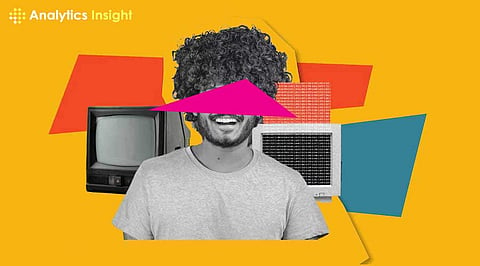
Data science is the art of mining expansive information sets of crude information, both organized and unstructured, to distinguish designs and extract significant knowledge from them. This is an intriguing field, and the establishments of data science incorporate measurements, induction, computer science, predictive analytics, machine learning algorithm development, and modern technologies to extract bits of knowledge from massive data.
To characterize data science and improve data science project administration, begin with its life cycle. The first organization in the data science pipeline workflow includes capture: securing information, in some cases extricating it, and entering it into the framework. The following arrangement is supported, which incorporates information warehousing, information cleansing, information preparation, data organizing, and data architecture.
Data processing is the second step and constitutes one of the data science basics. It is amid information investigation and handling that data researchers are separated from data engineers. This organization includes data mining, data classification and clustering, data modeling, and summarizing experiences gathered from the data—the forms that make viable data.
Next comes data analysis, which is a similarly essential organization. In this last arrangement, the data researcher communicates bits of knowledge. This includes data visualization, information reporting, the utilization of different commerce intelligence devices, and helping businesses, policymakers, and others make more brilliant choices. Let's have a deeper analysis of data science jobs and careers.
If you are planning to secure your life in data science jobs and careers, you can start by selecting one of the best options in this field.
Critical thinking is a vital aptitude that effortlessly applies to any calling. For data scientists, it's even more crucial since, in addition to finding experiences, you are required to be able to suitably outline questions and understand how those questions relate to the commerce or drive following steps that decipher into action.
You can't be a data scientist without the expertise or desire to illuminate issues. That's almost precisely what information science is all about. However, being a compelling issue solver is as much a craving to burrow to the root of an issue as it is knowing how to approach a problem to fathom it.
A data scientist must have intellectual interest and a drive to discover and reply to questions that the information presents, but moreover, respond to questions that were never inquired. Data science is about finding fundamental truths, and influential scientists will never settle for "just enough" but remain on the chase for answers.
Structured Query Language, or SQL, is the standard language for communicating with databases. Knowing SQL lets you upgrade, compose, and inquire about information stored in social databases and alter data structures (construction). Since nearly all information examiners will be required to utilize SQL to access data from a company's database, it's seemingly the most imperative expertise to learn to get a job. It's common for data analyst interviews to incorporate a specialized screening with SQL.
Statistical programming languages, like R or Python, empower you to perform progressed examinations. Data Analyst is one of the best data science careers in the present market. Composing programs in these languages implies you can clean, examine, and picture expansive data sets more productively. Machine learning, a department of artificial intelligence (AI), has become one of the most critical improvements in data science. This ability centers on building algorithms outlined to discover designs in enormous data sets, moving forward their precision over time.
As a data architect, you must understand your organization's trade objectives, challenges, and openings. You are required to communicate viably with business clients, examiners, and administrators and decipher their prerequisites into data solutions. Data modeling is the handle of making conceptual, consistent, and physical representations of data structures and connections. It is fundamental for data architects to ace information modeling strategies and devices, and apply them to distinctive sorts of data sources.
Data integration is the process of combining information from diverse sources and groups into a unique and coherent view. It is a key aptitude for data architects, as they are required to plan and execute information pipelines, workflows, and changes that empower information access, ingestion, handling, and conveyance. It is one of the best options for data science jobs and careers.
A foundational understanding of statistics is vital if you want to start your career as a machine learning designer. It permits you to translate data and extract significant experiences. This includes information on factual tests, dispersions, and probability hypotheses. You also must be well-versed in building exceedingly precise machine learning models, such as decision trees, clustering, and relapse algorithms.
After building a machine learning demonstration, you must assess its execution to ensure its viability and reliability. This includes utilizing suitable measurements like exactness and accuracy and reviewing them to evaluate whether the model is on standard with desires. To convey machine learning models and persistently screen their execution over time, you require learning DevOps, a combination of computer program improvement and IT operations.
Critical thinking empowers BI developers not only to report on information but also to scrutinize the legitimacy and unwavering quality of the data sources, address presumptions, and draw sound conclusions that bolster trade procedures. Capability in BI instruments such as Tableau, Power BI, or Qlik is essential for making dashboards and reports. Knowledge of SQL for questioning databases and understanding of programming languages like Python or R for information manipulation.
At the center of a DBA's role lies profound technical capability in database management. This expertise set incorporates skills in database planning, an understanding of structured query language (SQL), and recognition of database management systems (DBMS) such as Oracle, MySQL, Microsoft SQL Server, and newer NoSQL databases. Understanding the basic framework design is significant for DBAs. This includes information on equipment, storage frameworks, and networking as they relate to database execution and scalability.
With data security's expanding significance, DBAs must have a strong knowledge of information security standards. This incorporates actualizing get-to controls, encryption, and inspecting to defend sensitive data. Viable communication and collaboration are essential for DBAs, who frequently work with cross-functional groups.
Join our WhatsApp Channel to get the latest news, exclusives and videos on WhatsApp
_____________
Disclaimer: Analytics Insight does not provide financial advice or guidance. Also note that the cryptocurrencies mentioned/listed on the website could potentially be scams, i.e. designed to induce you to invest financial resources that may be lost forever and not be recoverable once investments are made. You are responsible for conducting your own research (DYOR) before making any investments. Read more here.