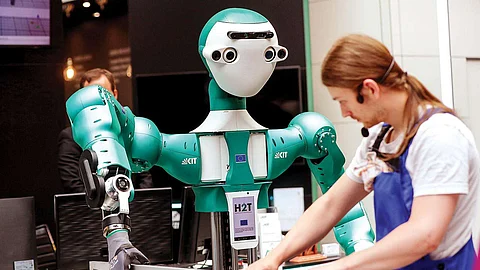
Researchers at the University of Southern California (USC) have developed a system that can perform complicated tasks with only a few demonstrations. The research paper titled, "Learning from Demonstration Using Signal Temporal Logic", was presented at the Conference of Robot Learning last week.
The research paper cites that the system addresses the existing limitations of reinforcement learning in robots such as imperfections, safety, and interpretability. Researchers have used Signal Temporal logic-so that robot can learn tasks such as driving a car and cooking the food from only a few demonstrations.
Aniruddh Puranic, a PhD student in computer science at the USC Viterbi School of Engineering and the lead author of the research says, "Many machine learning and reinforcement learning systems require large amounts of data and hundreds of demonstrations—you need a human to demonstrate over and over again, which is not feasible,"
The existing learning-from-demonstration-model in robots involves reinforcement learning. Over the years existing LfD has enabled researchers to draw insights on specifications of the robot. However, there are certain limitations to this model:
Introduced by Arthur Prior under the name Tense Logic in 1960, the Signal Temporal Logic is a mathematical language that enables robotic reasoning about current and future outcomes.
Researchers have earlier used STL in cyber-physical system applications such as robots and self-driving cars, to evaluate the logic and reasoning during an emergency. The existing STL system delivers positive outcomes for evaluating the temporal behaviour in robots such as the multi-dimensional signal consisting of the robot's position, joint angles, angular velocities, and linear velocity, amongst others.
It also evaluates and automatically rank demonstrations based on their fitness of the specific task performed, and enhance the control policy in robots. This system also estimates the quality of demonstrations so that robots cannot learn from undesired examples.
The researchers states that "The key insight of this work is that the use of even partial STL specifications can help in a mechanism to automatically evaluate and rank demonstrations, leading to learning robust control policies and inferring rewards to be used in a model-free RL setting."
Unlike the traditional model, this system enables the robot to learn from both its success and failure, with only a few demonstrations. Through this system, the robots can learn and perform the task without requiring logic and can draw out its conclusion about the performance.
The researchers conclude that this approach will provide new directions for safety and interpretability of robot control policies and verification of model-free learning methods. It is also well suited for applications where the maps are known beforehand but there exist dynamic obstacles in the map, such as for robots in household and warehouse environments, space exploration rovers, etc.
Join our WhatsApp Channel to get the latest news, exclusives and videos on WhatsApp
_____________
Disclaimer: Analytics Insight does not provide financial advice or guidance. Also note that the cryptocurrencies mentioned/listed on the website could potentially be scams, i.e. designed to induce you to invest financial resources that may be lost forever and not be recoverable once investments are made. You are responsible for conducting your own research (DYOR) before making any investments. Read more here.