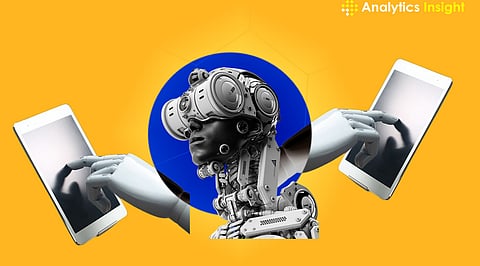
Edge AI has emerged as a revolutionary approach to enhancing the performance and capabilities of artificial intelligence (AI) applications. By processing data locally on devices rather than relying solely on cloud computing, Edge AI brings several benefits including reduced latency, enhanced privacy, and improved efficiency. This article explores the top 10 platforms for developing Edge AI applications, providing insights into their features, advantages, and how they contribute to the growing ecosystem of AI applications.
Edge AI refers to the deployment of AI algorithms on local devices such as smartphones, sensors, and other IoT (Internet of Things) devices. Unlike traditional AI systems that depend heavily on cloud-based processing, Edge AI performs computations at the edge of the network. This approach not only speeds up data processing but also enhances data security and reduces the reliance on internet connectivity. The development of Edge AI applications is crucial for industries where real-time decision-making and data privacy are paramount.
NVIDIA Jetson is a powerful platform for Edge AI applications, offering a range of hardware and software solutions tailored for AI and deep learning tasks. It provides high-performance computing capabilities in a compact form factor, making it ideal for robotics, smart cities, and healthcare applications. The Jetson family includes various modules like Jetson Nano, Jetson Xavier NX, and Jetson AGX Xavier, catering to different performance and power requirements.
Google Coral is already intended to extend the AI processing to the edge devices. To this end, it provides system on modules such as the Coral Dev Board and a USB Accelerator, in addition to the Edge TPU which is a Tensor Processing Unit for AI inference. Google Coral works with TensorFlow Lite which enables developers to deploy the models easier. The mentioned platform is suitable, for example, in the environmental monitoring system, security or retailed-based analysis.
AWS IoT Greengrass is a technology that extends the possibility for AWS cloud services to edge devices, which is giving a possibility for them to act locally on the data they generate. It has support for machine learning inference, data processing, and secure communication between devices. Greengrass eliminate, the rigidity in using AWS services and the flexibility in deploying and managing Edge AI applications.
Microsoft Azure IoT Edge is a fully managed service that provides cloud intelligence locally by deploying and running AI, Azure services, and custom logic directly on IoT devices. It employs a range of development applications and is also compatible with Azure Machine Learning for model deployment. Azure IoT Edge is the perfect solution for low latency required applications that include predictive maintenance, and real-time analytics.
Edge Impulse is a development platform that is specially designed for building embedded machine-learning applications. It provides assistance in data acquisition, model training, and even deployment of models on edge devices. By means of a user-friendly interface and the capability to work with many diverse hardware platforms, Edge Impulse is the best choice for developers that want to create and publish Edge AI applications in an efficient and timely manner.
The Intel OpenVINO (Open Visual Inference & Neural Network Optimization) toolkit accelerates AI workloads across Intel hardware by its design. It makes the focus on deep learning models and enables distribution to a wide range of edge devices. In the shape of an alternative, by OpenVINO, computer vision and natural language processing applications are supported in this way, thus, it can be seen also as a multifunctional tool for potential AI development in such important areas like healthcare, retail, and industrial automation.
IBM Edge Application Manager is a full package solution for the installation and operation of AI-based applications for edge devices. This tool is self-managed and users can deploy new versions or updates as they appear. Edge Cluster Manager platform of IBM provides a wide variety of use-case scenarios such as industrial automations, energy management, and remote productivity tools.
Sony Spresense is a small development board made for the applications of edge computing. It is built out of a multicore microcontroller and supports several AI frameworks, and, therefore, it is very appropriate for the processing of audio, the operation of computer vision, and other AI usage. Presence is very well-matched for devices that work with batteries because it needs very little power.
Balena is a powerful solution that eases the process of launching and handling applications based on containers on edge devices. It comes with a set of instruments for deploying, running, and monitoring the applications that are at the edge, as well as it can be interconnected with numerous types of hardware. Balena is also important for IOT tasks that depend upon scalability, and dependability is very instrumental.
Siemens MindSphere is a solution for industrial IoT as a service that connects products, plants, systems, and machines to give them advanced analytics and AI applications. The Siemens MindSphere application allows for the development of Edge AI applications in the area of industrial processes. It enables data integration, processing, and visualization through providing instruments for those needs.
1. Decreased Latency: Edge artificial intelligence inventions execute details immediately in a limited way, therefore the decision and output time in case of the automatic system speed up.
2. Tightened Privacy: The accomplishment of functionality through devices nearby your local area instead of distant cloud data processing is the point of the lower use of the internet for transmitting private data. This is a key moment for the security of information.
3. Increased Efficiency: Moving tasks to devices at the edge releases the pressure in the cloud, thus, they could be used to the maximum extent possible.
4. Scalability: Edge AI application development abstracts its handling from a flexible platform that empowers the users to deploy and manage their applications in various devices and environments.
5. Robust Ecosystem: These platforms often integrate with existing cloud services and development tools, streamlining the development process and enabling seamless deployment.
The growth of Edge AI has changed our approach to AI apps which involves thinking of where intelligence is closest to the data is created. The 10 best platforms for developing Edge AI discussed and reviewed in this article offer a wide range of features and an awesome stack of technologies, with different features that can be used in various applications and industries.
Through the use of these platforms, developers can come up with new AI solutions that are simple, quick and secure. The course of Edge AI development will show which companies take the mobile app idea and enable it to become useable at a faster pace time and enable the mobile app idea.
Edge AI refers to the deployment of artificial intelligence algorithms on local devices (such as sensors, smartphones, and IoT devices) rather than relying solely on cloud-based computing. This allows for faster data processing, enhanced privacy, and reduced latency.
Edge AI is important because it enables real-time data processing and decision-making, enhances data security by minimizing data transmission to the cloud, and improves the efficiency of AI applications by reducing reliance on cloud infrastructure.
Common use cases for Edge AI applications include autonomous vehicles, predictive maintenance, smart cities, healthcare monitoring, industrial automation, and retail analytics.
Platforms for developing Edge AI applications provide tools and frameworks for data acquisition, model training, deployment, and management. They streamline the development process, support various hardware, and integrate with existing cloud services, making it easier for developers to create and deploy AI applications.
Challenges associated with developing Edge AI applications include ensuring data security and privacy, managing computational resources on edge devices, dealing with hardware limitations, and maintaining scalability and reliability in diverse environments.