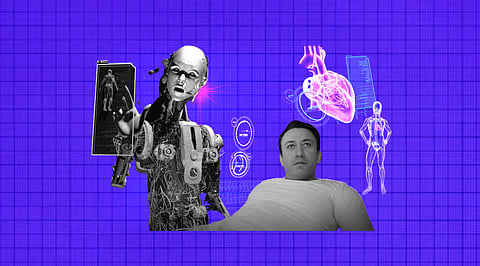
Research has led to improvements in machine learning algorithms that can help clinicians access information in a patient's health record more rapidly.
Medical specialists regularly refer to electronic health records for information that helps them decide on treatments, but the cumbersome nature of these documents makes this process challenging. According to research, even when a doctor has been taught to utilize an electronic health record (EHR), it can still take more than eight minutes to locate the answer to just one query.
Doctors have less time to engage with patients and administer care the longer they must spend navigating a frequently cumbersome EHR interface.
Researchers are creating ML algorithms to expedite the procedure by finding the data that clinicians need in an EHR automatically. Large datasets with relevant medical questions must be used to train effective models, however, these may be hard to get by due to privacy regulations. Current models frequently produce inaccurate results and struggle to think of legitimate inquiries that a human doctor would make.
MIT researchers and medical experts worked together to examine the questions doctors ask while reviewing electronic health records to fill up this data gap (EHRs). Then, they developed a dataset with over 2,000 clinically relevant questions developed by these medical experts and made it available to the public.
They used their dataset to train an ML model to generate clinical questions, and they found that the program asked high-quality and genuine questions over 60% of the time when compared to actual questions from specialists.
They plan to create a significant number of actual medical queries from this dataset, which will be used to develop an ML model that will help doctors identify relevant information in patient records more rapidly.
Lehman explains that there were several problems with the few sizable datasets of medical questions the researchers were able to locate. Some of these included patient-posted medical questions from online message boards, which varied greatly from inquiries for physicians. Most of those queries in other datasets are implausible since they were created using templates, which were used for other datasets.
To create their dataset, the MIT researchers worked with practicing doctors and senior medical students. These medical experts received more than 100 EHR release statements, and they were told to review each one and ask any questions they might have. The researchers did not put any restrictions on question formats or patterns to acquire genuine questions.
They discovered that the majority of questions were centered on the patient's symptoms, medications, or test findings. Even if these results weren't a surprise, measuring how many questions there were on each major subject will enable them to create a useful dataset that can be applied in a real-world clinical context.
They trained ML models that would create new questions depending on the trigger text using their dataset of test queries and linked trigger text.
When a model was provided trigger language, the researchers discovered that it could come up with a decent question 63 percent of the time, while a human doctor would ask a fair question 80 percent of the time.
Using the publicly accessible datasets they had discovered at the beginning of this study, they also trained algorithms to recover answers to clinical inquiries. These trained models were then put to the test to evaluate how well they could react to "great" questions from actual medical practitioners.
Only around 25% of the answers to the questions that the specialists came up with could be recovered by the algorithms.
To fulfill their original goal of creating a model that can automatically answer physicians' inquiries in an EHR, the group is now setting this work to use.
The following phase will involve using their dataset to teach an ML model that can automatically produce tens of thousands or millions of high-quality clinical questions. This model could then be used to design a new model for answering questions.
Join our WhatsApp Channel to get the latest news, exclusives and videos on WhatsApp
_____________
Disclaimer: Analytics Insight does not provide financial advice or guidance. Also note that the cryptocurrencies mentioned/listed on the website could potentially be scams, i.e. designed to induce you to invest financial resources that may be lost forever and not be recoverable once investments are made. You are responsible for conducting your own research (DYOR) before making any investments. Read more here.