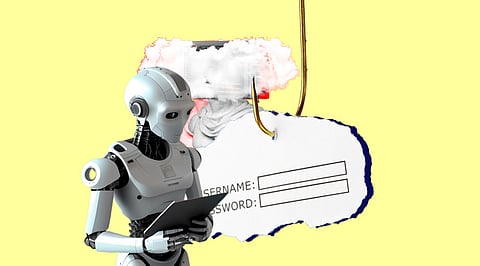
Cyber attackers now have more power than ever thanks to the development of Artificial Intelligence(AI) technology and the general availability of it, which they use to create more convincing phishing messages for their targets. However, there is some good news as well; AI technology has positive applications as well. Amid the escalating threat of phishing attacks, the fusion of AI and machine learning emerges as a potent strategy. This article delves into how these technologies empower robust defenses, thwarting evolving cyber threats.
One of the biggest cybersecurity dangers that affect both personal and business contexts is phishing. According to a Deloitte study, phishing emails are the starting point of 91% of all cyberattacks.
Phishing attacks are carried out when dishonest individuals send a message that appears to come from a trustworthy source (such as your bank, a coworker, or an internet retailer you do business with). In order to install malware on their machine, they try to trick the recipient into clicking on a dangerous link or providing personal information, such as credit card or other account details.
Different platforms are used by cybercriminals to distribute their fraudulent phishing messages. Email is the most popular method. However, additional platforms, like SMS text messages, social media platform messages, and internet messaging, are being effectively employed.
Machine learning algorithms are pivotal in the battle against phishing attacks, bolstered by the capabilities of artificial intelligence (AI). These algorithms, intricately trained on vast datasets of legitimate and malicious emails, possess the proficiency to discern subtle patterns and anomalies that escape human detection. By scrutinizing email headers, content, attachments, and sender behavior, these algorithms can identify telltale signs of phishing attempts, such as suspicious URLs or mismatched domains.
Through continuous learning, these algorithms evolve and adapt to emerging phishing tactics, fortifying their accuracy over time. They can swiftly classify incoming emails into various risk categories, allowing security teams to prioritize responses. Additionally, AI augments this process by automating responses and enabling real-time threat mitigation.
A SlashNext study showed a surge in zero-hour threats in 2022, comprising 54% of detections, with 76% being spear-phishing for credential harvesting. These novel attacks outsmart traditional anti-phishing measures. The research highlights:
1. Evolving attack strategies based on prior failures.
2. Employing automation and ML to launch tailored attacks for higher infection chances.
3. Utilizing three main tactics: link-based, malicious attachments, and natural language threats.
To deploy ML algorithms for phishing detection, extensive training on both normal and suspicious emails is essential. This training allows algorithms to discern anomalies and malicious patterns. Three primary ML methods for identifying phishing emails are employed:
Enterprises construct social graphs depicting regular communication flows among employees. This aids in detecting unusual interactions that could be suspicious. For instance, communications between departments like marketing and public relations are typical, but exchanges between accounting and the company CEO might be rare and warrant scrutiny.
Each individual possesses a distinct email writing style, tone, and phrasing. Specific opening or closing phrases, formatting choices, and sentence structures characterize writing patterns. Leveraging Natural Language Processing (NLP), a subset of AI, enables the extraction of these patterns, facilitating the identification of emails from particular employees.
ML analyzes technical email content to spot suspicious aspects. For instance, IP addresses associated with the email's journey are scrutinized, revealing any unusual or deceptive routes. If an email claims to originate from Microsoft Outlook (Microsoft servers), yet its header indicates Gmail, it could be forged or manipulated.
These ML-driven methods collectively enhance the efficacy of phishing detection systems, providing multifaceted protection against evolving cyber threats.
Disclaimer: Analytics Insight does not provide financial advice or guidance. Also note that the cryptocurrencies mentioned/listed on the website could potentially be scams, i.e. designed to induce you to invest financial resources that may be lost forever and not be recoverable once investments are made. You are responsible for conducting your own research (DYOR) before making any investments. Read more here.