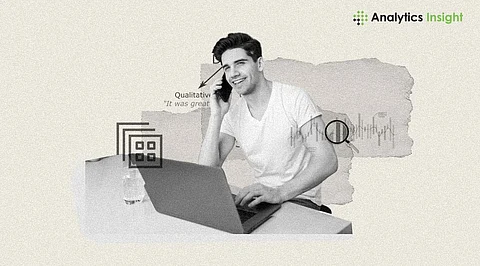
In the dynamic landscape of technology, Machine Learning (ML) has emerged as a transformative force, extending its capabilities beyond conventional data science applications to reshape the way businesses operate. As organizations increasingly recognize the potential of ML, adopting a thoughtful and strategic approach becomes paramount. In this article, we explore the crucial considerations that can unlock the full transformative power of ML in the business realm.
The journey into machine learning begins with setting the right targets for models. While accuracy is often a primary focus, businesses must think beyond this metric and align targets with broader organizational goals. For instance, in scenarios where certain misclassifications carry higher costs, optimizing for precision may be more relevant than aiming for overall accuracy. By tailoring ML targets to specific business needs, organizations can ensure that their machine learning endeavors align seamlessly with strategic objectives.
Imbalanced datasets, where one class significantly outweighs the others, pose a common challenge in ML. Ignoring this imbalance can result in biased models. To overcome this hurdle, businesses need to implement techniques such as oversampling the minority class or leveraging algorithms designed for imbalanced data. This approach ensures that the model is trained to recognize patterns in all classes, promoting more equitable and robust results.
The effectiveness of ML models lies in their ability to perform well in real-world scenarios. To achieve this, testing and validation processes should mirror the actual conditions the model will encounter. While conventional cross-validation methods are valuable, incorporating real-life testing is crucial. This approach validates the model with data resembling what it will face in production, ensuring adaptability to dynamic and practical business settings.
Selecting meaningful performance metrics is a critical aspect of evaluating ML models. Relying solely on accuracy may not provide a comprehensive picture, especially in situations with imbalanced classes. Metrics such as precision, recall, and F1 score offer a more nuanced assessment of a model's performance. By tailoring metrics to specific business objectives, organizations gain deeper insights into how well their ML models align with strategic goals.
ML models often generate scores or probabilities associated with predictions. While these scores can provide valuable insights, their interpretation requires careful consideration. The article emphasizes the importance of striking the right balance between the predictive power of the model and the practical utility of scores. In certain scenarios, the actual prediction might be more relevant than the associated score, emphasizing the need for a nuanced understanding of the business context.
Join our WhatsApp Channel to get the latest news, exclusives and videos on WhatsApp
_____________
Disclaimer: Analytics Insight does not provide financial advice or guidance. Also note that the cryptocurrencies mentioned/listed on the website could potentially be scams, i.e. designed to induce you to invest financial resources that may be lost forever and not be recoverable once investments are made. You are responsible for conducting your own research (DYOR) before making any investments. Read more here.