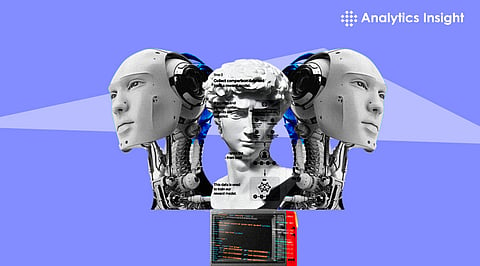
With the emergence of Artificial Intelligence (AI) and Machine Learning (ML) in almost every segment including healthcare, education, etc. new possibilities have opened up.
However, AI and ML are still falling short in every segment, especially in the business segment. According to research 80% of CIOs are assigned to research and evaluate analytics of AI, among them 74% are working closely with their business leaders to deliver inputs, yet only 32% of them successfully implement over 60% of ML models.
Companies or businesses shouldn’t expect the best results initially as models require a lot of experimentation and iteration to establish accurate models, robust dashboards, and AI-driven operations that boost productivity. This article explores the actual reasons why AI and ML are falling short.
There are several reasons why they are falling short. In this article, we will explore the reasons behind the falling back of AI and ML.
Data Quality Issues – AI and ML models require high-quality, labeled data to function efficiently. In many cases, such data is not available, out of budget, or it contains biases that can degrade results.
Generalization – AI models don’t have that much capability to generalize new and unseen data quickly. They perform fluently on the training data but fail when they have to go through situations or processes that differ from the training set.
Ethical and Privacy Concerns – Questions are raised when it comes to privacy, as AI and ML models are trained on personal data that can reveal sensitive data unknowingly.
Lack of Explanation and Transparency – AI and ML models often function as “black boxes”, which make situations critical to reaching specific decisions. This lack of transparency can create issues, especially in the finance and healthcare sectors.
Computational and Resource Intensity – It requires certain computational power and energy to train and deploy AI and ML models including the larger ones, which can be expensive and unsafe for the environment.
Interaction between AI and Humans- There remains a gap in human-AI collaboration, as users may not trust AI when it comes to resolving severe issues, including if they don’t know how AI or ML works besides, making mistakes.
Bias and Fairness – Models can inherit biases from the training data, leading to unfair or discriminatory outcomes. This is a notable issue, especially in sensitive situations such as hiring, lending, and law enforcement.
Regulation and Standards – The lack of clear rules and standards comprehensively for AI, can lead to inconsistent practices and misuse.
Here are some of the solutions that can be brought into practice to make sure the growth of AI and ML in business sectors is not terribly slow.
Data Quality Issues – It's advisable to invest in good data collection and cleaning processes.
Generalization – Implement techniques such as cross-validation and standardization to improve model generalization. Update models with new data tailored to changing conditions.
Ethical and Privacy Concerns – Develop ethical guidelines for AI and ML platforms. Ensure secured privacy and potential usage of technology.
Lack of Explanation and Transparency – Establish models that are easier to access and interpret in difficult situations. Deploy tools and techniques that make AI decision-making processes clearer and more concise to users.
Computational and Resource Intensity – Keep a check upon algorithms for efficiency and less requirement of computational and energy. Discover alternative methods that support less power and resources.
Interaction between AI and Humans – Create user-friendly interfaces that can give clearer explanations of the functions to build trust and initiate better collaboration between humans and machines.
Bias and Fairness – Apply techniques to detect and reduce bias in data and algorithms, besides audit models every day to ensure fairness and provide diverse perspectives in development.
Regulation and Standards – Advocate for comprehensive regulations and industry standards for AI and ML systems.
Even with the upgrade, AI and ML fall short in business sectors. There are several reasons why users aren’t utilizing them appropriately. They require lots of experimentation and iteration to adapt to the changing conditions of real-world scenarios. Initiatives of easy-going usage of technology should be overseen, besides checking upon its correct implementation ethically.
A: AI and ML face numerous challenges such as data quality issues, bias and fairness, difficulty in interaction with users, lack of explanation and transparency, ethical and privacy concerns, computational and resource intensiveness, and lack of regulations and standards.
A: AI and ML require a vast number of datasets to learn efficiently and generalize with the new data aligned with the outside world. Insufficient or poor-quality data may lead to inadequate results.
A: The “Black Box” issue refers to the lack of transparency in AI models especially when it comes to deep learning and decision-making. This can cause issues in sensitive fields such as healthcare and finance.
A: Ethical concerns include their over and incorrect usage, whereas privacy concerns involve revealing of sensitive information when trained on personal data.
A: Training and implementing large AI and ML demands significant computational power and energy, which can be expensive and unfriendly to the environment.
A: There’s a need for comprehensive regulations and standards as without clear regulations, and standards, there’s a risk of inconsistent usage. Regulations and standards ensure the fair and responsible use of AL and ML systems.