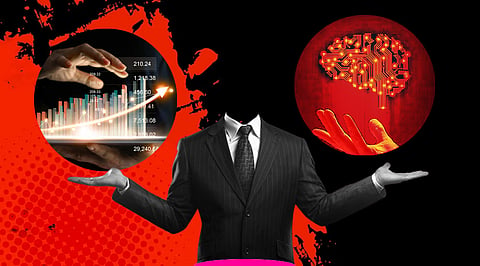
In today's rapidly changing technological landscape, businesses are constantly seeking ways to optimize their operations and stay ahead of the competition. One area that has become increasingly important is the use of data analysis to extract valuable insights and make informed decisions. However, there are multiple approaches to analyzing data, including machine learning and statistical data analytics. In this article, we will explore the key differences between these two methods and the benefits they offer. By understanding the unique features of each approach, businesses can make more informed decisions about which method is best suited for their specific needs and goals.
Machine Learning: Machine learning is a subset of artificial intelligence (AI) that involves building algorithms that can learn and make predictions or decisions based on data. These algorithms can be trained on large datasets to identify patterns and relationships in the data, and then use that knowledge to make predictions or take actions on new data.
Data Analytics: Data analytics involves using statistical and computational methods to extract insights from data. It includes descriptive analytics, which involves summarizing and visualizing data, and predictive analytics, which involves using statistical models to make predictions based on historical data.
Machine Learning: The purpose of machine learning is to build models that can make accurate predictions or decisions based on new data. It is commonly used in applications such as image recognition, speech recognition, and natural language processing.
To achieve its purpose, machine learning requires a significant amount of data and computational resources. Algorithms are trained on large datasets to identify patterns and make predictions based on new, previously unseen data. This process can be computationally intensive and requires expertise in areas such as statistics, mathematics, and computer science. However, the potential benefits of machine learning are significant, particularly in areas such as healthcare, finance, and transportation.
Data Analytics: The purpose of data analytics is to gain insights into the data that can be used to make better decisions. It is commonly used in applications such as customer segmentation, market analysis, and fraud detection.
It focuses on using statistical methods to analyze data and gain insights. This process involves identifying patterns, trends, and correlations within the data, as well as uncovering outliers and anomalies. Data analytics can be used to gain insights into customer behavior, market trends, and operational inefficiencies, among other things. While it does not typically require as many computational resources as machine learning, it does require expertise in areas such as statistics, data visualization, and business intelligence. The insights gained through data analytics can be used to drive decision-making and improve business performance
Machine Learning: Machine learning algorithms are typically trained on large datasets using a variety of techniques, such as supervised learning, unsupervised learning, and reinforcement learning. Supervised learning involves training an algorithm on labeled data, while unsupervised learning involves training an algorithm on unlabeled data. Reinforcement learning involves training an algorithm to make decisions based on feedback from its environment.
Data Analytics: Data analytics techniques include statistical analysis, data mining, and data visualization. Statistical analysis involves using statistical models to analyze data, while data mining involves discovering patterns and relationships in data. Data visualization involves presenting data in a graphical form to make it easier to understand.
Machine Learning: The output of machine learning algorithms is typically a prediction or decision based on new data. For example, a machine learning algorithm could be used to predict whether a customer is likely to churn or not, based on their previous behavior.
Data Analytics: The output of data analytics is typically insights and recommendations that can be used to make better decisions. For example, data analytics could be used to identify which products are selling well and which are not, and then make recommendations to improve sales.
Join our WhatsApp Channel to get the latest news, exclusives and videos on WhatsApp
_____________
Disclaimer: Analytics Insight does not provide financial advice or guidance. Also note that the cryptocurrencies mentioned/listed on the website could potentially be scams, i.e. designed to induce you to invest financial resources that may be lost forever and not be recoverable once investments are made. You are responsible for conducting your own research (DYOR) before making any investments. Read more here.