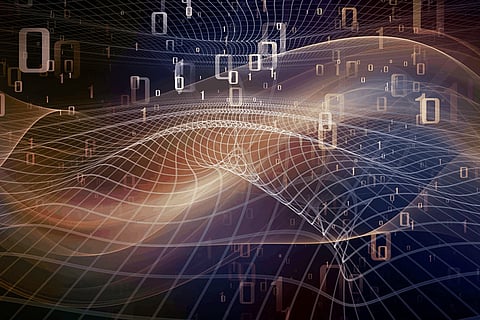
Organizations today are going through a variety of digital hardships. They are trying to find ways to derive value from data through which they want to achieve specific business outcomes. But doing so is not a cakewalk, it takes a lot of effort from data scientists' end to mine data to carve analytics application for driving innovative and efficient decision-making. In order to make analytics more effective, businesses are replacing traditional data management with an emerging set of practices. These practices are focused on collaboration and automation and are known as data operations, or DataOps.
DataOps is a junction of advanced data governance and analytics delivery practices that incorporates the data life cycle. Similar to DevOps, which intend to speed up software development, DataOps embraces agile and continuous-delivery development methods. These methods are supported by on-demand IT resources.
The emergence of DataOps seems promising to organizations to help them optimize their data management; drive initiatives involving data-intensive technologies including AI, ML, and deep learning.
Michael Daw, law library associate dean, and director, and professor of law at Golden Gate University School of Law said – "Finding high-quality, relevant data, promptly, with time to contemplate the meaning of that data will be the challenge. Big data vendors, such as LexisNexis and Westlaw, have designed their natural language searching—their 'smart searching'—to retrieve as much information as possible from as many of their thousands of databases as possible, assuming that more data from more sources leads to better choices."
DataOps helps streamline data management processes in order to maximize the value of huge data stores of companies. It is a process-oriented methodology that tends to improves cross-functional communication, integration, and automation of data flow between different stakeholders, from the network edge to multi-cloud platforms. It connects data creators with data consumers to increase collaboration and digital innovation.
According to Forrester Research, some of the main priorities of DataOps practices are to spin up labs at a quick pace to support insights from enhanced data analysis, to develop pipelines and APIs for operational real-time services, to develop and deploy architecture from data strategies, to federate data governance and to handle data regulatory requirements.
Where on one hand DataOps is a relatively new field, best practices and proven technologies are already emerging in the space. These practices possess wide applicability for data analytics, data governance, and operational agility. But, significantly, DataOps is particularly well-suited to drive AI, ML, and deep learning as these data-hungry technologies require progressive improvements to remain effective.
A number of organizations are already leveraging their data to aid the enhancement of efficiency and drive advanced functions. DataOps can empower AI-driven data-intensive applications – IoT, advanced research efforts and complex financial analysis.
William Mayo, CIO at the Broad Institute of MIT and Harvard asserted, "Algorithm experts are dreaming up new ideas every day to leverage this new scale [of data]. On one hand, change is simply—more of the same. The problem is that 'the same' now means everything will continue to change, just faster."
The emergence of DataOps marks the true evolution of process and philosophy altogether. According to Mayo, 'to a very real extent, the industry has inverted the very basis of the scientific method, which was essentially to form a hypothesis, design an experiment and collect data, and analyze, refine, and repeat. Today, it is more like collect data, ask an algorithm what might be interesting, then react and repeat.'
Join our WhatsApp Channel to get the latest news, exclusives and videos on WhatsApp
_____________
Disclaimer: Analytics Insight does not provide financial advice or guidance. Also note that the cryptocurrencies mentioned/listed on the website could potentially be scams, i.e. designed to induce you to invest financial resources that may be lost forever and not be recoverable once investments are made. You are responsible for conducting your own research (DYOR) before making any investments. Read more here.