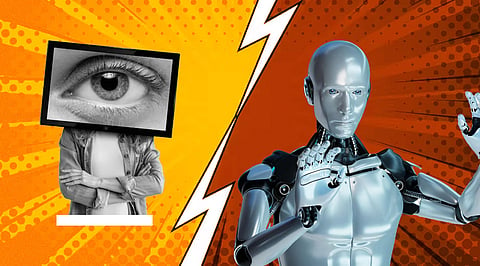
Computer vision is a branch of artificial intelligence (AI) that enables computers and systems to extract useful information from digital photos, videos, and other visual inputs and to execute actions or make recommendations based on that information. If AI gives computers the ability to think, computer vision gives them the ability to see, observe, and comprehend. Human vision has an advantage over computer vision in that it has been around longer. With a lifetime of context, human sight has the advantage of learning how to distinguish between things, determine their distance from the viewer, determine whether they are moving, and determine whether an image is correct. Using cameras, data, and algorithms instead of retinas, optic nerves, and the visual cortex, computer vision teaches computers to execute similar tasks in much less time. A system trained to inspect items or monitor a production asset can swiftly outperform humans since it can examine thousands of products or processes per minute while spotting imperceptible flaws or problems. Energy, utilities, manufacturing, and the automobile industries all use computer vision, and the market is still expanding.
A lot of data is required for computer vision. It repeatedly executes analyses of the data until it can distinguish between things and recognize images. For instance, a computer needs to be fed a huge amount of tire photos and tire-related things to be trained to detect automotive tires. This is especially true of tires without any flaws. This is done using two key technologies: convolutional neural networks and deep learning, a sort of machine learning (CNN). With the use of algorithmic models, a computer can learn how to understand the context of visual input using machine learning. The computer will "look" at the data and educate itself to distinguish between different images if enough data is sent through the model. Instead of needing to be programmed to recognize an image, algorithms allow the machine to learn on its own.
By dissecting images into pixels with labels or tags, a CNN aids a machine learning or deep learning model's ability to "see." It creates predictions about what it is "seeing" by performing convolutions on the labels, which is a mathematical operation on two functions to create a third function. Until the predictions start to come true, the neural network conducts convolutions and evaluates the accuracy of its predictions repeatedly. Then, it is recognizing or views images similarly to how people do. Similar to how a human would perceive a picture from a distance, a CNN first recognizes sharp contours and basic forms before adding details as it iteratively tests its predictions. To comprehend individual images, a CNN is utilized. Like this, recurrent neural networks (RNNs) are employed in video applications to assist computers in comprehending the relationships between the images in a sequence of frames. Here are some applications of computer vision:
A dog, an apple, or a person's face are examples of images that can be classified using image classification. More specifically, it can correctly guess which class a given image belongs to. A social network corporation would want to utilize it, for instance, to automatically recognize and sort out offensive photographs shared by users.
To identify a specific class of image and then recognize and tabulate its existence in an image or video, object detection can employ image classification. Detecting damage on an assembly line or locating equipment that needs maintenance are a couple of examples.
After an object is found, it is followed or tracked. This operation is frequently carried out using real-time video streams or a series of sequentially taken pictures. For instance, autonomous vehicles must track moving things like pedestrians, other vehicles, and road infrastructure in addition to classifying and detecting them to avoid crashes and follow traffic regulations.
Instead of focusing on the metadata tags that are attached to the photos, content-based image retrieval employs computer vision to browse, search, and retrieve images from massive data repositories. Automatic picture annotation can be used in place of manual image tagging for this activity. These tasks can be used in digital asset management systems to improve search and retrieval precision.
Join our WhatsApp Channel to get the latest news, exclusives and videos on WhatsApp
_____________
Disclaimer: Analytics Insight does not provide financial advice or guidance. Also note that the cryptocurrencies mentioned/listed on the website could potentially be scams, i.e. designed to induce you to invest financial resources that may be lost forever and not be recoverable once investments are made. You are responsible for conducting your own research (DYOR) before making any investments. Read more here.