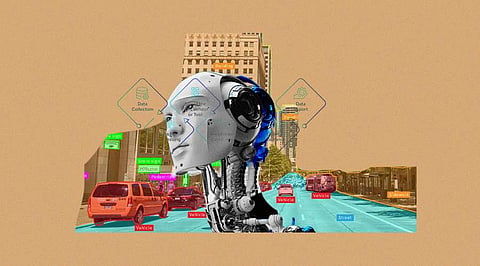
In machine learning (ML) and artificial intelligence (AI), data is the lifeblood that fuels the development of sophisticated algorithms and models. However, raw data must be appropriately labeled and organized to leverage the full potential of data-driven technologies. This process is known as data annotation, a critical step that enhances the accuracy and performance of ML and AI systems.
Data annotation is the process of labeling and categorizing data to provide context and meaning for ML and AI algorithms. Human annotators, often domain experts, manually label data points with specific attributes, classes, or categories, enabling the algorithm to recognize patterns, make predictions, and learn from the data effectively.
Image Annotation: In image-based tasks, annotators label objects, regions, or key points within an image. Common techniques include bounding boxes, semantic segmentation, and landmark annotation.
Text Annotation: Text data requires various annotation methods, such as named entity recognition (NER), sentiment analysis, part-of-speech tagging, and semantic role labeling.
Audio Annotation: For audio data, annotators may transcribe speech, label sound events, or mark specific timestamps for various events.
Enhanced Model Training: Data annotation provides labeled data, a ground truth for training ML and AI models. Well-annotated data enables models to understand patterns and relationships, improving accuracy and generalization.
Algorithm Performance: Annotated data enhances the performance of algorithms by reducing noise and ambiguity. Accurate annotations help algorithms make informed decisions and predictions, reducing the risk of biased outputs.
Domain-Specific Customization: Data annotation allows ML and AI models to be tailored to specific domains or industries. This customization ensures that the algorithms align with the unique challenges and requirements of the target applications.
Rapid Development Cycles: Annotated data expedites the development process by providing a ready-to-use dataset for model training. This saves valuable time and resources, allowing faster prototyping and iterations.
Increased Model Trustworthiness: Annotating data enables the monitoring and auditing of ML and AI models, making them more transparent and accountable. This fosters trust among users and stakeholders, crucial in sensitive applications like healthcare and finance.
Data annotation comes with challenges, such as needing skilled annotators, ensuring data quality, and addressing biases. To overcome these challenges, organizations can adopt best practices like:
Ensuring clear annotation guidelines and regular training for annotators.
Implementing quality control measures and validation checks for accuracy.
Addressing bias by diversifying the annotation workforce and continuous monitoring.
Join our WhatsApp Channel to get the latest news, exclusives and videos on WhatsApp
_____________
Disclaimer: Analytics Insight does not provide financial advice or guidance. Also note that the cryptocurrencies mentioned/listed on the website could potentially be scams, i.e. designed to induce you to invest financial resources that may be lost forever and not be recoverable once investments are made. You are responsible for conducting your own research (DYOR) before making any investments. Read more here.