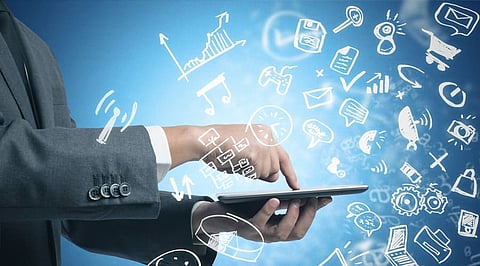
Today, artificial intelligence is already making its way into every business function, transforming how the work is done. More and more businesses understand the capabilities of AI and machine learning. And to harness its power, they are intensifying their AI investments for business growth. It delivers a variety of opportunities to businesses to deliver innovative, unique and effective solutions to their customers. But many businesses leverage AI and machine learning face accuracy failure. They get narrowly focused on model accuracy while wasting valuable resources in over-optimization. They have placed good solutions but don't get great accuracies that are huge lost opportunities for them.
Despite AI and ML models could be inaccurate, they can still transform the business for the better. According to Ryan Moore, Associate Director of Marketing Sciences at Astellas Pharma, many factors influence model accuracy, especially these four:
a) Are we including all relevant inputs that can influence outcomes?
b) What is the quality of historical data we have collected?
c) Does our model capture the true relationships between the inputs and outcome?
d) How different will future scenarios be from what was used to train the model?
By optimizing these factors, the data science team can strive for accuracy improvement.
Artificial intelligence has the potential to support imperative business needs. These include automation of business processes, gaining insight through data analysis, engaging with customers and employees, etc.
Machine learning can deliver numerous benefits to enterprises. These include the ability to find out patterns and correlations, enhance customer segmentation and targeting, and eventually bolster businesses' revenue, growth and market position. Many organizations utilize AI/ML in customer relationship management, underwriting, fraud detection, automating processes and even social media monitoring to gauge brand perception.
Once businesses have a machine learning solution, how can they evaluate its business impact? Dan Ariely, the author of Predictably Irrational, says "Most people don't know what they want unless they see it in context." They can't make sense of a number in isolation. This is the challenge with model accuracies. Thus, to contextualize model accuracy, Ganesh Kesari, a Data Science leader notes, comparing it with human error rate for the same task within an organization.
AI is often seen as humans' competitor and a better replacement in some context. Most organizations believe an AI-powered approach can help them drive innovation and put them forward against their peers. But bringing AI and humans together can facilitate greater productivity and gains, augmenting each other's capabilities. As per Pew Research, 72% of employees fear AI stealing their jobs. This technology can boost business productivity by up to 40%, Accenture noted. However, it doesn't mean the adoption of AI is a wipeout of work available to humans. As some tasks can be done by AI, like the algorithms that drive recommendation engines on platforms like Amazon, Google, Netflix, etc., others are reserved for human skill only. Thus, keeping humans in the loop when designing machine learning systems can derive great accuracy and improve outcomes.
Moreover, businesses must check if the model has scope for continuous improvement as well as its real purpose when gauging ML systems' business impact.
Join our WhatsApp Channel to get the latest news, exclusives and videos on WhatsApp
_____________
Disclaimer: Analytics Insight does not provide financial advice or guidance. Also note that the cryptocurrencies mentioned/listed on the website could potentially be scams, i.e. designed to induce you to invest financial resources that may be lost forever and not be recoverable once investments are made. You are responsible for conducting your own research (DYOR) before making any investments. Read more here.