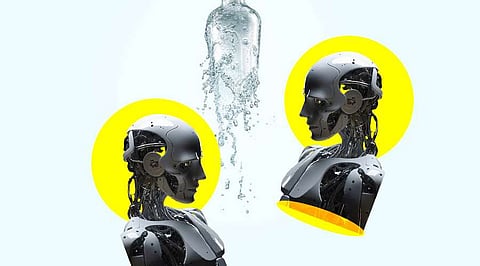
Artificial Intelligence (AI) has undoubtedly revolutionized numerous industries, providing advanced solutions and transforming our lives and work. AI algorithms have become indispensable in our increasingly digital world, from voice assistants to autonomous vehicles. However, as the capabilities and applications of AI expand, so does its environmental impact. One critical concern that has emerged is the water footprint of AI training, highlighting the need for sustainability practices in this rapidly evolving field.
AI training involves feeding massive amounts of data into algorithms to enable machine learning and decision-making. These algorithms require significant computational power, which demands substantial energy consumption and cooling systems. What often goes unnoticed is the water-intensive nature of cooling data centers and the manufacturing of computer chips required for AI infrastructure.
According to a report published by ChatGPT AI, the popular language model developed by OpenAI, the water consumption associated with training a single AI model can be staggering. Estimates suggest that training a large AI model could consume over 626,000 gallons (2,369,000 liters) of water, equivalent to the water usage of an average person for more than two decades. These figures reveal the hidden water footprint of AI, which can have profound environmental consequences if left unaddressed.
The intensive water usage of AI training is mainly attributed to the cooling requirements of data centers. Cooling systems are essential to prevent overheating of servers and ensure optimal performance. However, the process often involves using water for cooling towers or in direct contact with server racks. As AI infrastructure expands to meet the growing demand, the water consumption associated with data centers is expected to increase significantly, amplifying the environmental impact.
To put this issue into perspective, let's consider the example of India. As reported by India Times, the country rapidly embraces AI technology across various sectors. However, with its already water-stressed regions, the water footprint of AI training poses a significant challenge. The report highlights that a single data center in India can consume up to 2.8 million gallons (10,599,200 liters) of water daily. With multiple data centers operating in the country, cumulative water consumption becomes a cause for concern, especially in water scarcity areas.
Efforts are being made to address the environmental impact of AI training. Tech giants and AI developers are increasingly optimizing algorithms and infrastructure to minimize energy and water consumption. For instance, Google has made significant progress in reducing water usage in its data centers by employing advanced cooling technologies and water recycling systems. Similar initiatives are being undertaken by other industry leaders to improve the sustainability of AI operations.
Furthermore, researchers are exploring innovative techniques to make AI training more water-efficient. One such approach involves "transfer learning," where pre-trained models are adapted to new tasks with minimal additional training. By reducing the data required for training, this technique can potentially decrease the water footprint associated with AI model development.
In addition to industry initiatives, policy interventions are necessary to ensure responsible and sustainable AI development. Governments and regulatory bodies can play a vital role in promoting water-efficient practices and setting standards for AI infrastructure. Collaborative efforts between technology companies, policymakers, and environmental organizations are essential to drive positive change and mitigate the environmental impact of AI training.
Moreover, the responsibility does not solely lie with AI developers and policymakers. As users of AI technologies, we can contribute to water conservation by adopting conscious practices. For instance, optimizing algorithms to reduce computational requirements, utilizing energy-efficient hardware, and supporting companies that prioritize sustainability can all make a difference in reducing the water footprint of AI.
The remarkable advancements in AI technology have brought forth numerous benefits and possibilities. However, it is crucial to acknowledge and address the environmental challenges associated with AI training. The water footprint of AI is a pressing concern that demands immediate attention from all stakeholders involved. By leveraging innovative solutions, promoting sustainable practices, and fostering collaboration, we can quench the AI thirst while safeguarding our precious water resources for future generations.
Join our WhatsApp Channel to get the latest news, exclusives and videos on WhatsApp
_____________
Disclaimer: Analytics Insight does not provide financial advice or guidance. Also note that the cryptocurrencies mentioned/listed on the website could potentially be scams, i.e. designed to induce you to invest financial resources that may be lost forever and not be recoverable once investments are made. You are responsible for conducting your own research (DYOR) before making any investments. Read more here.