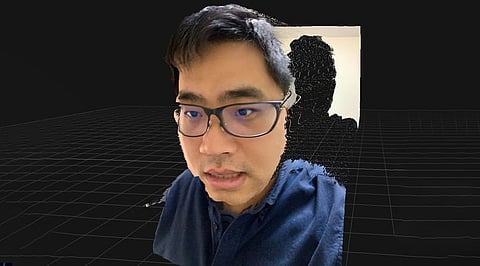
Last December, Google Photos had introduced new features for its app, including improvements to the 'Memories' feature that surfaces images from the past depending on the location and date of the images. One of the most intriguing features is Cinematic Photos.
This feature uses deep learning to predict an image's depth and produce a 3D representation of the scene. It then animates the picture to produce a panning effect. Thus, making the images look more vivid and realistic.
According to a Google blog, this feature even works for original images which don't include depth information from the camera – gathered by portrait modes. Generally, only few cameras have depth sensors, but various Google products — including ARCore and the Google Pixel's Camera app for Portrait Mode — can calculate it from a flat input. Generally, smartphone depend on multi-view stereo, a geometry method to solve for the depth of objects in a scene by simultaneously capturing multiple photos at different viewpoints, where the distances between the cameras are known. The Google Pixel phone creates views come from two cameras or dual-pixel sensors. Besides, Cinematic Photo feature only requires the relative depths of objects in the scene for producing 3D representations, not the absolute depths.
For the photos that lack depth information or the ones that were not taken in multi-view stereo, the Google team trained a convolutional neural network with encoder-decoder architecture to predict a depth map from just a single RGB image. A convolutional neural network is a form of deep learning that can take in an input image, assign importance (learnable weights and biases) to various aspects/objects in the image and be able to differentiate one from the other. Using only one view, the model learned to estimate depth using monocular cues, such as the relative sizes of objects, linear perspective, defocus blur, and more.
Since, datasets for monocular depth estimation are usually designed for areas like AR, robotics, and self-driving, they tend to emphasize street scenes or indoor room scenes instead of elements that are more common in casual photography. These can be people, pets, and objects. So, to offset this, Google developed its own dataset for training the monocular depth model using photos captured on a custom 5-camera rig as well as another dataset of Portrait photos captured on Pixel 4. Both datasets included ground-truth depth from multi-view stereo that is critical for training a model.
Another concern was sensitivity to the depth map's accuracy at person boundaries. This is crucial since any error in depth map can end up producing bizarre final image. Therefore, the Google team used a DeepLab segmentation model for median filtering to improve the edges, and also infer segmentation masks of any people in the photo. The DeepLab segmentation model was trained on the Open Images dataset.
The next task was to build 3D representation of the Google Photos images, via meshing and masking. Normally, 3D rendered objects can have multiple degrees of freedom – which is why Cinematic photos feature must be capable of identifying the optimal pivot point for the virtual camera's rotation to yield the best results by drawing one's eye to the subject.
The first step in 3D scene reconstruction is to create a mesh by extruding the RGB image onto the depth map. This will enable neighboring points (or nodes) in mesh to have large depth differences – which can make the rendered output look 'stretched'. This means that now, Cinematic photos must find a trajectory that introduces parallax and at the same time reduce these 'stretchy' elements.
As a result, it uses a loss function that captures how much of the stretchiness can be seen in the final animation. This allows optimization of the camera parameters for each unique photo. The Google team utilizes padded segmentation masks from a human pose network to divide the image into three different regions: head, body and background. Then the loss function is normalized inside each region before computing the final loss as a weighted sum of the normalized losses.
Last part of Cinematic photos feature involves reframing the final output video /image into a rectangle with portrait orientation. To achieve this, it is crucial to frame the output with the correct right aspect ratio while still retaining the key parts of the input image. So, the Google team uses a deep neural network that predicts per-pixel saliency of the full image. When framing the virtual camera in 3D, the model identifies and captures as many salient regions as possible while ensuring that the rendered mesh fully occupies every output video frame.
Since Cinematic photos provide a smooth zooming effect that's certainly eye-catching, it could work nicely for some well-framed people shots. Users can turn it on by tapping on their account profile photo and then going to Google Photos settings > Memories > Advanced > toggle on/off Cinematic photos. The key idea behind this innovation is to offer the viewer a feeling of being taken back to the moment that was captured in the photograph—to enhance emotions.
Google Photos was launched back in 2015. Though it has split from the now-defunct social media site Google+, Google Photos has gone on to become one of the largest and most recognizable cloud-based photo-sharing services in the world. Memories launched in September 2019 to encourage users to revisit moments from the past one, two, three, four, five, etc. years.
To read more, visit here.
Join our WhatsApp Channel to get the latest news, exclusives and videos on WhatsApp
_____________
Disclaimer: Analytics Insight does not provide financial advice or guidance. Also note that the cryptocurrencies mentioned/listed on the website could potentially be scams, i.e. designed to induce you to invest financial resources that may be lost forever and not be recoverable once investments are made. You are responsible for conducting your own research (DYOR) before making any investments. Read more here.