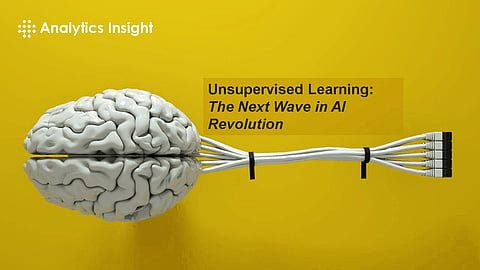
Throughout the last decade, machine learning has gained exceptional ground in areas as varied as image recognition, self-driving vehicles and playing complex games like Go. These victories have been generally acknowledged via preparing deep neural systems with one of two learning paradigms which are supervised learning and reinforcement learning. The two standards require training signals to be structured by a human and then passed to the computer. On account of supervised learning, these are the "objectives, (for example, the right name for a picture); on account of reinforcement learning, they are the "rewards" for fruitful conduct, (for example, getting a high score in an Atari game). The cutoff points of learning are in this way characterized by human mentors.
A key inspiration for unsupervised learning is that, while the information given to learning algorithms is incredibly wealthy in inner structure (e.g., pictures, videos and content), the objectives and prizes utilized for training are ordinarily extremely scanty (e.g., the name 'dog' alluding to that especially protean species, or a solitary one or zero to indicate achievement or failure in a game). This recommends the bulk of what is found out by an algorithm must comprise of understanding the information itself, instead of applying that understanding to specific tasks.
According to Yann LeCun, the chief AI scientist at Facebook and a professor at NYU, an infant can build up a comprehension of an elephant after seeing two photographs, while deep learning algorithms need to see thousands, if not millions. A teenager can figure out how to drive safely by rehearsing for 20 hours and figure out how to stay away from accidents without first encountering one, while reinforcement learning algorithms (a subcategory of deep learning) must experience a huge number of trials, including numerous deplorable failures.
The appropriate response, he supposes, is in the underrated deep learning subcategory known as unsupervised learning. While algorithms dependent on supervised and reinforcement learning are instructed to accomplish a target through human info, unsupervised ones identify patterns in data totally all alone. LeCun leans towards the expression "self-supervised learning" since it basically uses some portion of the training information to foresee the remainder of the training data.
As of late, such algorithms have increased noteworthy footing in natural language processing on account of their capacity to discover the connections between billions of words. This demonstrates valuable for building text prediction systems like autocomplete or for creating convincing prose. Yet, by far most of AI research into different areas have concentrated on supervised or reinforcement learning.
Maybe the easiest target for unsupervised learning is to train an algorithm to create its very own instances of information. Alleged generative models should not just recreate the information they are trained on (an uninteresting demonstration of memorisation), yet rather assemble a model of the fundamental class from which that information was drawn: not a specific photo of a pony or a rainbow, however, the set of all photos of horses and rainbows; not a particular expression from a particular speaker, yet the general circulation of spoken articulations. The core principle of generative models is that having the option to develop a persuading model regarding the data is the most grounded proof of having understood it: as Richard Feynman put it, "what I can't create, I don't understand."
For pictures, the best generative model so far has been the Generative Adversarial Network (GAN for short), in which two networks, generator and a discriminator—take part in a challenge of discernment akin to that of an aesthetic counterfeiter and a detective. The generator produces pictures with the objective of fooling the discriminator into believing they are genuine; the discriminator, in the interim, is compensated for recognizing the fakes. The produced pictures, first messy and irregular, are refined over numerous cycles, and the progressing dynamic between the networks leads to perpetually realistic pictures that are much of the time indistinct from genuine photos. Generative adversarial networks can likewise dream details of scenarios characterized by the rough sketches of users.
LeCun opines that the emphasis should be flipped. He further adds that all that we learn as humans, everything, is learned through self-regulated learning. There's a thin layer we learn through supervised learning, and a little sum we learn through reinforcement learning. If AI, or AI, is a cake, by far most of the cake is self-supervised learning.
Researchers should start by concentrating on worldly prediction. At the end of the day, train huge neural networks to foresee the second 50% of a video when given the first. While not everything in our reality can be anticipated, this is the basic aptitude behind an infant's capacity to understand that a toy truck should fall. He believes that this is somewhat of a simulation of what's happening in your mind if you need.
When the field creates strategies that refine those capacities, they will have significant practical uses too. It's a smart thought to do video prediction with regards to self-driving vehicles since one should need to know ahead of time what different cars in the city are going to do. At last, unsupervised learning will help machines build up a model of the world that would then be able to foresee future conditions of the world. It's a grandiose desire that has evaded AI research yet would open up a completely new host of abilities.
Join our WhatsApp Channel to get the latest news, exclusives and videos on WhatsApp
_____________
Disclaimer: Analytics Insight does not provide financial advice or guidance. Also note that the cryptocurrencies mentioned/listed on the website could potentially be scams, i.e. designed to induce you to invest financial resources that may be lost forever and not be recoverable once investments are made. You are responsible for conducting your own research (DYOR) before making any investments. Read more here.