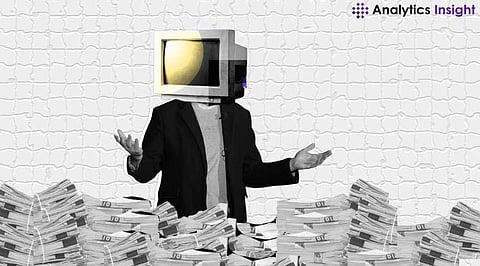
The pace of inventions and discoveries, especially as we head towards the year 2024 in many fields, has been inevitable. These are discoveries in artificial intelligence, new health methods, and fast-stacking research across the scientific landscape that will leave people speechless. In doing so, AI (Artificial Intelligence) is setting the frontiers of knowledge and promising to rewrite the future. This article delves into some of the noteworthy research papers of 2024, which has given exemplary material so that any academic, professional, or enthusiast can stay atop in their fields after reading it.
This paper explains a few of the first peeks into the mind of GPT-4. GPT-4 can solve zero-shot and challenging tasks spanning from mathematics to coding, vision, medicine, law, and psychology, among many others, not requiring any special prompting. More than that, in all these tasks, the performance of GPT-4 stays very close to human-like, with most of the tasks way higher than prior models like ChatGPT.
This study aims to answer the question, 'What is the smallest profile that supports large emergent abilities?' In line with its natural predecessor GPT-4, the largest models show their robust performance and compete with top-tier experts in every textual domain. The short answer is that quite small quantities of high-quality data seem to suffice to achieve very high levels of reasoning ability. Building on this work, and on each other, models Phi1, Phi1.5, and Phi2, each at a scale of 2.7 billion, branch from a parent study.
This paper explores the Meta of releasing the largest segmentation dataset to date with more than 1 billion masks on 11M licensed and privacy-respecting images. The model is designed and trained to follow prompts so that it can transfer zero-shot to new image distributions and tasks. The reason that it is a big deal is mainly due to its ability to identify all "objects" in an image out-of-the-box and generate masks accordingly! No fine-tuning is needed.
Once you have the mask of an object, then you will be able to easily manipulate the image at will manually or via API focusing on that particular object. Examples include fashion virtual try-on, object counting, prompt-based precise editing, etc. The possibilities become endless.
This paper explains DPO (Direct Preference Optimization), which provides a much easier way to fine-tune unsupervised language models for alignment with human preferences. Unlike complex traditional methods, simple classification loss that doesn't require heavy sampling or tuning of hyperparameters makes DPO much lighter, more stable, and amazingly good at tasks like sentiment control and summarization. DPO reflects huge progress in fine-tuning LMs. The great thing about this approach is that it saves a lot of time and is pretty resource-friendly. It is somehow very much akin to a substitute for traditional methods like reinforcement learning with human feedback.
This paper prioritizes ChatGPT moment for Robotics. The work investigates how far vision-language models, trained on vast Internet data, go in generalization in robotic control and emergent semantic reasoning. It simply allows for general-purpose robotics that outperforms all other models that are specialized.
This paper explains how LLM innovations discover new algorithmic solutions, including explaining a technique that combines a pre-trained large language model with a systematic evaluator that has accomplished brand new problem-solving capabilities, which in turn give ground-breaking discoveries in extremal combinatorics and algorithmic problems by searching for source code of problem-solving programs rather than explicit solutions.
This paper ranks among the most influential AI papers on drug discovery. DeepMind, this new paper presents how large-scale deep learning opens up new perspectives into the advanced discovery of new materials and discovers more than 2.2 million new stable structures that increase the known database of stable materials ten times.
For example, lean energy technology with better solar cells and batteries; discovering material with unique quantum properties; nanotechnology; electronics with better sensors, display, and lighting technologies; aerospace and automotive industries with improved strength-to-weight ratios that alone could mean lighter, more fuel-efficient vehicles and aircraft.
This paper explores the problem of fine-tuning these large language models (LLMs) in LLaMA models with the Alpaca approach but on a single machine. QLoRA is an effective method for fine-tuning the model. It allows the training very large language models, such as a 65B model, on a single GPU. Equipping it with methods such as 4-bit quantization and Low-Rank Adapters to drastically save on memory is something.
Its backbone model, Guanaco, almost reaches the same performance as ChatGPT on the Vicuna benchmark, demanding drastically less resources. This makes it possible for finetuning with a very large pool of models while showing that GPT-4 evaluations are a practical method for the assessment of chatbots. Findings, models, and code, courtesy of QLoRA, are publicly released for the good of language model development.
At the cutting edge of novelty, just before 2024 arrives, with so much rapid development in artificial intelligence and frontier fields have never ceased to amaze and stimulate the mind's imagination. The research papers mentioned below have represented insights in the ability to help redefine to others what was edging toward impossible. These range from things that GPT-4 can already do, to pioneering applications in the domains of AI in material discovery and robotics.
They aren't just abstract papers, the inferences and contributions drawn in AI industries have their impact on policy-making and are changing the very way people have been interacting. Whether it be more effective drug design, democratizing AI through primo-tuning, or just thinking of the ethics of deploying AI, the work being done miles and years from today will surely echo through the annals of history.
Anyone who has the compulsion and the instinct to stay informed and up to date about these rapidly growing fields should consider reading these research papers. They provide an in-depth review of what Artificial Intelligence represents today, how it is in practice, and what to expect in the future.
These are only steps closer to more studies exploring the possibilities of artificial intelligence, which, simply through being written, convert into predominant texts for the researcher, practitioner, or enthusiast, establishing a solid foundation from which to stride forward into the future, in which technology and the creativity of humans work hand in hand to provide solutions to the world's ever-growing list of problems.
1. What makes the research papers of 2024 particularly noteworthy?
A: The research papers of 2024 are significant because they showcase groundbreaking advancements across various fields, particularly in artificial intelligence, that are expected to have a profound impact on both industry and academia. These papers introduce novel methodologies, innovative applications, and new technologies that are likely to shape the future.
2. Why is the paper "Sparks of Artificial General Intelligence: Early Experiments with GPT-4" important?
A: This paper is crucial because it offers insights into GPT-4’s capabilities, which are significantly closer to human-like performance across a wide range of tasks. It marks a major step forward in the development of artificial general intelligence (AGI), demonstrating the potential of AI systems to perform complex tasks with minimal human intervention.
3. What are the key findings of the ‘Textbooks are All You Need’ paper?
A: The key finding is that relatively small quantities of high-quality data can suffice to achieve high levels of reasoning ability in AI models. This research highlights the efficiency of large models like Phi1, Phi1.5, and Phi2, suggesting that scaling AI does not always require massive data inputs.
4. How does the "Segment Anything" model impact image processing and AI?
A: The ‘Segment Anything’ model is a major leap in image processing as it can identify and generate masks for all objects in an image without the need for fine-tuning. This capability opens up numerous possibilities for applications such as virtual try-ons, precise editing, and object recognition, making it a versatile tool in various industries.
5. What is Direct Preference Optimization (DPO) and why is it significant?
A: DPO (Direct Preference Optimization) is a novel approach to fine-tuning unsupervised language models for alignment with human preferences. It simplifies the process by using a classification loss method that is resource-efficient and stable, making it an important advancement for tasks like sentiment control and summarization.