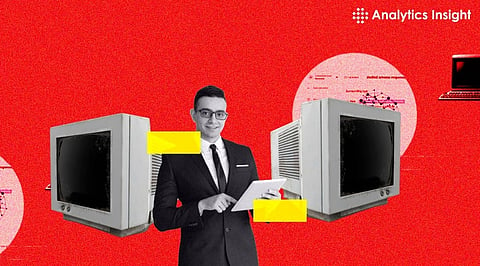
Transitioning from a Data Engineer to an Analytics Engineer can be a strategic career move for professionals seeking to align their technical skills with business acumen. This article explores the pathway from data engineering to analytics engineering, highlighting the skills required, the benefits of making the switch, and practical steps to facilitate the transition.
Data engineering and analytics engineering are closely related fields within the broader spectrum of data science. While data engineers focus on the infrastructure and architecture needed to process and store data efficiently, analytics engineers specialize in extracting insights and creating actionable intelligence from that data. The transition between these roles involves a shift in focus from building and maintaining data pipelines to utilizing data to drive strategic decision-making and problem-solving.
One of the key advantages for data engineers transitioning to analytics engineering is their strong technical foundation. Data engineers are well-versed in programming languages such as Python, Scala, and Java as well as technologies like Spark, Hadoop, and SQL databases. These skills form the backbone of analytics engineering, enabling professionals to manipulate, analyze, and visualize data effectively.
However, transitioning to analytics engineer role requires a deeper understanding of statistical methods, machine learning algorithms, and data visualization techniques. Data engineers must augment their existing skill set by familiarizing themselves with statistical concepts such as hypothesis testing, regression analysis, and clustering. Moreover, proficiency in data visualization tools like Tableau, Power BI, or Matplotlib is crucial for presenting insights clearly and compellingly.
To facilitate a smooth transition, data engineers can take advantage of various resources and learning opportunities. Online platforms such as Coursera, Udacity, and edX offer specialized courses and certifications in data analytics, machine learning, and visualization. Additionally, participating in workshops, attending industry conferences, and joining professional networking groups can provide valuable insights and connections within the analytics community.
Mentorship programs are another valuable resource for aspiring analytics engineers. Engaging with experienced professionals in the field can offer guidance, support, and practical advice for navigating the transition process. Mentors can provide valuable feedback on projects, recommend relevant resources, and help individuals identify areas for skill development.
One of the defining characteristics of analytics engineering is its emphasis on problem-solving and critical thinking. Analytics engineers are tasked with uncovering actionable insights from complex datasets to inform business decisions and drive innovation. As such, transitioning professionals must cultivate a curious mindset and a passion for tackling challenging problems.
To excel in an analytics engineering role, individuals should hone their ability to ask the right questions, dissect data effectively, and derive meaningful conclusions. This requires a blend of analytical rigor, creativity, and business acumen. By approaching problems with a strategic mindset and leveraging their technical expertise, data engineers can make a seamless transition to the world of analytics.
While the transition from data engineering to analytics engineering involves acquiring new skills and knowledge, many core competencies are transferable between the two roles. For example, strong communication skills are essential for collaborating with cross-functional teams, presenting findings to stakeholders, and translating technical jargon into actionable insights.
Moreover, project management skills acquired as a data engineer, such as task prioritization, resource allocation, and deadline management, remain valuable in an analytics engineering context. By leveraging these transferable skills, transitioning professionals can position themselves as valuable assets within their organizations and accelerate their career growth in the field of data science.
The career transition from data engineer to analytics engineer offers a promising pathway for professionals seeking to expand their horizons and delve deeper into the world of data analysis and interpretation. By building on their technical foundations, embracing a problem-solving mindset, and leveraging transferable skills, data engineers can make a seamless transition to the field of analytics engineering.
As organizations increasingly rely on data-driven insights to inform strategic decisions, the need for skilled analytics professionals rises. By investing in continuous learning, staying abreast of industry trends, and embracing new challenges, transitioning professionals can position themselves for success in this dynamic and rewarding field.
Join our WhatsApp Channel to get the latest news, exclusives and videos on WhatsApp
_____________
Disclaimer: Analytics Insight does not provide financial advice or guidance. Also note that the cryptocurrencies mentioned/listed on the website could potentially be scams, i.e. designed to induce you to invest financial resources that may be lost forever and not be recoverable once investments are made. You are responsible for conducting your own research (DYOR) before making any investments. Read more here.