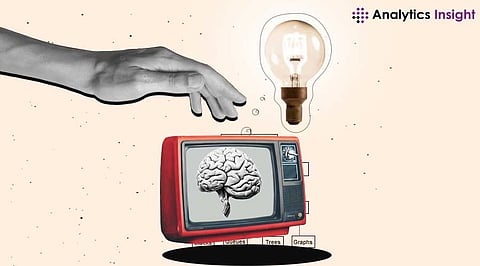
Generative AI technologies have, in recent times, had their pace of evolution representative of a huge step in the history of human technological advances. They have found wide applications and significant attention. And also, capital inflows in either artistic representation, text writing, scientific discovery, or the capacity to create individualized user experiences. Most such projects will fail by the year 2025, though. The article discusses why it is a foresight that about 30% of Gen-AI projects will be abandoned, discussing issues across technology, market dynamics, ethics, and others.
Why 30% of Gen-AI Projects Will Be Abandoned by 2025
Generative AI projects are highly technologically complex. Such technologies, born in technological complexity, deal with heavy, complex algorithms, large data processing, and high computational resources. While recent generative models like GPT-4 and DALL-E have been great, the underlying technology, by its very nature, is extremely complicated and forever blossoming.
Generative AI models are changing the landscape of technology every day. But their development is, at the best of times, an expensive affair. Much has to go into their initial budgets in terms of data acquisition, huge computational power, and talent. For the case of startups and new entrants into the Gen-AI space, the pace might be kept up in terms of investment once projects are rolled out. Most of the time, this results in strapped financial abilities to keep a project running for the much-needed time.
Computational resources are enormous, and computation time is considerably large to train generative AI models for peak performance. Again, high complexity fine-tunes these models for use-case requirements. Models are continuously being adjusted. Potential unpredictability in model performance can get too overwhelming for the project teams, with possible abandonment if results do not play out as expected.
The challenge of scaling such prototypes to production environments lies in making their generative AI solutions multidimensional. The actual question would be whether the models are broad enough. And reasonably scalable to perform according to requirements at scale without any hardware issues. There are other problems such as latency management, working in large-scale systems, and resource management. Such natural issues often arise to raise critical scalability problems that can be the cause for delay and, in general, things that may result in project failure if the solution cannot be properly scaled.
The generative market is pretty much competitive and dynamic, with many players wanting to dominate it. With the fast rate of technological innovation and change in market demand, it leaves single projects at a higher risk.
The more widespread adoption of generative AI technologies leads to saturation within the market. With an ever-increasing flow of new entrants in the market with similar or even better technologies, a high rate of competition is potentially inflicted. What this may mean is potential market fragmentation, in which every individual project has to struggle a great deal to stand out from the crowd or find a proper base for sustainable competitive advantage.
The habits and expectations of consumers change at an extreme rate depending on the market trends and variations in technological advancement. Relevance is lost if the projects are adapted not to these changes in consumer demand or can't provide a new value proposition. In case AI generative projects aren't copacetic with these shifts in the brand, some of them will go into the shelf in preference of a new fitting solution.
Economic conditions drive market dynamics. In case of an economic downturn or uncertainty related to the economy, investment in emergent technologies will be reduced. Companies that feel financial pressure will focus on short-term aims and likely cancel experimental or high-risk projects, killing several generative AI projects.
Generative AI ethical considerations and challenges are not different from the ones that plague any new AI development. Generative AI acts within a complex, wide, and multifaceted ethical and regulatory environment. Within the nature of its sophistication lies a myriad of ethical and legal considerations at play, guiding and influencing its development and implementation.
The major ethical issues are the ones associated with authenticity, bias, and misuse of generative AI technologies. They are capable of making machine-generated content appear human. For example, deepfakes and manipulated media create huge possibilities for privacy risks and misinformation because they are the product of AI. It requires care and a degree of proactiveness in engaging with these kinds of issues that may be difficult to sustain for most project teams. That load may propel the abandonment of innovation projects unable to make the trade-off between innovation and ethics.
In itself, the regulatory environment for generative AI is at a very rudimentary stage. Governments and all levels of regulatory agencies are presently drawing outlines and frameworks to capture the uniqueness of these innovations. In light of this context, the inability to clear and consistent pre-existing legislation would cause uncertainty in the enforcement of legislation by parties. High regulatory dynamics could burden some projects to the extent that they stop their projects instead, for fear of noncompliance.
Most generative AI projects have an extended requirement for data to train the model to fit. There is mandatory compliance with Data protection regulations, especially GDPR and CCPA, yet it can be elusive. Responsible data management with responsive reactions to the users' data whenever their privacy is concerned. Poor strategies and resources increase legal and reputational risks, which might cause project abandonment.
While all generative AI demonstrates some extent of promise, not every use case is created equal, some are more viable or useful than others. A successful generative AI project will fulfill actual needs or bring concrete value.
Some generative AI projects may fail to provide a clear value proposition. If the purpose is not clearly defined, or the benefits are not demonstrated, little is then known to users and partners about what the value proposition is. In such a project, interest will wane with no compelling use cases coming or measurable results present. This kind of project risks abandonment.
It may prove to be an overwhelming task for underappreciated legacy systems and workflows to which the solution of generative AI needs accommodating. In this respect, elusiveness will characterize the diffusion for initiatives that do not consider the integration problem or those that do not have seamless solutions at hand. The biggest reason that generative AI initiatives are bound to fail in a lack of diffusion is a lack of integration into the existing infrastructure.
The determinant of success or failure for any generative AI project generally rests on user acceptance and trust. For example, if the AI-generated solution is considered intrusive, opaque, and unreliable by users, then the rate of acceptance will be impaired. Hence, taking into consideration that this project will ensure the establishment of trust by the user, and displaying the reliability of the AI-generated output will be very important. Projects that are unable to win the confidence of users can't go ahead for long and are finally grounded.
Success in generative AI is heavily dependent on the quality of the teams behind these projects. Team dynamics and the required skill development add yet another layer of importance for successful project delivery.
Generative AI creating new job opportunities is a fact. However, such a niche application is generative AI that requires specialized knowledge from areas like machine learning and data science. Often, demand outstrips supply, and a resultant talent crunch comes to the fore. If there is an inability of certain projects to attract or retain sought-after talent. It can result in development issues and delays in the project, which may ultimately result in its abandonment.
The success of a project team is premised on collaboration and communication. Projects operating in the wake of generative AI are affected by technical misalignment efforts. In the event of disagreements, miscommunication, or no cohesion whatsoever, progress is at a snail's pace, and abandonment rates are high.
The dynamism might just be too high, with pressure to work on the bleeding edge, so that it will simply burn people out. Very long working hours linked to high expectations and strong pressure to get things done reduce team morale and productivity. These challenges could be further enhanced by resource constraints in either funding or computational resources. Burnout or resource constraint problems make a project too hard to handle and, in most cases, get abandoned.
Every generative AI project has to be aligned to broader business strategies and goals. If the objectives of the projects mismatch the actions on strategic organizational priorities. This may hit the result of the project.
There are innumerable instances when the strategic priorities of the organizations have to be reassessed again and again concerning the emerging trends in the market, pressures of the competition, and other internal factors. Those initiatives of the generative AI that do not coincide with the newly emerging priorities for the business are at risk of losing sponsorship or funding. Such projects which are not coherent with the core organizational strategy can be canceled and potentially deprioritized.
Every generative AI project needs to have clear, achievable objectives. If the targets are not well-defined or success metrics are missing, the result could be the establishment of a project that cannot clearly show progress or value added. Measurable outcomes can quickly lead to disillusionment if the road mapping is not clear, with abandonment imminent.
Success means doing what the investors, customers, or partners expect of you. The generative AI projects must, therefore, come up to the expectations of the stakeholders by rendering the expected outcome as promised by the model. When that doesn't happen, support and funding decrease, after which most projects are normally abandoned.
There are still many companies in the Generative AI landscape but the future of the field doesn’t look so bright. By 2025, only 30% of generative AI projects will be dropped amidst an agglomeration of technical complexity, market dynamics, ethical challenges, shallow utility, team or talent issues, and strategic misalignment. In other words, success in projects can help cut through various challenges in the rapidly evolving landscape of generative AI. While this abandonment rate might be a worrying statistic, it also reflects the dynamic and rapid change potential in the region. Only those projects that can effectively help solve these challenges, adapt to changing circumstances, and generate some concrete value within an increasingly competitive environment will tend to succeed.