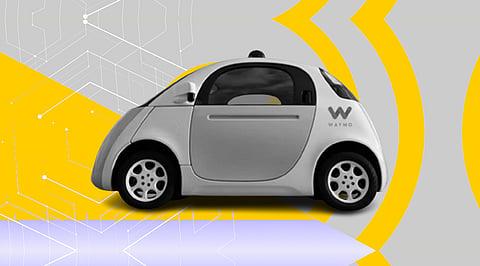
Some of the most exciting companies in the autonomous industry have traveled thousands of kilometers to delicate their AI-based technology. It costs time and money, and the bulk of test drive locations have been in warm places with dry roads, and are hardly average driving conditions. If self-driving vehicles follow this mile-by-mile strategy, they are unlikely to become popular anytime soon. Another technique to train and tweak AI for optimal self-driving is to use cutting-edge simulators that increase deep learning and allow AI to be taught in a range of driving circumstances, allowing autonomous vehicles to reach the market faster (AVs). The latest self-driving technologies recognize that using a simulation testing environment may save development expenses dramatically. Surprisingly, the aviation business, with its high level of automation and excellent safety requirements, acts as a role model for the car industry in this area. However, due to sheer numbers, it is difficult to educate automobile drivers like pilots. The easiest approach to replicate aviation's low accident percentages, according to smart autonomous engineers, is to replace drivers with computers. To make those algorithms as clever as possible, the AI must be exposed to as many traffic scenarios as feasible.
In a gaming engine-based virtual reality environment, scenes may be replayed an unlimited number of times, which would either never happen or pose an intolerable risk for human drivers. Unlike pilot training for airplanes, information obtained while driving through various traffic conditions may be readily transferred to an endless number of automobiles via Over-the-Air (OTA) updates, allowing a single simulator to service all AVs. Data collected by simulating varied weather, lighting, and road surface conditions opens up a much wider variety of options than any other real-world driving experiment. Take Google's Waymo self-driving vehicle project, for example. The experiment did not meet any strange events despite traveling over 2 million miles and encountering a few minor traffic accidents.
To educate AI on how to behave in a variety of traffic circumstances, billions of miles of driving would be required in a range of locales and at various times of the year. It would also include navigating risky events, accidents, and barriers that may result in serious damage, and it would have to be done numerous times to guarantee that the technology has been properly taught. To put it another way, bringing autonomous technology to the public is nearly difficult and probably a zero-sum game.
Overall, it's safe to assume that the deep learning utilized in autonomous driving will continue to lag behind the professional knowledge of well-trained pilots for a long time. However, AI-based systems can drive automobiles as safely as the most experienced driver, avoiding the majority of human-error accidents that occur today. It's worth noting that, while simulation promises to be more cost-effective, quicker, and safer than real-world testing, the latter will not go away completely. That's a positive development. Only a small number of real-world tests are required to precisely complement AI simulation, allowing for speedier attainment of complete autonomy.
When serial entrepreneur Barry Lunn wanted to launch a driving software company, he visited several industry insider gatherings, where he claims he discovered "the reality" of the hyped-up autonomous-driving scenario. He began attending sensor and autonomous trade fairs in 2015, traveling from Detroit to Silicon Valley, where he rubbed elbows and had cocktails with prominent artificial intelligence figures, he claims. Lunn had a formula for getting information during conferences: establish a reputation as the go-to after-party guy, invite who's who out with you, and pick their brains about issues they wouldn't talk about in public.
The AI simulator technology resembles an automobile game in appearance and feel. The only difference is that instead of a gamer putting their abilities to the test, each new simulation trains a machine to navigate and respond. Artificial intelligence learns via neural networks in the same manner the human brain does. It's not just a matter of programming rules or concepts into the computer's memory; rather, after being exposed to a variety of traffic events and experiences, the machine may eventually "learn" how to drive.
Researchers from Carnegie Mellon University drove an all-terrain vehicle on a wild journey through tall grass, loose gravel, and mud to see how the vehicle interacted with a difficult off-road environment. They accelerated the extensively instrumented ATV to speeds of up to 30 miles per hour. They slid it around corners, up and down hills, and even got it stuck in the mud, all while collecting data from seven different sensors, including video, wheel speed, and suspension shock travel.
The resulting dataset, TartanDrive, has about 200,000 of these real-world contacts. According to the researchers, the dataset is the world's biggest real-world, multimodal, off-road driving dataset in terms of both interactions and sensor types. Just five hours of data is enough to educate a self-driving car on how to deviate from the way less travelled. "Off-road driving is more challenging than autonomous street driving because you need to understand the dynamics of the terrain to drive safely and quickly," Wenshan Wang, a project scientist at the Robotics Institute (RI), said.
Join our WhatsApp Channel to get the latest news, exclusives and videos on WhatsApp
_____________
Disclaimer: Analytics Insight does not provide financial advice or guidance. Also note that the cryptocurrencies mentioned/listed on the website could potentially be scams, i.e. designed to induce you to invest financial resources that may be lost forever and not be recoverable once investments are made. You are responsible for conducting your own research (DYOR) before making any investments. Read more here.