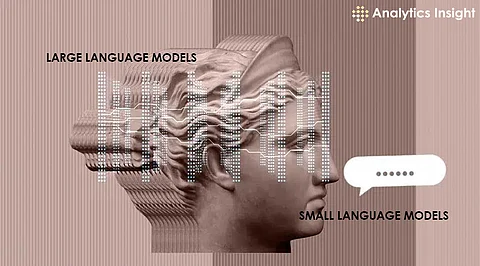
The quick evolution of the field of natural language processing can be seen in the discussions surrounding the language models' types, that is, between the large language models (LLMs) and the small language models (SLMs). As organizations and researchers delve deeper into harnessing the power of NLP for various applications, they are confronted with the question: Which one to consider? Large Language Models or Small Language Models? The attention is not only on the model sizing or performance, the latter extends beyond robustness, and attributability to ethics. Hence we discuss in this article about the Language Models of AI ranging from Large Language and Small Language models and which suits your purpose with their performance.
Large language models are those Language Models of AI boasting extensive multitudinous parameters, which are provisionally counted in the billions or trillions. These values make the nodes a numeric representation of the algorithm to implement the input and produce the output. When the parameter count is extended, a model gains in complexity and accuracy. In most cases, large language models will have been trained on extensive databases of textual information, often coming from the web the whole length and breadth of which the models will have found it possible to assimilate the complicated grammatical and lexical structures of natural language. One such revolutionary feature of these language models is their size. Models like GPT-3, BERT, and T5 are the ones that are best known for their immersive nature.
Small language model highlights are often characterized by a low parameter count, typically between a few millions to a few tens of millions. These parameters are the numbers that underlie the internal language of the model and hold it together in the process of input processing and output generation. Diminishing the expressiveness and complexity of model at lower parameters is the main functionality of Small Language Models. Generally, small language models are trained on restricted text datasets having more focused content pertaining to specific domain or tasks that helps learn contextual associations and language patterns quickly. Case studies of such language with space compact models are ALBERT, DistilBERT, and TinyBERT.
Now that we are aware of both Large Language and Small Language models, let us dive deep into the pros and cons of both Large Language and Small Language models to get a deeper understanding of the best fit.
Large Language Models use large amounts of data to learn more thoroughly, and they become a lot better at generating fluent, coherent yet varied texts. This is because of their unmatchable grasp of linguistic patterns and structures derived from vast amounts of data
The neural nets perform outstandingly well in carrying out the challenging and novel tasks including elaborate statements and accurate classification, which the small neural networks are incapable of.
Large Language Models brilliantly harness transfer learning and few-shot learning mechanisms; their pre-existing knowledge helps them to automatically adapt aptly to all-new tasks and domains with little or no additional coaching.
Large Language Models differ from Small Language models in theri demand of higher costs, and complexities for both training and deployment which in turn can increase the costs for more hardware, software and human resources.
Apart from this, Large Language Models can most likely make more errors and use biased rules which in turn leads to incomplete text, missing the mark or even ending up in a place which could be dangerous, especially in the event of the paucity of data or shallow supervision. Large language models, on the other hand, exhibit much more stability.
In contrast to Small Language Models, large language models for their numerous hidden layers and parameters are transparent and difficult to be understood even by the experts or users, creating the real challenges for comprehending their function and for making decisions regarding their outputs.
The Small Language Models are developed into a relatively inexpensive and straightforward solution in opposition to the expensive and complicated processes of the large models, making the hardware, software, and human demands quite low.
Small Language Models also stand alone with their developed and more enhanced reliability and resilience by creating the text which is more clear, precise and secure especially when there is large amounts of data and supervision which cannot be the case with Large Language Models
Unlike Large Models which use many hidden layers and parameters for various problems, small models keep things simple by distilling to the basics thus becoming more transparent so as to facilitate better understanding. Ultimately, this helps to make them more comprehensible unlike the more complicated large models.
Small Language Models have the drawback of producing text that lacks more fluency, coherency and diversity when compared to the large language models as they leverage very few linguistic patterns and structures from data chunks.
They show an inferiority compared to large language models concerning versatility of usage, the ability to cope with sequences of lesser variety and a smaller generalization expertise, as a consequence of their small capacity of expression.
Their potential for leveraging transfer learning and few-shot learning is comparatively limited, necessitating a greater reliance on additional data and fine-tuning to facilitate adaptation to novel tasks and domains.
Choosing the operational language model that suits your application needs the best also involves some variables to be taken into account. As the creation of the model is your initial step, you should specifically indicate the tasks you want the model to accomplish. If your primary interest is to analyze sentiment or provide answers to questions or perform text summarization which all are the requirements that necessitate deep understanding of natural language, then a large language model will be the right platform for you. In contrast, for a clear-cut case of different objectives like text classification or language generation, a small language model can be your choice to implement.
Data has a primal influence in determining the accessibility of a language model. Large language designs in turn require huge amounts of data during the training phase to achieve top-end quality. If you are on the limited data side, you rather have a small language model trained with less data to optimally fit with the task.
Computational resources along with infrastructure also are among the major concerns to be tackled. Large Language Models are the most sophisticated and consume large amounts of computing power and process. If the deficit of computational resources is a little bit of a problem for you, a Small Language Model also could be a good alternative.
The precision-efficiency trade-off is one important thing to be thought about when this topic is taken into account. A small language model would allow for speedy and less expensive operations, as these usually have lower technological overhead. On the contrary, they may not attain the same level of accuracy compared to large language models. If accuracy is the all-important, a large language model would be the obvious choice.
As AI is revolutionizing the entire world with its day to day advancements choosing the specific language model can be a challenge to pose. But by considering the factors we mentioned in the article, it can be an easy task to do as all the Language Models of AI have their own merits and demerits that make them fit in the place based on the user’s requirements.
1. What is the difference between NLP and Large Language Models?
NLPs encompass diverse number of models and ways to take human language into account, whereas Large Language Models is a true reflection of particular model within the domain
2. Which language model does ChatGPT use?
ChatGPT uses GPT3 Large Language Model to process data
3. What is the best Large Language Model?
Falcon is the best Large Language Model to consider so far, surpassing previous models like GPT 3.
4. What is the best Small Language Model to consider?
TinyLlama is one of the best Small Language Models to consider owing to its capacity to perform various tasks
5. Is Bert a Small Language Model?
No, Bert is a Large Language Model
Join our WhatsApp Channel to get the latest news, exclusives and videos on WhatsApp
_____________
Disclaimer: Analytics Insight does not provide financial advice or guidance. Also note that the cryptocurrencies mentioned/listed on the website could potentially be scams, i.e. designed to induce you to invest financial resources that may be lost forever and not be recoverable once investments are made. You are responsible for conducting your own research (DYOR) before making any investments. Read more here.