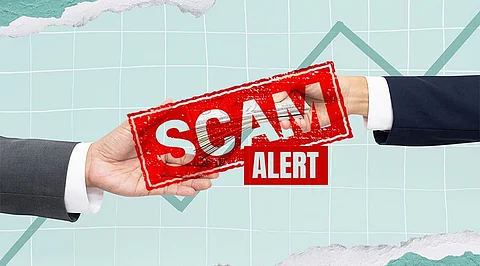
Machine learning refers to analytic techniques that "learn" patterns in datasets without being guided by a human analyst. Artificial intelligence refers to the broader application of specific kinds of analytics to accomplish tasks, from driving a car to, yes, identifying a fraudulent transaction. For our purposes, think of machine learning as a way to build analytic models and AI as the use of those models.
Machine learning helps data scientists efficiently determine which transactions are most likely to be fraudulent, while significantly reducing false positives. The techniques are extremely effective in fraud prevention and detection, as they allow for the automated discovery of patterns across large volumes of streaming transactions.
If done properly, machine learning can clearly distinguish legitimate and fraudulent behaviors while adapting over time to new, previously unseen fraud tactics. This can become quite complex as there is a need to interpret patterns in the data and apply data science to continually improve the ability to distinguish normal behavior from abnormal behavior. This requires thousands of computations to be accurately performed in milliseconds.
Because organized crime schemes are so sophisticated and quick to adapt, defense strategies based on any single, one-size-fits-all analytic technique will produce sub-par results. Each use case should be supported by expertly crafted anomaly detection techniques that are optimal for the problem at hand. As a result, both supervised and unsupervised models play important roles in fraud detection and must be woven into comprehensive, next-generation fraud strategies.
Given the sophistication and speed of organized fraud rings, behavioral profiles must be updated with each transaction. This is a key component of helping financial institutions anticipate individual behaviors and execute fraud detection strategies, at scale, which distinguish both legitimate and illicit behavior changes.
In order to maintain a positive consumer experience, specialized fraud analytics must be used to assess the "tough" questions. This is where advanced profiling, fraud-specific predictive characteristics, and adaptive capabilities separate themselves from generic behavior analytics. In a world of real-time payment processing and rapidly changing consumer preferences, generic behavior models are not sufficient for cross-channel, enterprise fraud solutions. After all, when and how someone chooses to transact is not as predictable as his or her likelihood to cancel a fitness club membership.
Join our WhatsApp Channel to get the latest news, exclusives and videos on WhatsApp
_____________
Disclaimer: Analytics Insight does not provide financial advice or guidance. Also note that the cryptocurrencies mentioned/listed on the website could potentially be scams, i.e. designed to induce you to invest financial resources that may be lost forever and not be recoverable once investments are made. You are responsible for conducting your own research (DYOR) before making any investments. Read more here.