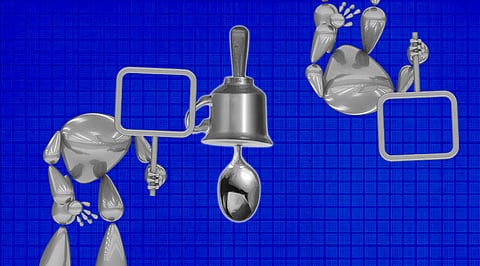
For AI to be called sentient it has to think like an average human being. Humans have evolved into thinking beings by taking inputs from their surroundings and putting them into action in a cyclic fashion. The logical connections they make are hardwired in their heads by virtue of repeated exposure to their environment. If we apply similar logic to AI image generators which are trained over billions of images, they should be able to generate compositionally correct images. A recent research paper, "Testing Relational Understanding in Text-Guided Image Generation", released by Harvard University has tested Dall-E 2's compositional capability using a set of 15 basic physical and social relations studied or proposed in the literature. Given the fact that Dall-E 2 has been trained over 12 billion parameters, the results arrived at should be a surprise.
For one particular prompt, 'Spoon in the cup' it got totally wrong with weird and out-of-world compositions while it could get images of children with bowl images correct in a context for which it could have seen thousands of images. For an unlikely event like 'Monkey touching an Iguana', DALL-E 2 couldn't bring it to perfection. What is the big deal if DALL E2 couldn't perform for imagined scenarios? The problem lies in its inability to relate two objects in their contextual sense. For example, the images posted on DALL-E 2 site, for a monkey astronaut and an otter with a pearl ear-ring clearly demonstrate that DALL-E 2 cannot differentiate representations of images in semantics and style. The research paper states, "DALL-E 2's difficulty with even basic spatial relations (such as in, on, under) suggests that whatever it has learned, it has not yet learned the kinds of representations that allow humans to so flexibly and robustly structure the world".
The neural network, CLIP, developed by OpenAI for text-based image-generating applications, holds the key to understanding the loophole. The network, by default, can take instructions in natural language to perform a variety of classification benchmarks, without directly optimizing for the benchmark's performance, which makes it more representative, bypassing the task of taking into consideration the labeled objects. Moreover, CLIP's functionality lies in embedding images and text in the same latent space – which allows text-based image generation in the first place.
To overcome this problem, the authors suggest, like in the robotics model, it needs to blend with the environment it wants to portray so as to avoid patchwork. CLIPort, which is similar to CLIP in model building, can identify the relations between different elements and also manipulate them in terms of abstract concepts. In fact, the problem is not restricted to DALL-E 2. The rival artificial intelligence image generator, Imagen of Google, has a compiled list of metrics under its Drawbench worksheet for comparison and gauging against the pre-defined parameters.
The authors of the paper also suggest pitting human estimation to judge the accuracy of generated images rather than depending on algorithmic metrics. For a typical prompt like 'T-rex chasing a man', DALL E-2 should be able to generate images worth having goosebumps. But there is a high probability that the T-rex would look dumb and harmless, at least in one of the images – a scenario only a human with a mind can identify as an error.
Join our WhatsApp Channel to get the latest news, exclusives and videos on WhatsApp
_____________
Disclaimer: Analytics Insight does not provide financial advice or guidance. Also note that the cryptocurrencies mentioned/listed on the website could potentially be scams, i.e. designed to induce you to invest financial resources that may be lost forever and not be recoverable once investments are made. You are responsible for conducting your own research (DYOR) before making any investments. Read more here.