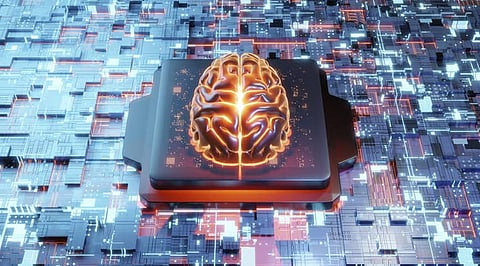
Today, two exceptionally talked about disruptive technologies are artificial intelligence and blockchain. Both AI and blockchain include technical complexity and there is by all accounts a sense of arrangement among specialists that these technologies will have intense business implications in the following five to ten years. The joint utilization of the two innovations may change the tech and business worldview altogether enough for business leaders to take more notice of advancements in this space.
The recent advances in machine learning within the field of AI have not just made another model for general computation, but they have opened the opportunities for the expansion of knowledge past the direct organization of the human mind
A blockchain can not just keep up the datasets on chain for input to AIs, it can likewise have an AI advanced enough to work with its own data and accomplish the alarm call of independently advancing knowledge—the artificial general intelligence (AGI). This is a controversial proposition, and all the more so in a decentralized setting where all users will actually want to access and benefit from its computational capacities.
Distributed storage systems like the blockchain require consensus protocols to choose which node will add newly created blocks to the blockchain and subsequently give the network the latest transactional data. At present, various conventions exist for arriving at consensus in a distributed system, with the most conspicuous ones being the proof-of-work (PoW) and proof-of-stake (PoS) protocols. Nonetheless, these protocols are imperfect in that they devour a ton of energy (PoW) or, will in general, support nodes with enormous coins stakes, possibly hoarding its blockchain (PoS).
Artificial intelligence, and particularly neural networks, represents a solution for these issues by utilizing them in a new, energy-saving protocol – proof of artificial intelligence (PoAI). By utilizing this protocol, the consensus system guarantees a reasonable node determination, keeps the blockchain decentralized, and decreases the issues with energy waste and mining conflicts.
An artificial intelligence agent (AIA) goes above and beyond a trained system by utilizing the new information in the user's request to propel the neural network forward by another age; in other words, it learns as it works. Fitness is determined when negligible or no different changes are required with any future submitted information. For example, in recent years, plenty of authored code in different programming languages has been stored in repositories such as GitHub. Complex algorithmic programming is a tedious and expensive assignment. A programmer requires a high insight and long stretches of education, and complex tasks regularly require numerous months of collaborating work between various parties.
Blockchains can aid in two different ways. Initially, a blockchain can store the code. Furthermore, an AIA, encoded on the blockchain, can help the software engineer from various perspectives: Conversion of code from one language to another, looking for algorithms that match trends, conformance of prerequisites or documentation to code and ultimately in writing new algorithms. Utilizing deep learning in techniques and big data mining from existing code repositories, this AIA would introduce a solid, secure and disruptive innovation.
Just as storing code and programs on the blockchains, we could likewise post trained neural networks. At that point, users could post new transactions that referred to and utilized the trained CNN frameworks to check the submitted data. For instance, consider a deep learning algorithm like Sci-kitin Python, that segments documents, in an altogether different way to deal with what we may expect: Words can be addressed as embedding vectors with the possibility that two words that alike have similar vectors
Under this model, ideas that are alike are close together (e.g., man and woman). Therefore, assuming that the embedding vectors for canines and pups are near one another, the similitude of two documents discussing canines and pups will be perceived by an ML algorithm or a deep neural network trained on that subject. Such tools, well-built, could help programmers in their utilization of the previously mentioned code repository.
The presentation of artificial intelligence, RNNs and particularly LSTMs has empowered complex time-series forecasting, which is the area of machine learning zeroed in on foreseeing parameters in the future by referring parameters from the past.
Utilizing information on bitcoin's (or any cryptocurrency, so far as that is concerned) past value points, RNNs can be trained to gauge its future cost. This empowers major parts of the retail business to represent future cost increases/decreases, conceivably encouraging the change to the execution of digital currencies.
Join our WhatsApp Channel to get the latest news, exclusives and videos on WhatsApp
_____________
Disclaimer: Analytics Insight does not provide financial advice or guidance. Also note that the cryptocurrencies mentioned/listed on the website could potentially be scams, i.e. designed to induce you to invest financial resources that may be lost forever and not be recoverable once investments are made. You are responsible for conducting your own research (DYOR) before making any investments. Read more here.