust models. A report by DataScience.com showed that 60% of startups struggle with data collection during their first two years.
Enterprises, on the other hand, have access to vast amounts of data accumulated over years of operation. However, managing this data poses its own challenges. The primary issue faced by enterprises is data quality. While there is plenty of data available, ensuring its accuracy, completeness, and relevance is difficult. According to Gartner, 47% of enterprise data is either inaccurate or incomplete, leading to flawed insights and wasted resources. Enterprises must invest heavily in data cleaning and governance to address these issues.
Budget constraints significantly impact data science efforts in startups. Most startups operate with limited financial resources, and data science often competes with other business functions for funding. Hiring a dedicated data science team can be costly, especially given the high demand for skilled professionals. Startups frequently rely on outsourcing or using affordable data science tools, which may limit the scope of analysis.
A study by DataRobot found that 72% of startups with fewer than 50 employees struggle to allocate sufficient budget for data science. This lack of funding impacts the ability to adopt advanced tools, access cloud services, or scale data operations.
In contrast, enterprises have larger budgets, allowing them to invest in the latest data science tools, cloud infrastructure, and top-tier talent. Enterprises can afford to build specialized teams, such as data engineers, data scientists, and machine learning engineers, to streamline data operations. Enterprises can also invest in scalable solutions, such as data lakes and cloud platforms, which offer greater flexibility for handling large datasets. However, managing these resources efficiently becomes a challenge, as larger teams and complex infrastructures require robust coordination and communication.
Both startups and enterprises face challenges in acquiring and retaining top data science talent. For startups, the competition for skilled data scientists is intense, as larger companies often offer higher salaries, benefits, and career growth opportunities. Startups may find it challenging to attract experienced professionals who seek job stability and competitive compensation.
Data science salaries in 2023 averaged around $120,000 annually, with enterprise-level companies offering even higher compensation. Startups may resort to hiring junior data scientists or outsourcing to freelancers, which can affect the quality and consistency of work. Retention is also an issue, as startup employees may leave for more lucrative offers at larger firms.
Enterprises, although better positioned to offer competitive salaries and benefits, face challenges in retaining data science talent due to a different set of issues. Data scientists in enterprises may face slower decision-making processes, bureaucratic hurdles, and rigid workflows that can stifle creativity and innovation. In large organizations, data scientists may become frustrated by the slower pace of project implementation, leading to dissatisfaction. A report from LinkedIn found that 42% of data scientists leave their jobs within two years due to lack of career growth and creative freedom.
Scalability presents a unique challenge for startups and enterprises, but in different ways. Startups often begin with small-scale data science projects, but as the business grows, data volumes increase exponentially. Startups must ensure that their data infrastructure and models can scale to meet increasing demands. Initially, startups may rely on lightweight data tools or local servers, but rapid growth necessitates transitioning to cloud platforms and scalable databases.
A survey by Clutch revealed that 68% of startups encounter scalability issues within their first three years of operation. Failure to scale data infrastructure effectively can lead to bottlenecks, slow data processing, and degraded model performance.
Enterprises, on the other hand, deal with the challenge of scaling data science initiatives across multiple departments and teams. Enterprises must ensure that data pipelines, storage solutions, and analytics platforms can handle large volumes of data in real time. A report by McKinsey highlighted that 55% of enterprises struggle to scale their data science models effectively due to legacy systems and siloed data architectures.
Scaling also involves integrating various data sources from different departments. Enterprises often struggle with data silos, where data is stored in isolated systems, making it difficult to unify and analyze data across the organization. To overcome these challenges, enterprises must invest in scalable cloud infrastructure, data integration tools, and cross-functional data teams.
In startups, decision-making tends to be more agile and dynamic. Startup founders and teams often rely on rapid iteration and experimentation, which allows data science teams to implement changes and test models quickly. However, the lack of structure in decision-making can sometimes lead to unplanned initiatives and misaligned priorities. Data science efforts may lack direction, especially when immediate business needs overshadow long-term data strategies.
According to research by the Harvard Business Review, 48% of startups report difficulty in aligning data science projects with business goals, often resulting in misused resources. Ensuring that data science initiatives align with business objectives from the outset is a key challenge for startup teams.
Enterprises face the opposite problem. Decision-making in large organizations tends to be slower due to bureaucratic layers and multiple stakeholders. Data science teams often face challenges in obtaining approvals for new projects, which delays implementation. A survey by Forrester found that 39% of enterprises cite slow decision-making as a key barrier to leveraging data science effectively.
Integrating data science insights into business operations can also be challenging in enterprises. Different departments may have varying priorities, making it difficult to establish a unified data strategy. Effective communication between data scientists and business leaders becomes essential to ensure data-driven insights inform critical business decisions.
Startups tend to adopt new technologies and frameworks more quickly due to their flexibility and lack of legacy systems. Startups are often early adopters of machine learning frameworks, cloud platforms, and automation tools. However, this eagerness can sometimes lead to inefficiencies if the chosen technology does not align with the startup’s long-term data strategy.
The ability to pivot quickly is beneficial, but without proper planning, technology changes can disrupt ongoing projects. A survey by TechCrunch reported that 32% of startups face challenges when transitioning to new technologies due to a lack of technical expertise or clear direction.
In contrast, enterprises often struggle with the slow adoption of new technologies due to existing legacy systems. Integrating modern AI and machine learning frameworks with legacy infrastructures can be complex, requiring significant investments in technology upgrades and staff training. A report by Deloitte found that 65% of enterprises are hindered by legacy systems that prevent them from adopting the latest data science tools and technologies.
Overcoming these challenges requires a structured approach to modernizing infrastructure and embracing innovative tools while minimizing disruptions to existing processes.
Startups and enterprises face unique challenges in the realm of data science. Startups often struggle with data availability, budget constraints, and scalability, while enterprises face challenges related to data quality, decision-making speed, and legacy systems. Addressing these challenges requires tailored strategies that align with each organization's specific needs, goals, and resources. By recognizing these hurdles, companies can leverage data science more effectively and unlock its full potential.