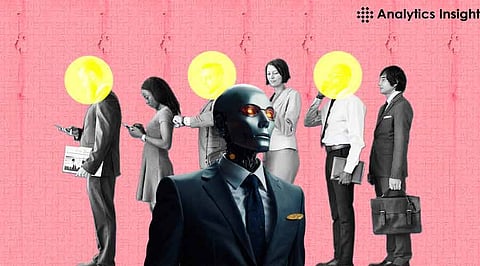
The introduction of artificial intelligence in recruitment has made the process faster and more objective. However, AI raises high risks in terms of bias and ethics. It is supposed to reduce human bias, but AI often promotes or could even worsen the situation.
Let’s take a look at controversies surrounding AI-based hiring tools closely, and understand their potential biases, and ethical concerns.
Bias is one of the biggest concerns associated with AI-based hiring. There are multiple ways through which an AI algorithm can propagate bias.
1. Bias via trained data sets: If data used for training mainly comprises one demographic, then the system would automate its favoritism towards such individuals. For instance, if AI is trained on data from only young workers, then, it may reject the resumes of older but experienced candidates.
2. Riding on non-relevant criteria: AI may give importance to the factors irrelevant to workplace performance, such as gender, race, or age, increasing discrimination. For example, if most past hires have traditionally Western-sounding names, then the system would prefer those and reject others.
Several high-profile cases revealed some seemingly hidden prejudices inherent in AI recruitment tools:
1. Amazon's Recruiting Tool: Amazon was compelled to scrap its AI hiring tool which was developed in 2015, after it showed bias against female candidates. It reportedly lowered the ranking of female resumes when it picked up phrases like "women's club." The bias of the tool arose from the fact that its training data set was taken from 10 years ago, consisting primarily of males.
2. HireVue's Bias Allegations: HireVue's video interview platform has been criticized for showing racial and gender biases. The firm relied on artificial intelligence to review candidates' facial expressions and voice. This led to discrimination against people with darker skin tones and people unfamiliar with the English language.
Beyond the question of bias, are other ethical concerns surrounding AI-based hiring tools, like transparency and accountability.
1. Opacity in decision-making: Many AI systems are black boxes, technically known as Blackbox AI. Meaning, employers, as well as candidates, do not fully understand how decisions are being made. That lack of transparency can erode trust. It also makes it difficult to challenge biased outcomes.
2. Lack of Accountability: Who is accountable for AI making discriminatory decisions? Is it the developer or the company that adopts the system? This type of confusion regarding the assignment of responsibility leaves no scope for affected parties to pursue remedies by direct complaints.
3. Increases Disparity: Algorithms used in hiring can affect the most vulnerable populations with more intensity. As these vulnerable groups already suffer from structural disadvantages regarding employment, biased AI tools would only further entrench such inequalities.
Though some risks cannot be overcome, AI doesn't have to perpetuate bias. Here's how to make sure that AI-based hiring tools are deployed ethically and fairly:
1. Diverse Data Sets: Companies need to ensure that the training data for AI is diverse and representative of all demographics. This can be achieved by seeking out data from underrepresented groups in a more active way.
2. Regular Algorithm Audits: The biases present in AI algorithms can be well identified and corrected if regular audits of these systems are conducted. Such auditors should be independent, unbiased individuals who hold accountability.
3. Accountability: AI-based hiring systems should provide transparency about the processes used in reaching an outcome. The AI tools have to give explanations about the decisions they make. This means the candidates would get answers on the reasons for their selection as well as rejection.
4. Human Over-Sight: AI definitely should not replace the judgment of humans completely. The best-case scenario would be if it serves as a supplement to human decision-making. The company can reduce the impact of bias and skewed results by giving authority to humans for the final call.
5. Inclusive Design: The AI model training process should include various demographics for age, skin color, gender, etc. Inclusivity in design will help avoid penalizing people for wrongful reasons.
Though hiring through AI-based tools is very efficient and objective, it brings in its risks of biases and ethical dilemmas. Companies will have to take on the onus of approaching these issues with a healthy dose of transparency, fairness, and inclusiveness.
However, without such proactive measures against the risks, AI could increase workplace inequalities rather than eradicate them. Businesses and developers must collaborate to design ethical AI systems whereby fairness takes precedence over efficiency.