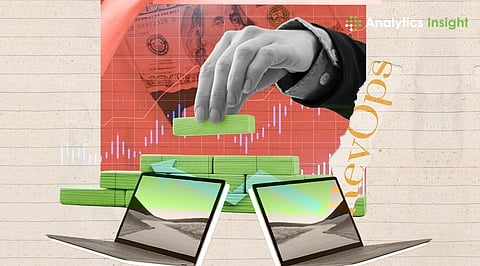
Exploratory Data Analysis (EDA) is a crucial step in the data analysis process, allowing analysts to understand the characteristics of a dataset and uncover insights that drive informed decision-making. To ensure a thorough and effective EDA process, it is essential to follow best practices that help maximize the value of the analysis. In this guide, we will explore the 10 best practices for exploratory data analysis(EDA), providing you with actionable tips and strategies to enhance your data exploration journey. Whether you are a seasoned data scientist or just starting, these best practices will help you make the most out of your data and derive meaningful insights that drive business outcomes.
Before embarking on any analysis, it is crucial to familiarize yourself with the dataset. Start by examining its structure, including the number of observations and variables. Identify the data types of each variable (e.g., numerical, categorical) and understand their meanings. Look at summary statistics to get a sense of the data's central tendency, dispersion, and shape.
Visualization is a powerful tool for gaining insights into the distribution and patterns present in the data. Create visualizations such as histograms, scatter plots, box plots, and density plots to explore the data's characteristics. Histograms can help you understand the distribution of numerical variables, while scatter plots can reveal relationships between variables.
Missing data is a common issue in datasets and can significantly impact the results of an analysis. It is essential to identify and understand the nature of missing values in your dataset. Decide on an appropriate strategy for handling missing data, such as imputation (replacing missing values with estimated values) or removal (excluding observations with missing values). Whatever approach you choose, ensure transparency in your methods to maintain the reproducibility of your analysis.
Data points known as outliers can cause statistical studies to be distorted because they differ noticeably from the rest of the data. Use visualizations like box plots or scatter plots to identify outliers in your dataset. Consider the context of your analysis and the nature of the data when deciding whether to keep or remove outliers. In some cases, outliers may represent valid observations and should be retained; in others, they may indicate errors and should be removed.
EDA is not just about exploring individual variables but also about understanding the relationships between variables. Use tools like correlation matrices, scatter plots, and heat maps to visualize relationships between variables. Look for trends, dependencies, and potential confounding factors that may influence your analysis. Understanding these relationships is crucial for making informed decisions and deriving meaningful insights from your data.
Data segmentation involves dividing your dataset into meaningful categories or segments to analyze patterns and trends more effectively. By segmenting data based on relevant criteria such as demographics, geography, or behavior, you can gain deeper insights and tailor your analysis to specific groups.
Descriptive statistics, such as mean, median, standard deviation, and quartiles, provide a summary of your data's central tendency and dispersion. These statistics help you understand the distribution of your data and identify outliers or patterns that may require further investigation.
Analyzing time trends is crucial if your data has a temporal component. Time series analysis can reveal patterns, seasonality, and trends over time. Visualizing data using line charts or seasonal decomposition plots can help you understand how variables change over different periods.
Multicollinearity occurs when independent variables in a regression model are highly correlated, leading to unstable estimates. To assess multicollinearity, calculate correlation coefficients between predictors and consider using variance inflation factors (VIFs) to identify problematic variables.
Documenting your exploratory data analysis (EDA) process is essential for reproducibility and collaboration. Keep a record of the steps you take, the insights you uncover, and any decisions you make during the analysis. This documentation ensures that others can understand and reproduce your analysis, leading to more reliable results.
In conclusion, effective EDA is essential for understanding a dataset deeply and making informed decisions in data analysis. By following these best practices, analysts can uncover hidden patterns, relationships, and insights that drive meaningful conclusions and inform future actions.
Join our WhatsApp Channel to get the latest news, exclusives and videos on WhatsApp
_____________
Disclaimer: Analytics Insight does not provide financial advice or guidance. Also note that the cryptocurrencies mentioned/listed on the website could potentially be scams, i.e. designed to induce you to invest financial resources that may be lost forever and not be recoverable once investments are made. You are responsible for conducting your own research (DYOR) before making any investments. Read more here.