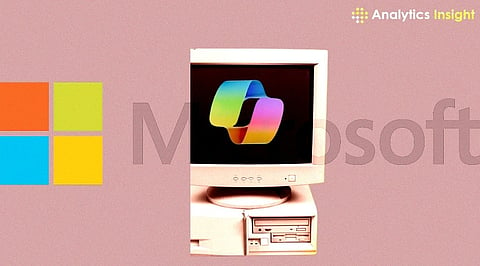
In the ever-evolving landscape of artificial intelligence (AI), the integration of advanced capabilities into products has become a focal point for tech companies worldwide. A recent in-depth study conducted by researchers at Microsoft and GitHub sheds light on the challenges, opportunities, and needs associated with building AI-powered product copilots. Let's delve into the key insights gleaned from this comprehensive exploration.
The overarching goal of embedding advanced AI capabilities into products has sparked a race among technology companies. Virtually every player in the industry is keen on incorporating AI features into their software, yet several challenges persist. One of the primary concerns is the coordination of multiple data sources and prompts, which can elevate the risk of failure cases. Large Language Models (LLMs) pose a unique challenge in testing due to their inherent variability, requiring developers to navigate through trial-and-error processes.
Developers, in this rapidly evolving field, find it challenging to keep up with best practices. Often, they resort to social media or academic papers for guidance. Safety, privacy, and compliance emerge as major concerns that demand careful management to prevent potential damage or breaches. The need for a comprehensive resource or "one-stop shop" for integrating AI into projects remains a significant challenge. Developers express a desire for a platform to kickstart projects quickly, transition seamlessly from a playground to a Minimum Viable Product (MVP), and efficiently integrate AI components into existing codebases.
Prompt engineering, the process of creating prompts that trigger an AI model's inference process, emerges as a significant challenge. The unpredictability of large language models, making them fragile in terms of responses, turns prompt engineering into more of an art than a science. Developers often find themselves engaged in trial-and-error processes, a time-consuming endeavor.
Testing benchmarks for generative models like LLMs introduces another layer of complexity. The variability in responses from these models makes writing assertions difficult, akin to treating every test case as a potentially flaky test. Participants in the study highlight the necessity of running tests multiple times to address this challenge. The lack of appropriate tools for experimentation adds to the time-consuming nature of testing benchmarks.
Privacy and safety concerns associated with integrating AI into products are paramount. Participants in the study voice apprehensions about the potential real-world impact of AI applications, especially in critical environments such as nuclear power plants. The absence of specific paths or guidelines for developers in this nascent field adds to the complexity. Many developers express the sentiment of learning as they go, with no predefined roadmap for the right way to integrate AI effectively.
The article also touches upon Microsoft's recent design changes and upgrades to its CoPilot tool. The inclusion of image editing capabilities for English-speaking Copilot users in select regions signifies a step forward. However, it is acknowledged that some Copilot Pro subscribers have reported performance issues.
The study conducted by Microsoft and GitHub researchers provides valuable insights into the challenges faced by developers in the realm of building AI copilots. From the intricacies of prompt engineering to the complexities of testing benchmarks and ensuring safety and privacy, developers are navigating uncharted territories. As the field continues to evolve, addressing these challenges becomes crucial for the successful integration of AI into products, reshaping the future of technology.
Join our WhatsApp Channel to get the latest news, exclusives and videos on WhatsApp
_____________
Disclaimer: Analytics Insight does not provide financial advice or guidance. Also note that the cryptocurrencies mentioned/listed on the website could potentially be scams, i.e. designed to induce you to invest financial resources that may be lost forever and not be recoverable once investments are made. You are responsible for conducting your own research (DYOR) before making any investments. Read more here.