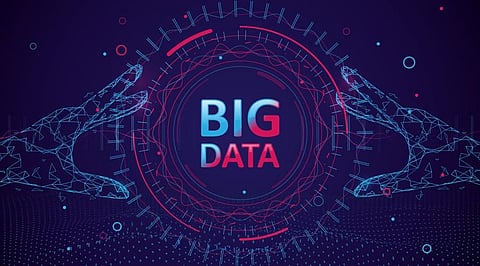
Each business is presently a data business. Data is a key vital resource today whether you're an enormous global conglomerate or a locally owned, family-run business. If you don't have a successful data strategy—or one by any means—your organization is passing up the immense potential business value data offers.
With the assistance of the most recent technologies, organizations of all sizes approach rich, granular information about their operations and customers, which is a key vital resource for them. However, understanding how to manage the gigantic volumes of data can be troublesome and expensive for the organizations and even with the correct tools available to them, it very well may be overpowering.
There are high odds of organizations committing errors with their datasets with such a lot of complexity around big data. Here are common mistakes organizations make while using big data
The world is changing rapidly and a business strategy that hasn't evolved to be applicable for the fourth Industrial Revolution won't be compelling. Your data strategy should uphold a business system that is right and applicable to the present world. It looks bad to invest energy and assets on gathering and analysing data on the side of an obsolete business strategy. Not exclusively will you not get to where you should be, yet you will waste time and resources attempting to get there.
From the start, it could be enticing to bounce directly in and gather data on an entire host of points without appropriate idea into how this will help your business. Raw information frequently says nothing at all to most business users, and making huge databases with overflowing amounts of information offers no specific benefit or helpful purpose, except from taking your time and assets.
A word of wisdom to assist business with staying away from this trap is to make a big data roadmap. This should zero in on critical business decisions, short and long-term, that will assist everybody with understanding what data can bring value to their business.
For effectively dealing with the significant business resource of customer data, organizations need technology to simplify data collection, auto-scale as information volumes fluctuate and empower core operations, including Artificial Intelligence, all while taking into account customization. A typical mistake which organizations make is to search for short term ROI from these advances instead of focussing on their long-term value and advantages they present to the business.
The next most significant angle is guaranteeing that you have excellent data. You may have immense amounts of data that is from the correct source and aligned with your goal; in any case, this doesn't subvert the requirement for that data to be precise and predictable. Enormous organizations really employ people just to clean up huge amounts of data to guarantee consistency and uniformity.
At the beginning of any data project, proper data governance should be set up. Contemplations for how to utilize data morally as well as the legal and privacy issues of data use should be defined. Customer trust is of core importance. Customers should feel certain that you will utilize their information securely and that they will pick up some tangible value for allowing you to utilize their information. The significance of deciding information access rights, security, morals, privacy and how to ensure data quality cannot be underestimated.
Subsequent to gathering data effectively, numerous organizations think that it's difficult to get value and insight from the data basically on the grounds that they haven't put enough resources in setting up a dedicated BI group which will help them gather, analyse and share data in ways to drive progress.
Join our WhatsApp Channel to get the latest news, exclusives and videos on WhatsApp
_____________
Disclaimer: Analytics Insight does not provide financial advice or guidance. Also note that the cryptocurrencies mentioned/listed on the website could potentially be scams, i.e. designed to induce you to invest financial resources that may be lost forever and not be recoverable once investments are made. You are responsible for conducting your own research (DYOR) before making any investments. Read more here.