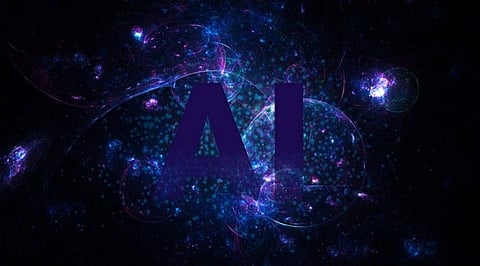
Artificial Intelligence (AI) is today a trending buzzword. By mimicking the human brain like-intelligence, it contributes to digital transformation and acts as a competitive differentiator. Today, AI can comprehend verbal commands, analyze raw data, distinguish pictures, and identify anomalies to prevent fraud and more. Tech giants like Google, IBM, Facebook are working to fuel innovation and encourage the same via the provision of Opensource AI platforms. As the applications of AI become more diverse and sophisticated, it will also enter new industry domains in the coming years. NASA scientists predict that AI will be at the forefront of space travel and innovation in the future.
Artificial intelligence is helping propel the field of space technologies. For instance, last year in March, astronomers at The University of Texas at Austin, teamed with Google, to use AI for uncovering two more hidden planets in the Kepler space telescope archive (Kepler's extended mission, called K2). They used an AI algorithm that sifts through Kepler's data to ferret out signals that were missed by traditional planet-hunting methods. This helped them discover the planets K2-293b orbiting around a star 1,300 light-years away in the constellation Aquarius and planet K2-294b, revolving around a star 1,230 light-years away, also located in Aquarius.
European Space Agency (ESA) plans to leverage AI to reduce the mission costs, extend battery life, and analyze the vast amount of imaging data produced by the satellites. NASA's free-flying robotic system, Astrobee, uses AI to help astronauts reduce their time on routine duties, leaving them to focus more on the things that only humans can do.
Through machine learning algorithms, Artificial intelligence has helped to discover unmarked galaxies, supernovas, stars, black holes, and studying cosmic events that would have otherwise gone unnoticed. E.g., researchers use deep learning to replicate galaxy formation and classify galactic images seen from the Hubble Space Telescope. Deep learning is also applied in automatic landing, intelligent decision-making and fully automated systems.
IBM has played a key role in various space projects and milestones. From early studies of multistage, solid- fuel rockets use an IBM CPC for engineering calculations to CIMON (Crew Interactive Mobile Companion), the first free-flying AI assistant, at the International Space Station (ISS). IBM also helped NASA achieve the first manned landing on the moon and first orbital flight of space shuttle Columbia.
In October, IBM Space Tech Hub Team, led by engineer and space tech CTO, Naeem Altaf, unveiled the two open source projects named KubeSat and Space Situational Awareness (SSA) system. KubeSat is an autonomous cognitive framework designed for swarms of cube satellites that allows for the simulation and optimization of multi-satellite communications. Whereas, SSA is an ongoing collaboration project between IBM and Dr. Moriba Jah of the University of Texas. It aims to improve orbit prediction of human-made anthropogenic space objects (ASOs) with the use of machine learning models, to determine where ASOs are (orbit determination) and where they will be in the future (orbit prediction). It will also help in developing models that learn when physical models incorrectly predict an ASO's future location. Both of these open source AI tools were built by the IBM's Space Tech Hub team and are available as containerized deployments on the company's Red Hat OpenShift platform.
"By open sourcing these two projects, we hope to give more people access to space tech and democratize access to space for all," IBM said in a statement.
KubeSat simulates accurate orbital mechanics for each object via Orekit and uses these calculations to place restrictions on communications between satellites, groundstation, and ground sensors. It incorporates NATS messaging services; optimizing their communications via reinforcement learning and publishes these communications to a UI dashboard. NATS is a high-performance messaging system that acts as a distributed messaging queue. KubeSat also uses Kubernetes as the core platform with microservices.
KubeSat's architecture enables users to swap in artificial intelligence models for specific use cases. Communications between satellite swarms can be autonomous, and depending on the sensors onboard and capabilities, swarms could be called upon for a host of applications and users ranging from meteorological analysis to deforestation.
Meanwhile, IBM's Space Situational Awareness (SSA) leverages two models to monitor space debris, i.e., a physics-based model and a machine learning-based model.
The physics-based model is for predicting orbits of ASOs in LEO (low Earth orbits). It uses Cowell's formulation to model perturbation in an ASO's orbit that is caused by the Earth. In contrast, the machine learning model uses, XGBoost gradient-boosted regression trees. The model is then trained on the orbit predictions of the baseline physical model to predict the error of the physics model using the USSTRATCOM (United States Strategic Command) data acting as 'the ground truth.' Space Situational Awareness combines the two models and adjusts the physical model's prediction according to the predicted error.
The team used IBM Cloud Bare Metal Server with 16 Intel Xeon Processors; 120 GB RAM; two NVIDIA Tesla V100 GPUs (each with 16GB of RAM) to run the physical model and train the machine learning model. They also used Cloud Object Storage to store the data from USSTRATCOM, the physics model predictions, and the final combined model predictions.
SSA can help scientists anticipate threats from ASOs. IBM reports that nearly tens of thousands of ASOs orbit around the Earth. With the privatization of rocket launches, the rate at which ASOs are put into orbit is set to increase dramatically. However, the major concern about these ASOs is that it is tricky to predict location due to infrequent and noisy data, and external factors like space weather and atmospheric density affect their trajectories in unpredictable ways.
Join our WhatsApp Channel to get the latest news, exclusives and videos on WhatsApp
_____________
Disclaimer: Analytics Insight does not provide financial advice or guidance. Also note that the cryptocurrencies mentioned/listed on the website could potentially be scams, i.e. designed to induce you to invest financial resources that may be lost forever and not be recoverable once investments are made. You are responsible for conducting your own research (DYOR) before making any investments. Read more here.